Biphasic Face Photo-Sketch Synthesis via Semantic-Driven Generative Adversarial Network With Graph Representation Learning
IEEE TRANSACTIONS ON NEURAL NETWORKS AND LEARNING SYSTEMS(2023)
摘要
Biphasic face photo-sketch synthesis has significant practical value in wide-ranging fields such as digital entertainment and law enforcement. Previous approaches directly generate the photo-sketch in a global view, they always suffer from the low quality of sketches and complex photograph variations, leading to unnatural and low-fidelity results. In this article, we propose a novel semantic-driven generative adversarial network to address the above issues, cooperating with graph representation learning. Considering that human faces have distinct spatial structures, we first inject class-wise semantic layouts into the generator to provide style-based spatial information for synthesized face photographs and sketches. In addition, to enhance the authenticity of details in generated faces, we construct two types of representational graphs via semantic parsing maps upon input faces, dubbed the intraclass semantic graph (IASG) and the interclass structure graph (IRSG). Specifically, the IASG effectively models the intraclass semantic correlations of each facial semantic component, thus producing realistic facial details. To preserve the generated faces being more structure-coordinated, the IRSG models interclass structural relations among every facial component by graph representation learning. To further enhance the perceptual quality of synthesized images, we present a biphasic interactive cycle training strategy by fully taking advantage of the multilevel feature consistency between the photograph and sketch. Extensive experiments demonstrate that our method outperforms the state-of-the-art competitors on the CUHK Face Sketch (CUFS) and CUHK Face Sketch FERET (CUFSF) datasets.
更多查看译文
关键词
Face photo-sketch synthesis,generative adversarial network,graph representation learning,intraclass and interclass,iterative cycle training (ICT)
AI 理解论文
溯源树
样例
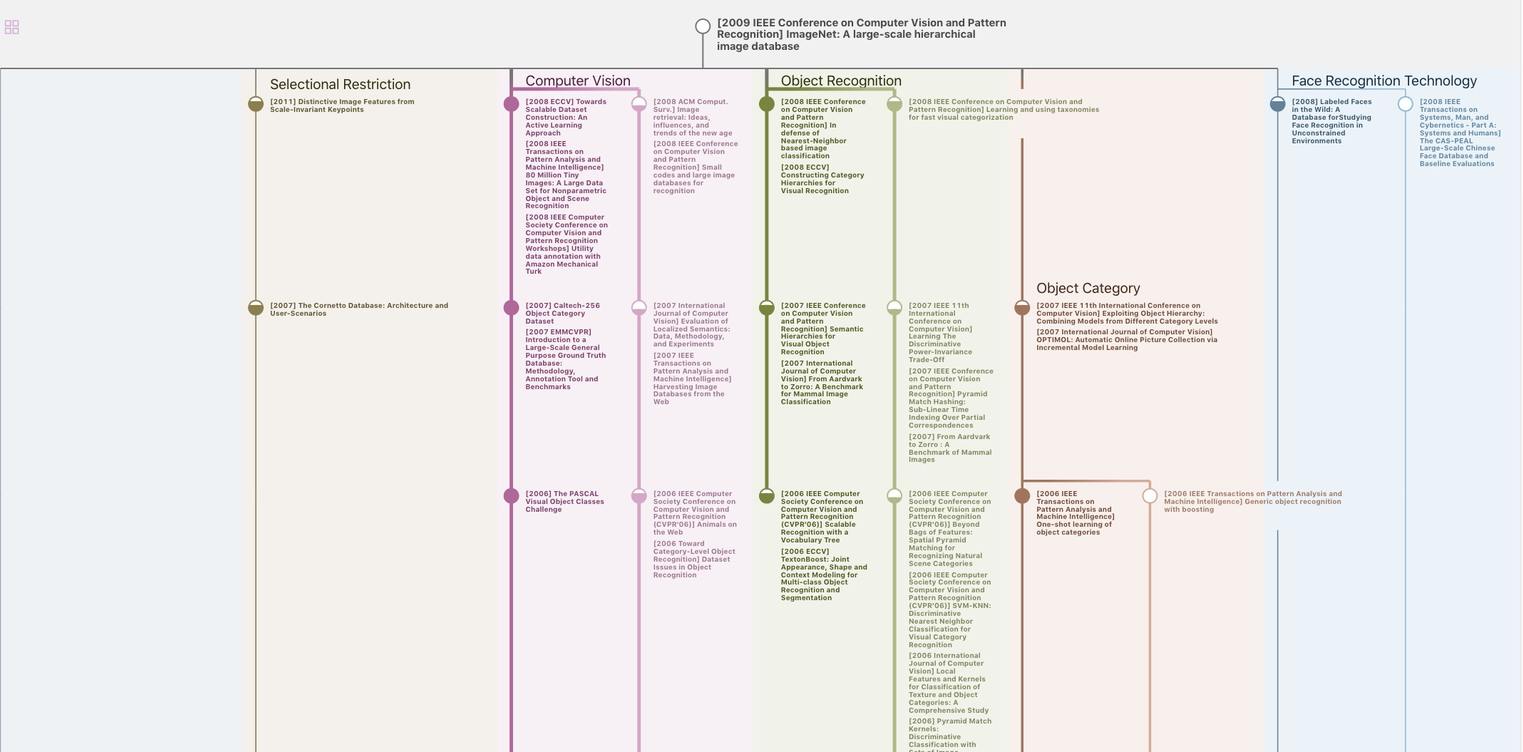
生成溯源树,研究论文发展脉络
Chat Paper
正在生成论文摘要