Generation of Simulated "Ultra-High Resolution" HiRISE Imagery Using Enhanced Super-Resolution Generative Adversarial Network Modeling
Lunar and Planetary Science Conference(2021)
摘要
Introduction: Convolutional neural networks have been used ever since the initial successes obtained in computer vision in a lot of different applications such as object detection, image recognition, semantic segmentation or super-resolution [1–3]. Single image super-resolution (SR) consists in increasing the resolution of a low resolution (LR) image to a higher resolution (HR) image. Generative adversarial networks (GANs) proved to be efficient in SR as demonstrated in [3] with Enhanced-SRGAN (ESRGAN). This architecture is now widely used in satellite imagery or in model prediction enhancement (e.g. [4, 5]). Training GANs consist in training a generator network to fool a discriminator network. In the case of SR, a HR image is downscaled to a lower resolution. The generator tries to build a fake HR image that could not be distinguished from the real one by the discriminator. This method implies that the model will only reconstruct HR images that could be already available. What is the point? In this study, we show that an ESRGAN model, can be used to generate simulated HiRISE images of higher spatial resolution than the original with high fidelity. The new details are “hallucinated” by the model. Other studies (e.g. [6, 7]) did SR for Mars imagery but to the best of our knowledge, this study is the first to use GANs on single image SR. Methods: First, we built our dataset using 49 HiRISE color images of 25 to 50 cm of spatial resolution. These images cover a wide range of geomorphological features such as dunes, craters, canyons or ancient riverbeds, which are used to show the model the textures that could be encountered on Mars. We cut each image in tiles of 1024 by 1024 pixels resulting in a total of 3520 tiles. We used a bicubic downscaling operation with a scale factor of 2 to obtain 512 by 512 pixel image tiles of 0.5 to 1 m of spatial resolution. The resulted dataset is called “DownScaled 2” (DS 2). This was done a second time. The resulted dataset, with 256 by 256 pixels images (1 to 2 m of spatial resolution) is called “DownScale 4” (DS 4). 1200, 150 and 150 images tiles were randomly chosen for the training dataset, the validation dataset and the test dataset respectively. It is important to note that the test dataset is not seen by the model during the training phase. An ESRGAN model is trained to reconstruct the DS 2 dataset from the DS 4 dataset. Peak Signal to Noise Ratio (PSNR) and Structural Similarity (SSIM) are used to quantify the degradation between the real and the fake tiles on the test datasets only. Without new training, the same model is used to reconstruct HR images from the DS 2 dataset. Again, PSNR and SSIM are used to quantify the quality of the reconstructed images. Finally, a new model is trained to build HR images from the DS 2 dataset and then, used to construct images with higher resolution than the original HiRISE dataset. The overall methodology is presented in figure 1.
更多查看译文
关键词
hirise imagery,super-resolution resolution,ultra-high
AI 理解论文
溯源树
样例
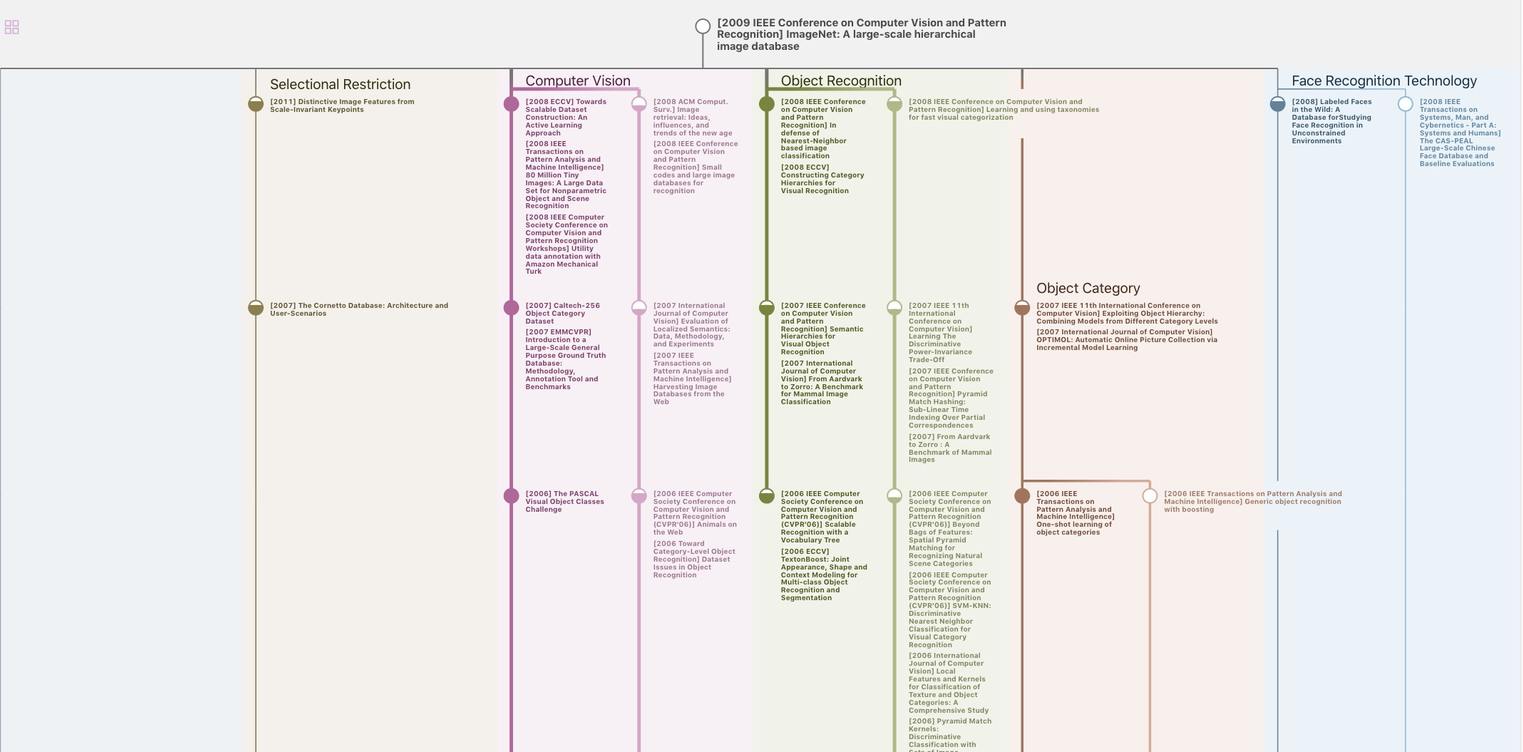
生成溯源树,研究论文发展脉络
Chat Paper
正在生成论文摘要