Auction Throttling and Causal Inference of Online Advertising Effects
Economics and Computation(2022)
摘要
BSTRACTCausally identifying the effect of digital advertising is challenging, because experimentation is expensive, and observational data lacks random variation. This paper identifies a pervasive source of naturally occurring, quasi-experimental variation in user-level ad-exposure in digital advertising campaigns. It shows how this variation can be utilized by ad-publishers to identify the causal effect of advertising campaigns. The variation pertains to auction throttling, a probabilistic method of budget pacing that is widely used to spread an ad-campaign's budget over its deployed duration, so that the campaign's budget is not exceeded or overly concentrated in any one period. The throttling mechanism is implemented by computing a participation probability based on the campaign's budget spending rate and then including the campaign in a random subset of available ad-auctions each period according to this probability. We show that access to logged-participation probabilities enables identifying the local average treatment effect (LATE) in the ad-campaign. We present a new estimator that leverages this identification strategy and outline a bootstrap procedure for quantifying its variability. We apply our method to real-world ad-campaign data from an e-commerce advertising platform, which uses such throttling for budget pacing. We show our estimate is statistically different from estimates derived using other standard observational methods such as OLS and two-stage least squares estimators. Our estimated conversion lift is 110%, a more plausible number than 600%, the conversion lifts estimated using naive observational methods. The full version of the paper : https://arxiv.org/abs/2112.15155
更多查看译文
关键词
online advertising effects,auction,causal inference
AI 理解论文
溯源树
样例
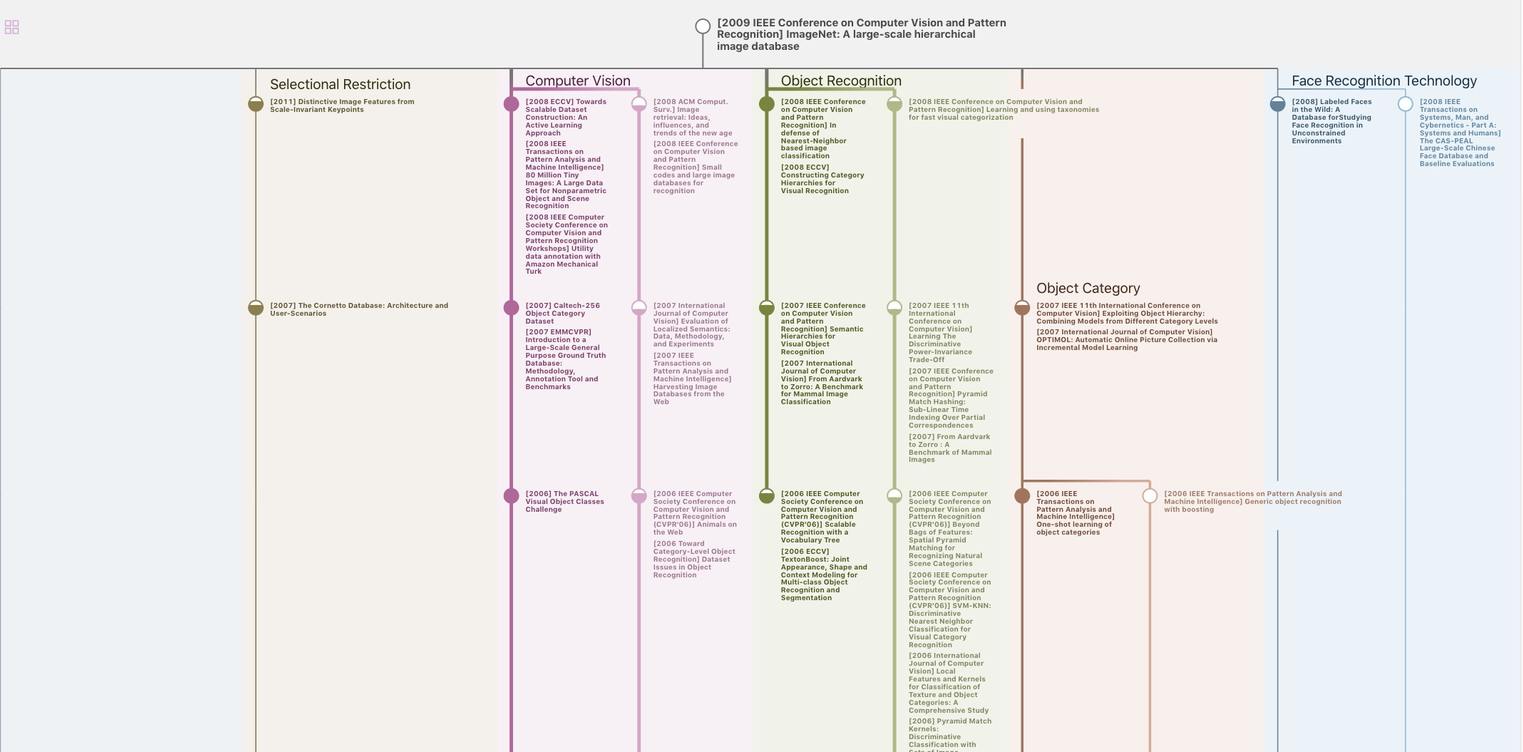
生成溯源树,研究论文发展脉络
Chat Paper
正在生成论文摘要