How Can Graph Neural Networks Help Document Retrieval: A Case Study on CORD19 with Concept Map Generation
ADVANCES IN INFORMATION RETRIEVAL, PT II(2022)
摘要
Graph neural networks (GNNs), as a group of powerful tools for representation learning on irregular data, have manifested superiority in various downstream tasks. With unstructured texts represented as concept maps, GNNs can be exploited for tasks like document retrieval. Intrigued by how can GNNs help document retrieval, we conduct an empirical study on a large-scale multi-discipline dataset CORD19. Results show that instead of the complex structure-oriented GNNs such as GINs and GATs, our proposed semantics-oriented graph functions achieve better and more stable performance based on the BM25 retrieved candidates. Our insights in this case study can serve as a guideline for future work to develop effective GNNs with appropriate semantics-oriented inductive biases for textual reasoning tasks like document retrieval and classification. All code for this case study is available at https://github.com/HennyJie/CNN-DocRetrieval.
更多查看译文
关键词
Document retrieval, Graph neural networks, Concept maps, Graph representation learning, Textual reasoning
AI 理解论文
溯源树
样例
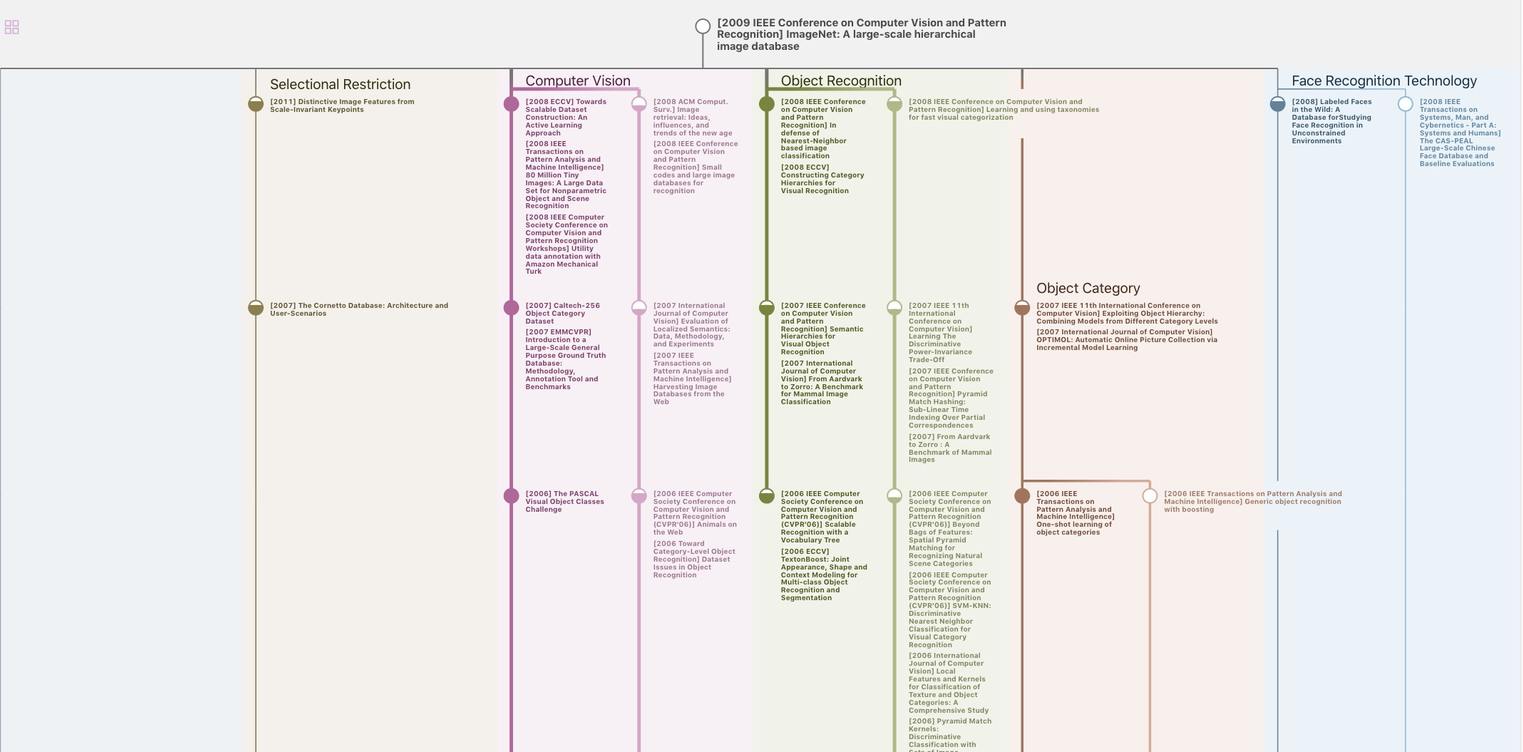
生成溯源树,研究论文发展脉络
Chat Paper
正在生成论文摘要