Monocular reconstruction of dynamic vehicles on arbitrary road profiles from a moving camera
semanticscholar(2019)
摘要
Accurate localization of other traffic participants is a vital task in autonomous driving systems. State-of-the-art systems employ a combination of sensing modalities such as RGB cameras and LiDARs for localizing traffic participants. However, with the advent and subsequent commercialization of autonomous driving, there has been an increased interest in monocular object localization (and reconstruction) for urban driving scenarios. While there have been very interesting attempts to tackle this problem using a single monocular camera, most approaches assume that the ego car and the car to be localized share the same road plane. This condition is often termed as the coplanarity assumption. In this thesis, we relax the aforementioned coplanarity assumption and demonstrate — to the best of our knowledge — the first results for monocular object localization and shape estimation on surfaces that are non-coplanar with the moving ego vehicle mounted with a monocular camera. We approximate road surfaces by local planar patches and use semantic cues from vehicles in the scene to initialize a local bundle-adjustment like procedure that simultaneously estimates the 3D pose and shape of the vehicles, and the orientation of the local ground plane on which the vehicle stands. We also demonstrate that our approach transfers from synthetic to real data, without any hyperparameter-/fine-tuning. We evaluate the proposed approach on the KITTI and SYNTHIA-SF benchmarks, for a variety of road plane configurations. The proposed approach significantly improves the state-of-the-art for monocular object localization on arbitrarily profiled/graded roads.
更多查看译文
AI 理解论文
溯源树
样例
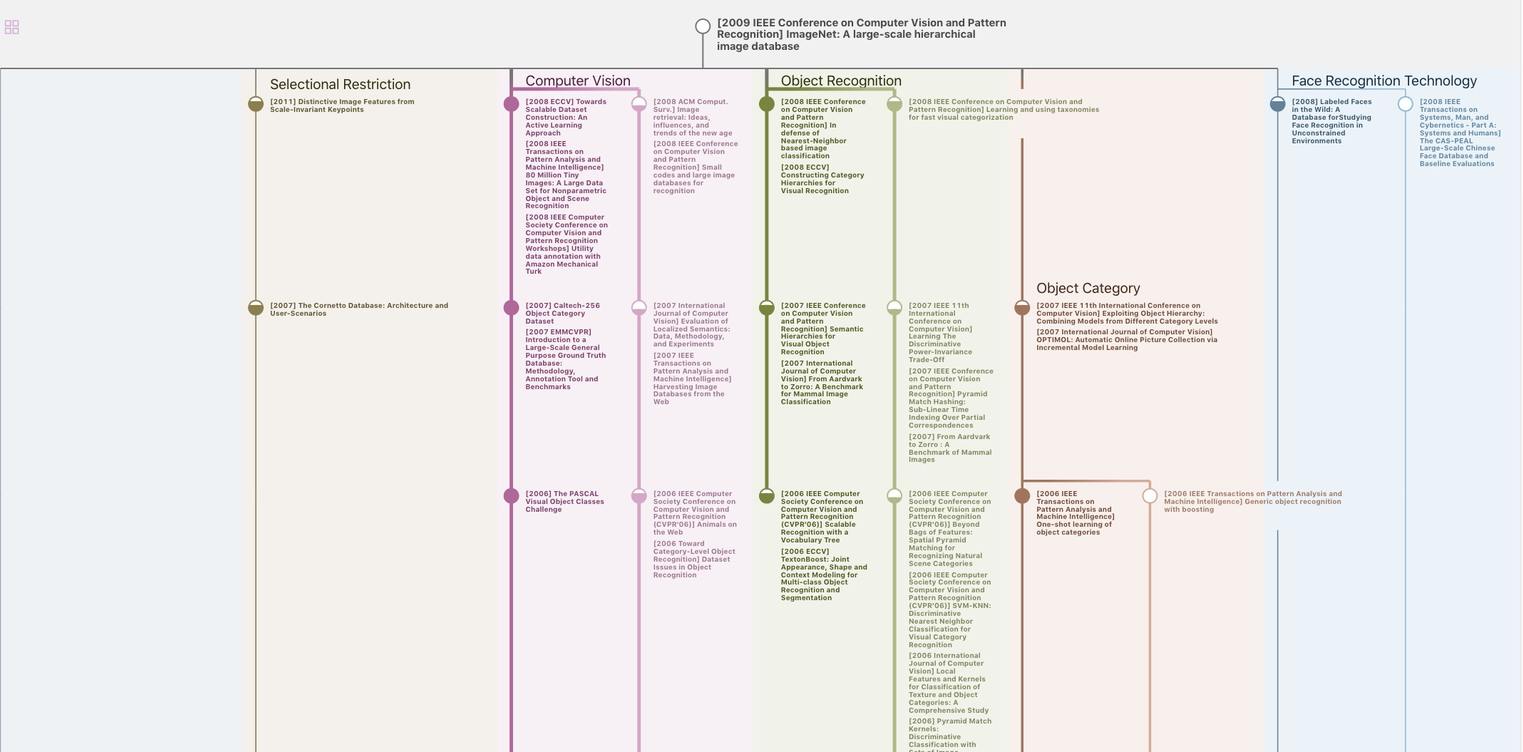
生成溯源树,研究论文发展脉络
Chat Paper
正在生成论文摘要