Spectrogram decomposition of ultrasonic guided waves for cortical thickness assessment using basis learning
Ultrasonics(2022)
摘要
Due to its multimode and dispersive nature, ultrasonic guided waves (UGWs) usually consist of overlapped wave packets, which challenge accurate bone characterization. To overcome this obstacle, a classic idea is to separate individual modes and to extract the corresponding dispersion curves. Reported single-channel mode separation algorithms mainly focused on offering a time–frequency representation (TFR) where the energy distributions of individual modes were apart from each other. However, such approaches are still limited to identifying the modes without significant overlapping in time–frequency domain. In this study, a spectrogram decomposition technique was developed based on a combination strategy of generalized separable nonnegative matrix factorization (GS-NMF) and adaptive basis learning, towards the automatic mode extraction under severe overlapping and low signal-to-noise ratio (SNR). The extracted modes were further used for cortical thickness estimation. The method was verified using broadband simulated and experimental datasets. Experiments were conducted on a bone-mimicking plate and bovine cortical bone plates. For simulated data, the relative errors between extracted and theoretical dispersion curves are 1.33% (SNR = ∞), 1.43% (SNR = 10 dB) and 0.88% (SNR = 5 dB). The root-mean-square errors of the estimated thickness for 3.10 mm-thick bone-mimicking plate, 3.83 mm- and 4.00 mm-thick bovine cortical bone plates are 0.039 mm, 0.049 mm, and 0.052 mm, respectively. It is demonstrated that the proposed method is capable of separating multimodal UGWs even under significantly overlapping and low SNR conditions, further facilitating the UGW-based cortical thickness assessment.
更多查看译文
关键词
Long cortical bone,Ultrasonic guided waves,Mode separation,Nonnegative matrix factorization,Time–frequency representation
AI 理解论文
溯源树
样例
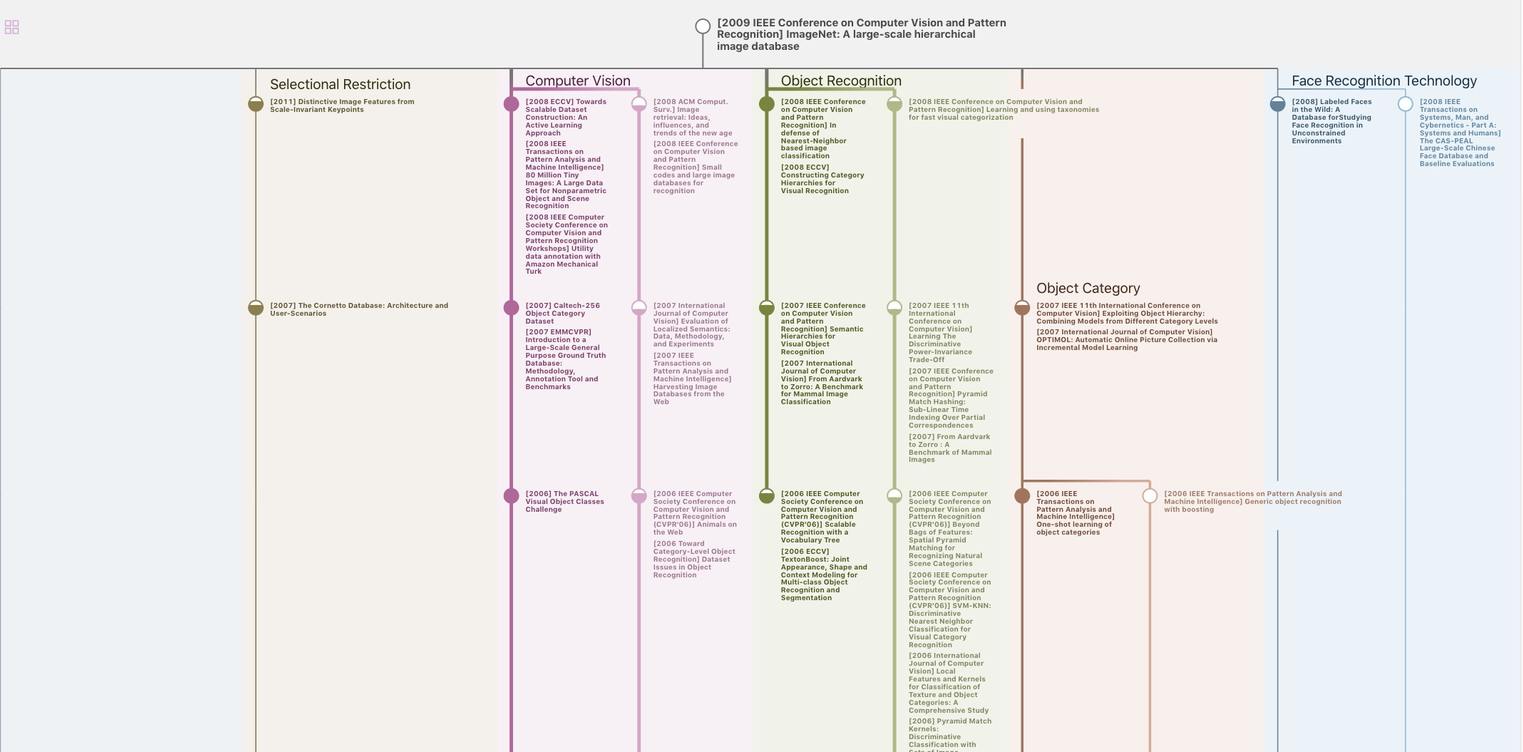
生成溯源树,研究论文发展脉络
Chat Paper
正在生成论文摘要