DermX: a Dermatological Diagnosis Explainability Dataset
semanticscholar(2021)
摘要
In this paper, we introduce DermX: a novel dermatological diagnosis and expla1 nations dataset annotated by eight board-certified dermatologists. To date, public 2 datasets for dermatological applications have been limited to diagnosis and lesion 3 segmentation, while validation of dermatological explainability has been limited to 4 visual inspection. As such, this work is a first release of a dataset providing gold 5 standard explanations for dermatological diagnosis to enable a quantitative evalua6 tion of ConvNet explainability. DermX consists of 525 images sourced from two 7 public datasets, DermNetNZ and SD-260, spanning six of the most prevalent skin 8 conditions. Each image was enriched with diagnoses and diagnosis explanations by 9 three dermatologists. Supporting explanations were collected as 15 non-localisable 10 characteristics, 16 localisable characteristics, and 23 additional terms. Derma11 tologists manually segmented localisable characteristic and described them with 12 additional terms. We showcase a possible use of our dataset by benchmarking 13 the explainability of two ConvNet architectures, ResNet-50 and EfficientNet-B4, 14 trained on an internal skin lesion dataset and tested on DermX. ConvNet visualisa15 tions are obtained through gradient-weighted class-activation map (Grad-CAM), a 16 commonly used model visualisation technique. Our analysis reveals EfficientNet17 B4 as the most explainable between the two. Thus, we prove that DermX can be 18 used to objectively benchmark the explainability power of dermatological diagnosis 19 models. The dataset is available at https://github.com/ralucaj/dermx. 20
更多查看译文
AI 理解论文
溯源树
样例
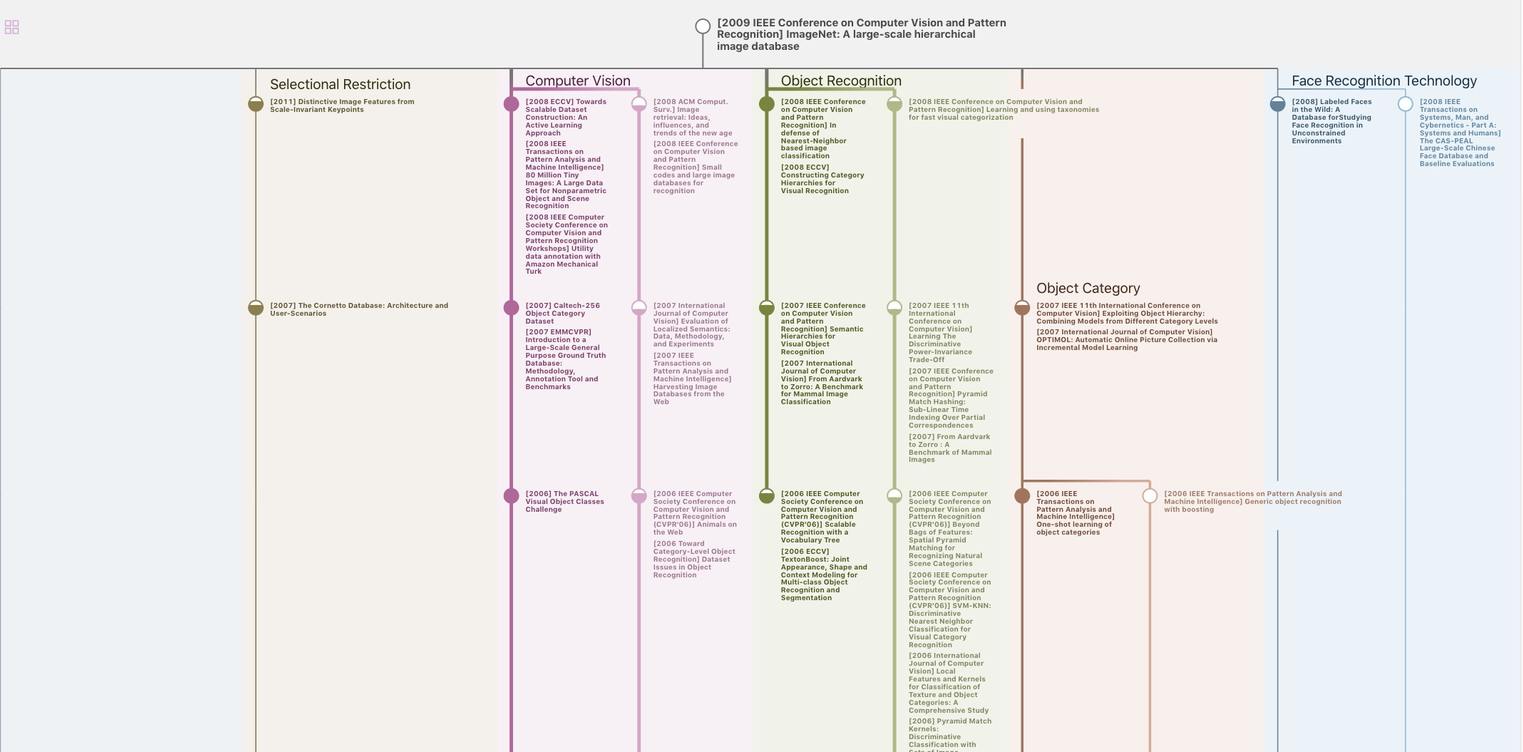
生成溯源树,研究论文发展脉络
Chat Paper
正在生成论文摘要