Hardware Implementation of Low-complexity Deep Learning-based Model Predictive Controller
2021 EUROPEAN CONTROL CONFERENCE (ECC)(2021)
摘要
Model predictive control (MPC) is an advanced control strategy that predicts the future behavior of the plant while considering the system dynamics and constraints. This optimization-based control algorithm needs huge amount of computational resources as it solves the optimization problem at each sampling time. This computational load demands powerful hardware and light-weight algorithms for implementing MPC on an embedded systems with limited computational resources. Deep neural network (DNN) is a attractive alternative for MPC as it provide the close approximation for various linear/nonlinear functions. In this paper, a feed forward neural network (FFNN) and recurrent neural network (RNN)-based linear MPC is developed. These neural network algorithms which are trained offline can efficiently approximate MPC control law which can be easily executed on low-level embedded hardware. The closed-loop performance is verified on a hardware-in-the-loop (HIL) co-simulation on ARM microcontroller. The performance of proposed DNN-MPC is demonstrated with a case study of a 2 degree-of-freedom (DOF) helicopter. Hardware result shows that the DNN-MPC is faster and consumes less memory as compared to MPC while retaining most of the performance indices.
更多查看译文
关键词
Model predictive control, deep neural network, approximate control law, linear systems, HIL cosimulation
AI 理解论文
溯源树
样例
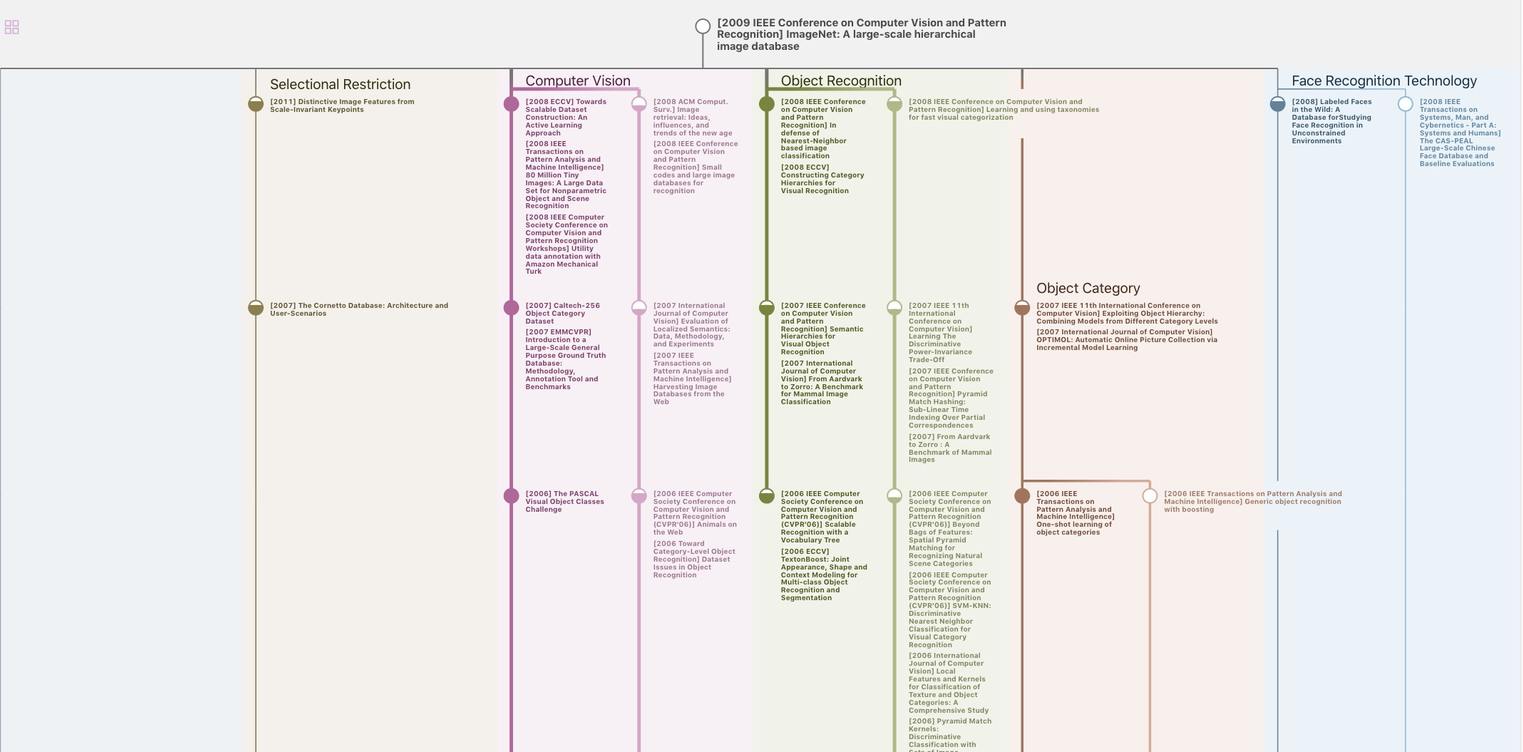
生成溯源树,研究论文发展脉络
Chat Paper
正在生成论文摘要