Cell-Type Annotation Priors for scRNA-seq
semanticscholar(2021)
摘要
Variational autoencoders (VAEs) are popular for interpreting scRNA-seq data. However, the unimodal prior distribution they typically assume is unrealistic: cells in most scRNA-seq datasets cluster by cell type in the posterior distribution. This model misspecification harms performance on downstream tasks. To address this problem, we propose capVI, a VAE that uses a hierarchical prior with one mode per cell type. To ensure that each mode corresponds to exactly one cell type, capVI leverages known cell-type annotations to pre-train a classifier. This classifier is then incorporated into a VAE-style encoder-decoder network which is trained end-to-end with unannotated scRNA-seq data. We validated capVI using two datasets: a public dataset containing scRNA-seq measurements for 11k peripheral blood mononuclear cells (PBMCs) and a second dataset containing scRNA-seq measurements for 41k PBMC cells which were sequenced and annotated by Immunai. For both datasets, capVI substantially outperformed both a popular VAE-based method (scVI) and Scanorama in terms of cLISI, a metric describing the extent to which cells are separated by cell type in latent space. UMAP-based visualizations confirmed that capVI tightly clusters cells of the same type while separating cells of different types.
更多查看译文
AI 理解论文
溯源树
样例
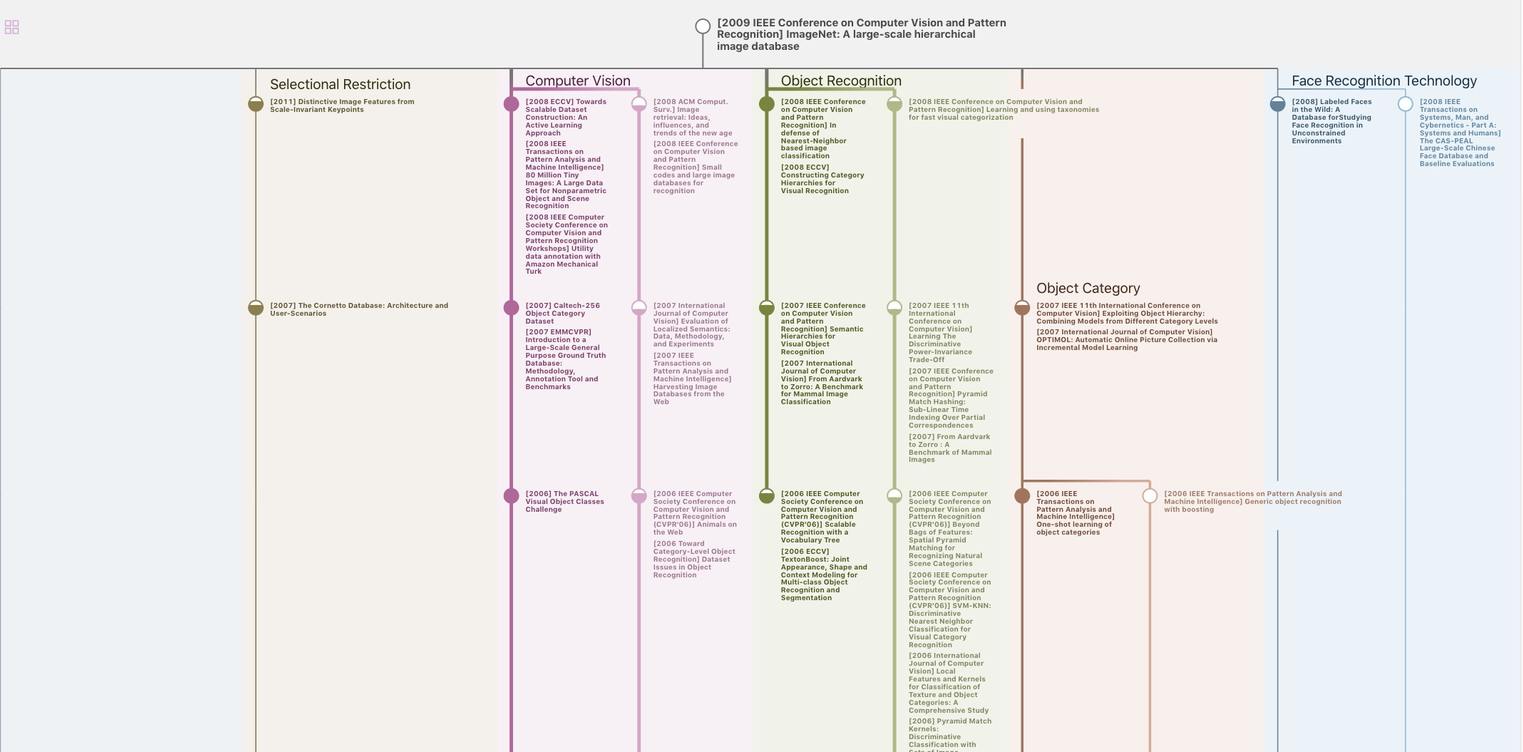
生成溯源树,研究论文发展脉络
Chat Paper
正在生成论文摘要