Submitted 28/12/18 Title: Universal continuous severity traits underlying hundreds of Parkinson’s disease clinical features Short running title: Parkinson’s disease phenotypic axes
semanticscholar(2019)
摘要
The generation of deeply phenotyped patient cohorts offers an enormous potential to identify disease subtypes with prognostic and therapeutic utility. Here, we quantify diverse Parkinson’s disease patient phenotypes on continuous scales by identifying the underlying axes of phenotypic variation using a Bayesian multiple phenotype mixed model that incorporates genotypic relationships. This approach overcomes many of the limitations associated with clustering methods and better reflects the more continuous phenotypic variation observed amongst patients. We identify three principal axes of Parkinson’s disease patient phenotypic variation which are reproducibly found across three independent, deeply and diversely phenotyped UK and US Parkinson’s disease cohorts. These three axes explain over 75% of the observed clinical variation and remain robustly captured with a fraction of the clinically-recorded features. Using these axes as quantitative traits, we identify significant overlaps in the genetic risk associated with each axis and other human complex diseases, namely coronary artery disease and schizophrenia, providing new avenues for diseasemodifying therapies. Our study demonstrates how deeply phenotyped cohorts can be used to identify latent heritable disease-modifying traits.
更多查看译文
AI 理解论文
溯源树
样例
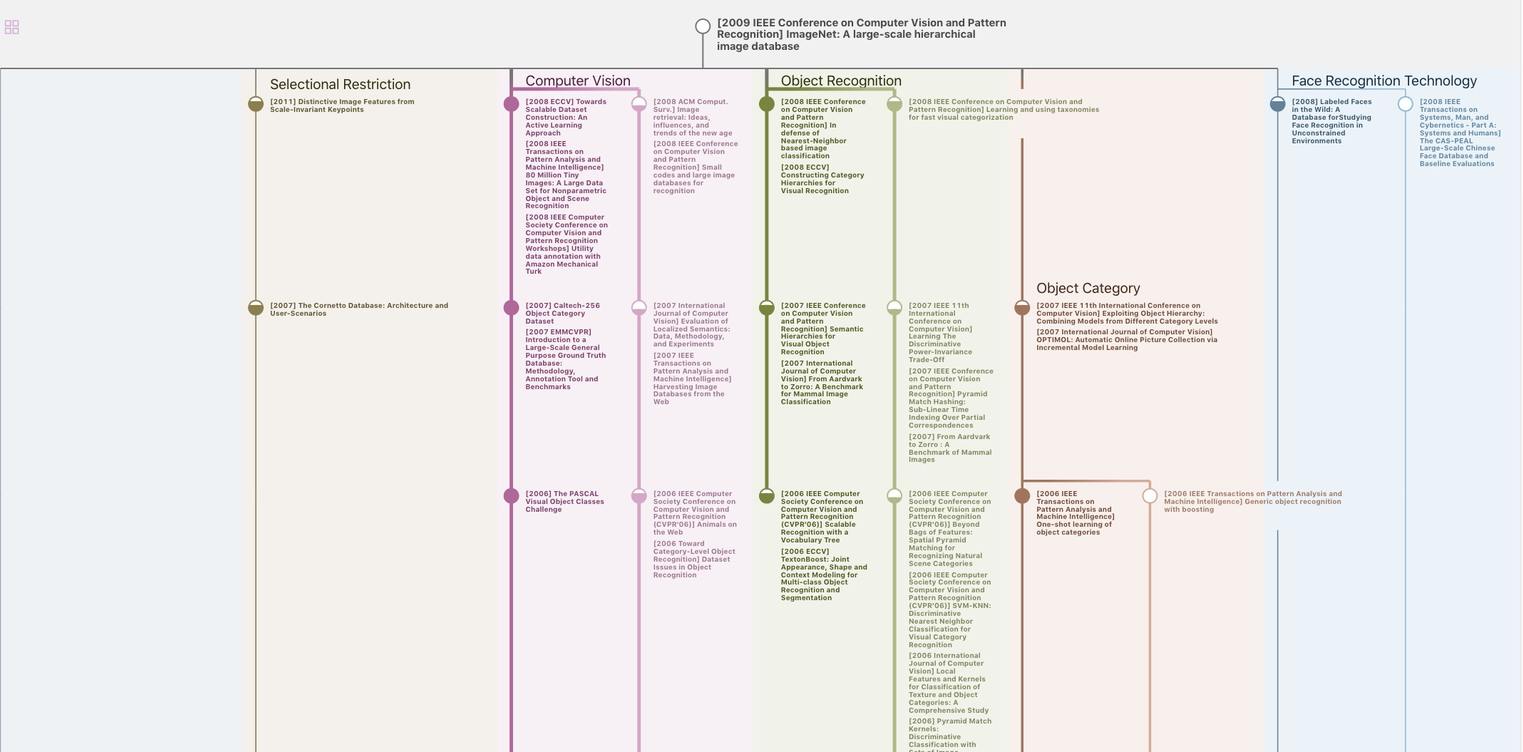
生成溯源树,研究论文发展脉络
Chat Paper
正在生成论文摘要