Deep learning applied to breast imaging classification and segmentation with human expert intervention
Journal of Ultrasound(2022)
摘要
Purpose Automatic classification and segmentation of tumors in breast ultrasound images enables better diagnosis and planning treatment strategies for breast cancer patients. Methods We collected 953 breast ultrasound images from two open-source datasets and classified them with help of an expert radiologist according to BI-RADS criteria. The data was split into normal, benign and malignant classes. We then used machine learning to develop classification and segmentation algorithms. Results We found 3.92% of the images across the open-source datasets had erroneous classifications. Post-radiologist intervention, three algorithms were developed based on the classification categories. Classification algorithms distinguished images with healthy breast tissue from those with abnormal tissue with 96% accuracy, and distinguished benign from malignant images with 85% accuracy. Both algorithms generated robust F1 and AUROC metrics. Finally, the masses within images were segmented with an 80.31% DICE score. Conclusions Our work illustrates the potential of deep learning algorithms to improve the accuracy of breast ultrasound assessments and to facilitate automated assessments.
更多查看译文
关键词
Dynamic U-net,Artificial intelligence,BIRADS,Segmentation
AI 理解论文
溯源树
样例
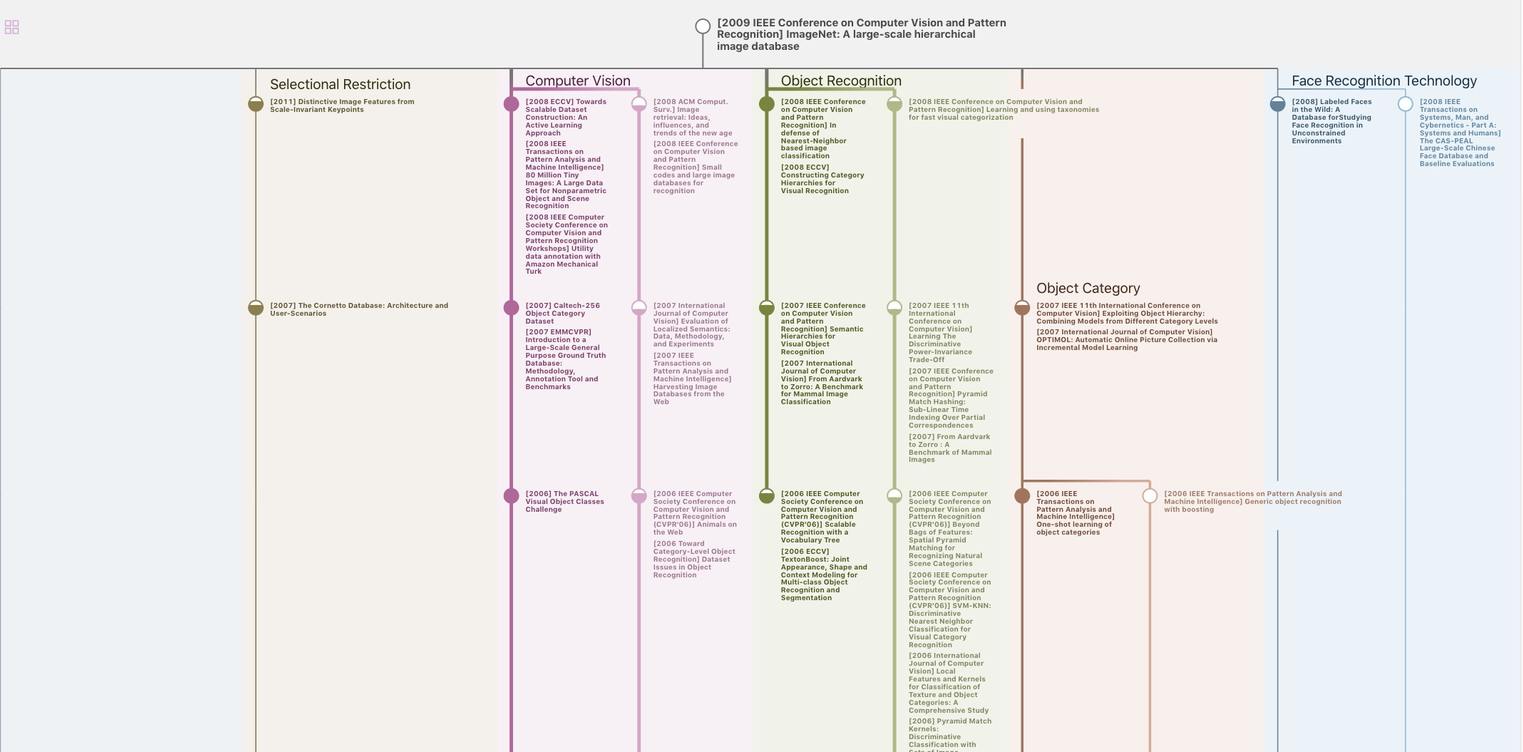
生成溯源树,研究论文发展脉络
Chat Paper
正在生成论文摘要