Local and Global Convergence of General Burer-Monteiro Tensor Optimizations.
AAAI Conference on Artificial Intelligence(2022)
摘要
Tensor optimization is crucial to massive machine learning and signal processing tasks. In this paper, we consider tensor optimization with a convex and well-conditioned objective function and reformulate it into a nonconvex optimization using the Burer-Monteiro type parameterization. We analyze the local convergence of applying vanilla gradient descent to the factored formulation and establish a local regularity condition under mild assumptions. We also provide a linear convergence analysis of the gradient descent algorithm started in a neighborhood of the true tensor factors. Complementary to the local analysis, this work also characterizes the global geometry of the best rank-one tensor approximation problem and demonstrates that for orthogonally decomposable tensors the problem has no spurious local minima and all saddle points are strict except for the one at zero which is a third-order saddle point.
更多查看译文
关键词
Search And Optimization (SO),Constraint Satisfaction And Optimization (CSO)
AI 理解论文
溯源树
样例
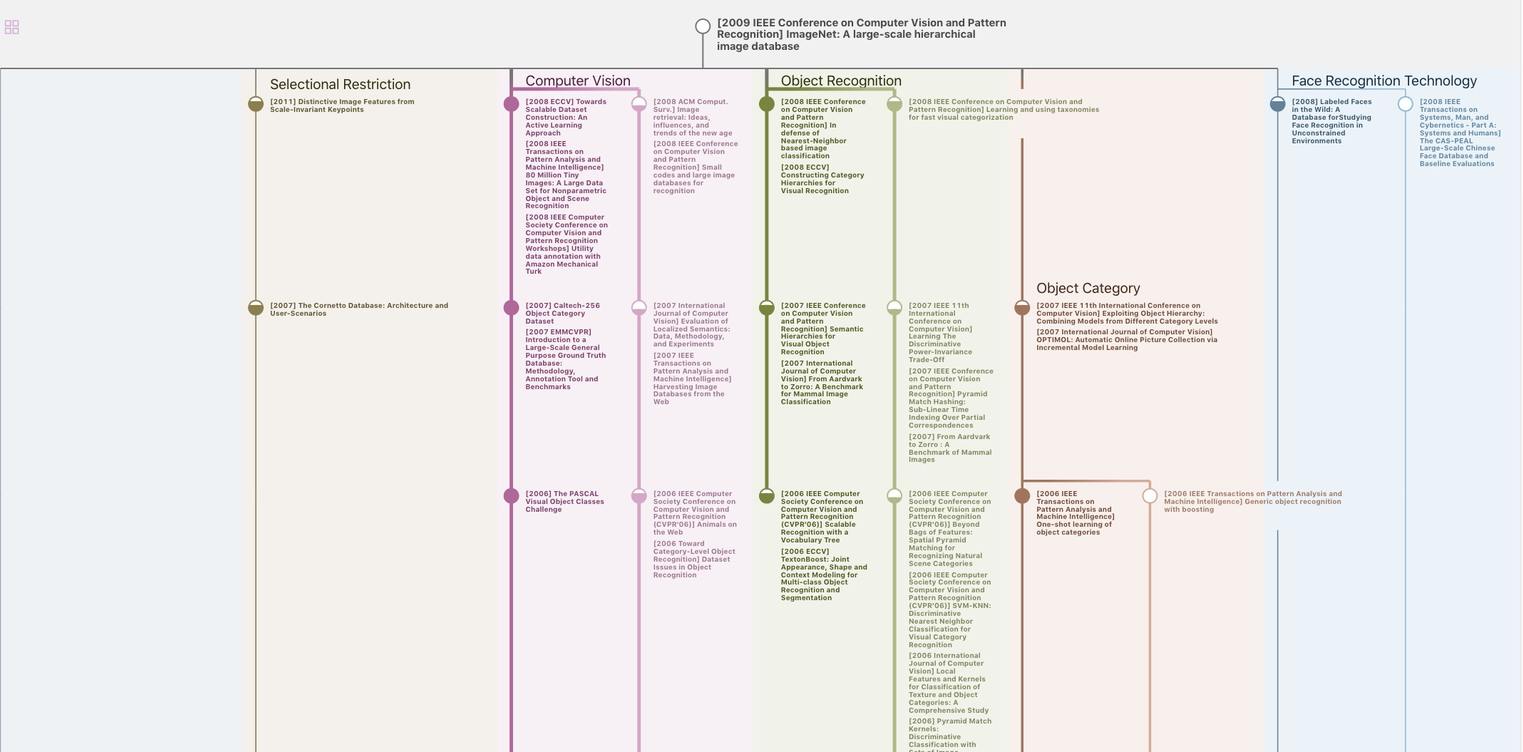
生成溯源树,研究论文发展脉络
Chat Paper
正在生成论文摘要