Application of Machine Learning and Reaction Optimization for the Iterative Improvement of Enantioselectivity of Cinchona-Derived Phase Transfer Catalysts
Organic Process Research & Development(2022)
摘要
Molecular design benefits from a strong partnership between chemical intuition and machine learning. Given the proliferation of machine learning in the small molecule catalyst space, several ideas on how best to apply and interpret various 2D or 3D methods are under discussion across the field. We undertook an investigation of several methods for modeling a catalyst system with a large training set. The synthesis of the drug letermovir involves a key conjugate addition that is promoted asymmetrically by a cinchonidine-derived “bis-quat” phase transfer catalyst. An initial data set acquired from 177 catalysts was used to drive five additional rounds of optimization based on machine learning approaches. For this specific data set, random forest with 2D molecular descriptors outperformed all other 2D methods tested, alternative descriptor combinations, and 3D-based approaches. Improvement in the model performance was observed over time, and a high-throughput approach for the synthesis of new catalysts was key to iterating through larger rounds of optimization. Optimizing reaction conditions for one of the best catalysts identified during the machine learning work led to improvement of enantioselectivity to 89%.
更多查看译文
关键词
phase transfer catalysis,PTC,machine learning,QSAR,random forest,cinchona,cinchonidine
AI 理解论文
溯源树
样例
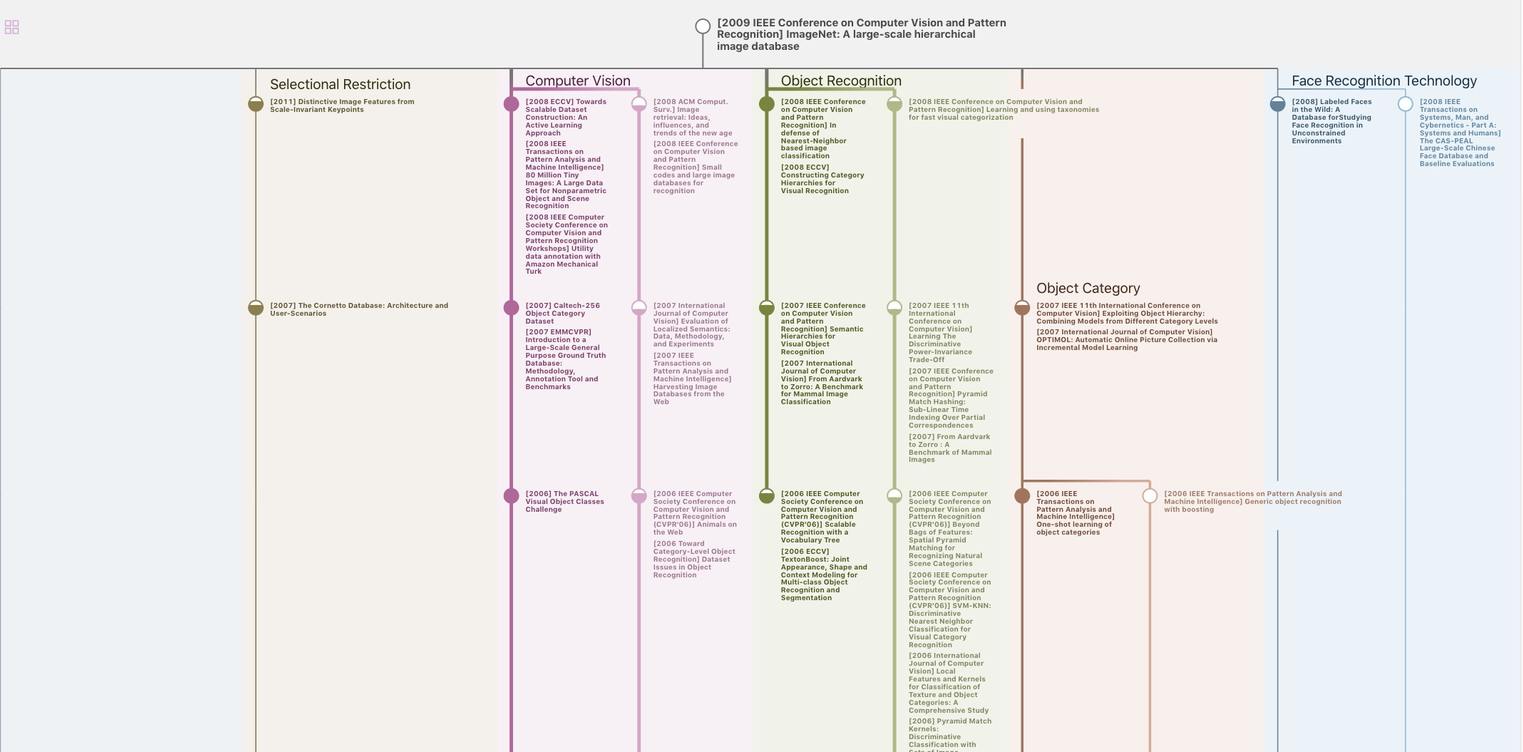
生成溯源树,研究论文发展脉络
Chat Paper
正在生成论文摘要