Non-Standard Errors
SSRN Electronic Journal(2021)
摘要
In statistics, samples are drawn from a population in a data-generating process (DGP). Standard errors measure the uncertainty in sample estimates of population parameters. In science, evidence is generated to test hypotheses in an evidence-generating process (EGP). We claim that EGP variation across researchers adds uncertainty: non-standard errors. To study them, we let 164 teams test six hypotheses on the same sample. We find that non-standard errors are sizeable, on par with standard errors. Their size (i) co-varies only weakly with team merits, reproducibility, or peer rating, (ii) declines significantly after peer-feedback, and (iii) is underestimated by participants.
更多查看译文
关键词
errors,non-standard
AI 理解论文
溯源树
样例
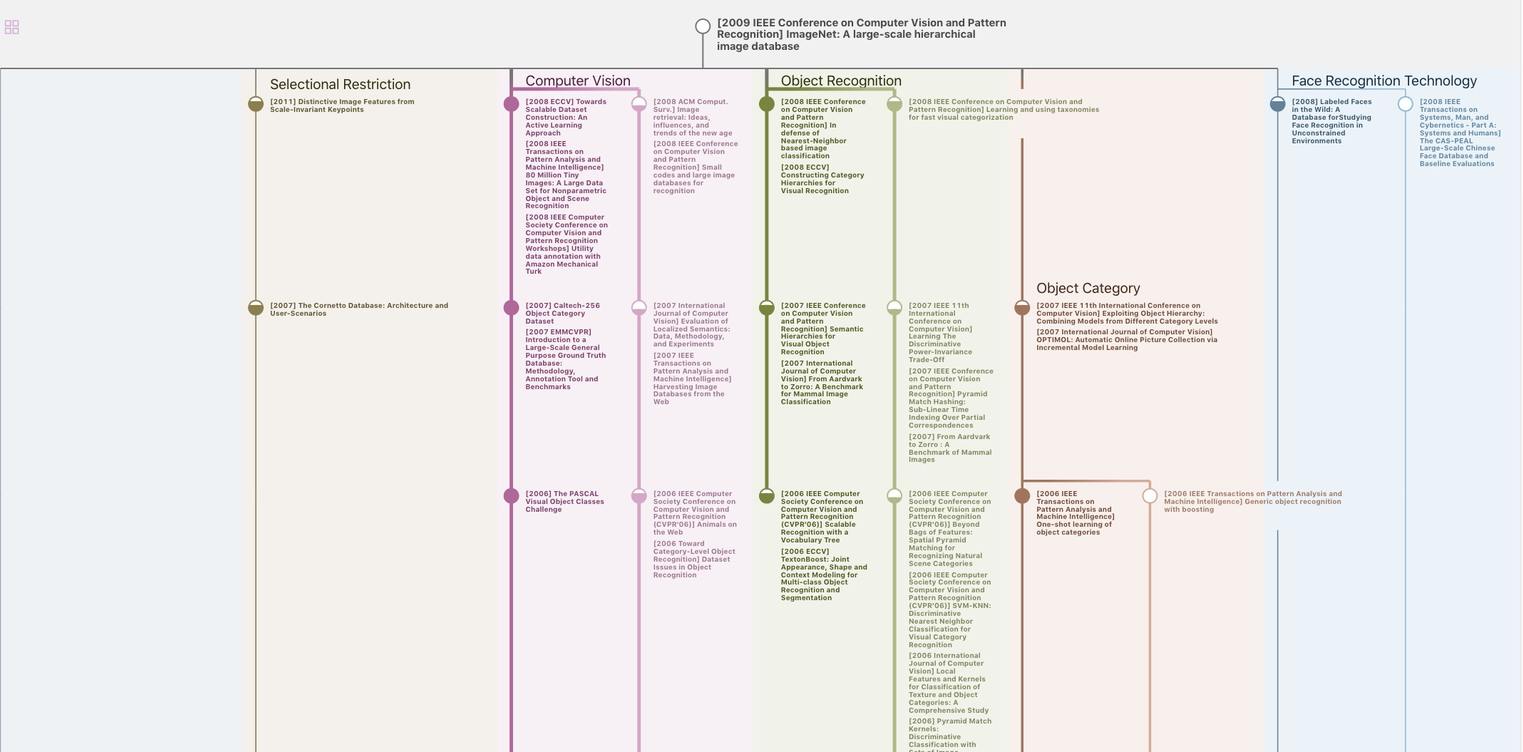
生成溯源树,研究论文发展脉络
Chat Paper
正在生成论文摘要