Low-Resource Event Extraction via Share-and-Transfer and Remaining Challenges
Computational Analysis of Storylines(2021)
摘要
Event Extraction aims to find who did what to whom, when and where from unstructured data. Over the past decade, research in event extraction has made advances in three waves. The first wave relied on supervised machine learning models trained from a large amount of manually annotated data and manually crafted features. The second wave eliminated this method of feature engineering by introducing deep neural networks with distributional semantic embedding features, but still required large annotated datasets. This chapter provides an overview of a third wave with a share-and-transfer framework, that further enhances the portability of event extraction by transferring knowledge from a high-resource setting to another low-resource setting, reducing the need there for annotated data. We describe three low-resource settings: a new domain, a new language, or a new data modality. The first share step of our approach is to construct a common structured semantic representation space into which these complex structures can be encoded. Then, in the transfer step of the approach, we can train event extractors over these representations in high-resource settings and apply the learned extractors to target data in the low-resource setting. We conclude the a Supported by ARL NS-CTA No. W911NF-09-2-0053, DARPA KAIROS Program # FA8750-19-2-1004, U.S. DARPA LORELEI Program # HR0011-15-C-0115, U.S. DARPA AIDA Program # FA8750-18-2-0014, Air Force No. FA8650-17-C-7715, and the Office of the Director of National Intelligence (ODNI), Intelligence Advanced Research Projects Activity (IARPA), via contract # FA8650-17-C-9116. The views and conclusions contained herein are those of the authors and should not be interpreted as necessarily representing the official policies, either expressed or implied, of DARPA, ODNI, IARPA, or the U.S. Government. The U.S. Government is authorized to reproduce and distribute reprints for governmental purposes notwithstanding any copyright annotation therein. 2Low-resource Event Extraction via Share-and-Transfer and Remaining Challenges chapter with a summary of the current status of this new framework and point to remaining challenges and future research directions to address them.
更多查看译文
AI 理解论文
溯源树
样例
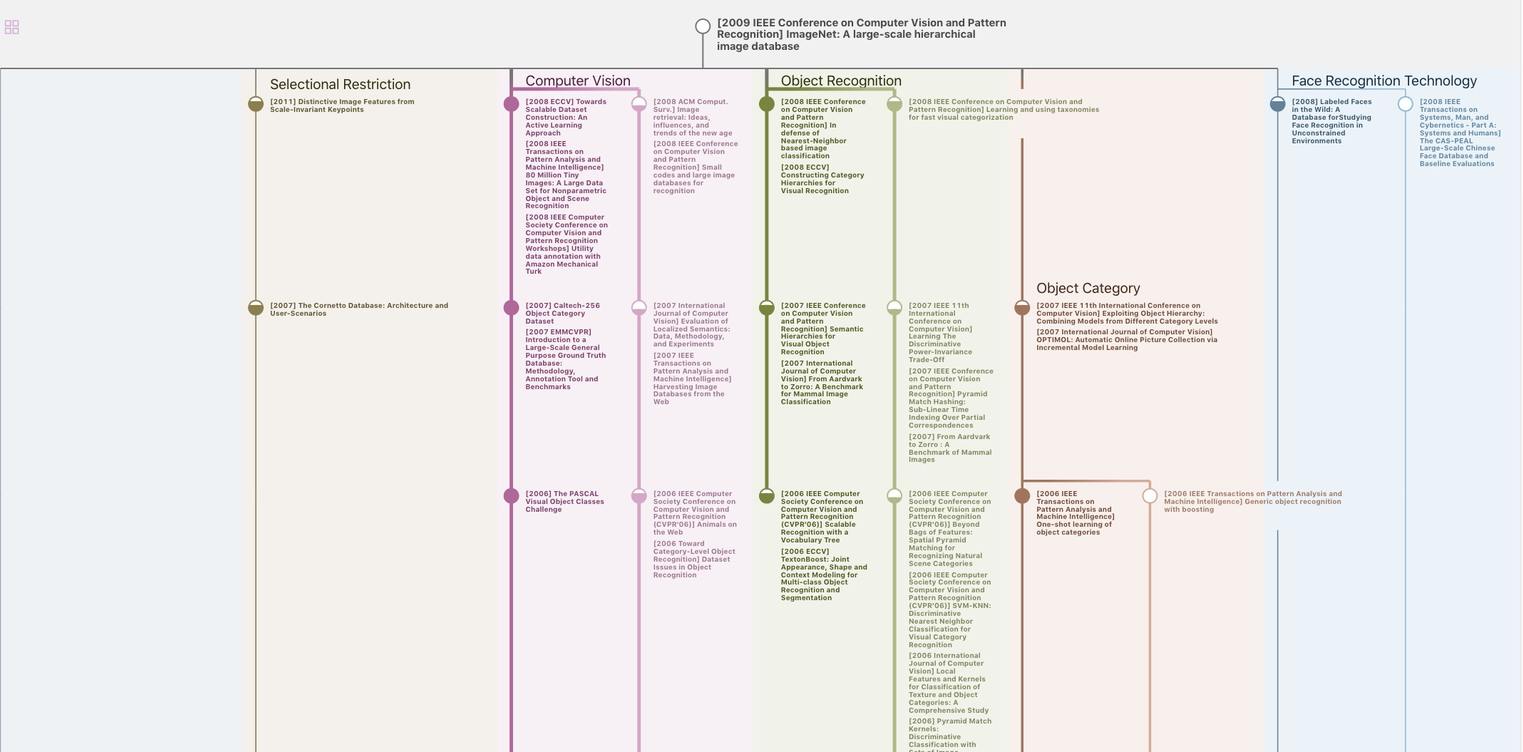
生成溯源树,研究论文发展脉络
Chat Paper
正在生成论文摘要