Generation and verification of learned stochastic automata using k -NN and statistical model checking
Applied Intelligence(2021)
摘要
Deriving an accurate behavior model from historical data of a black box for verification and feature forecasting is seen by industry as a challenging issue especially for a large featured dataset. This paper focuses on an alternative approach where stochastic automata can be learned from time-series observations captured from a set of deployed sensors. The main advantage offered by such techniques is that they enable analysis and forecasting from a formal model instead of traditional learning methods. We perform statistical model checking to analyze the learned automata by expressing temporal properties. For this purpose, we consider a critical water infrastructure that provides a scenario based on a set of input and output values of heterogeneous sensors to regulate the dam spill gates. The method derives a consistent approximate model with traces collected over thirty years. The experiments show that the model provides not only an approximation of the desired output of a feature value but, also, forecasts the ebb and flow of the sensed data.
更多查看译文
关键词
Learning automata,Stochastic automata,Statistical model checking,Temporal logic,Sensors
AI 理解论文
溯源树
样例
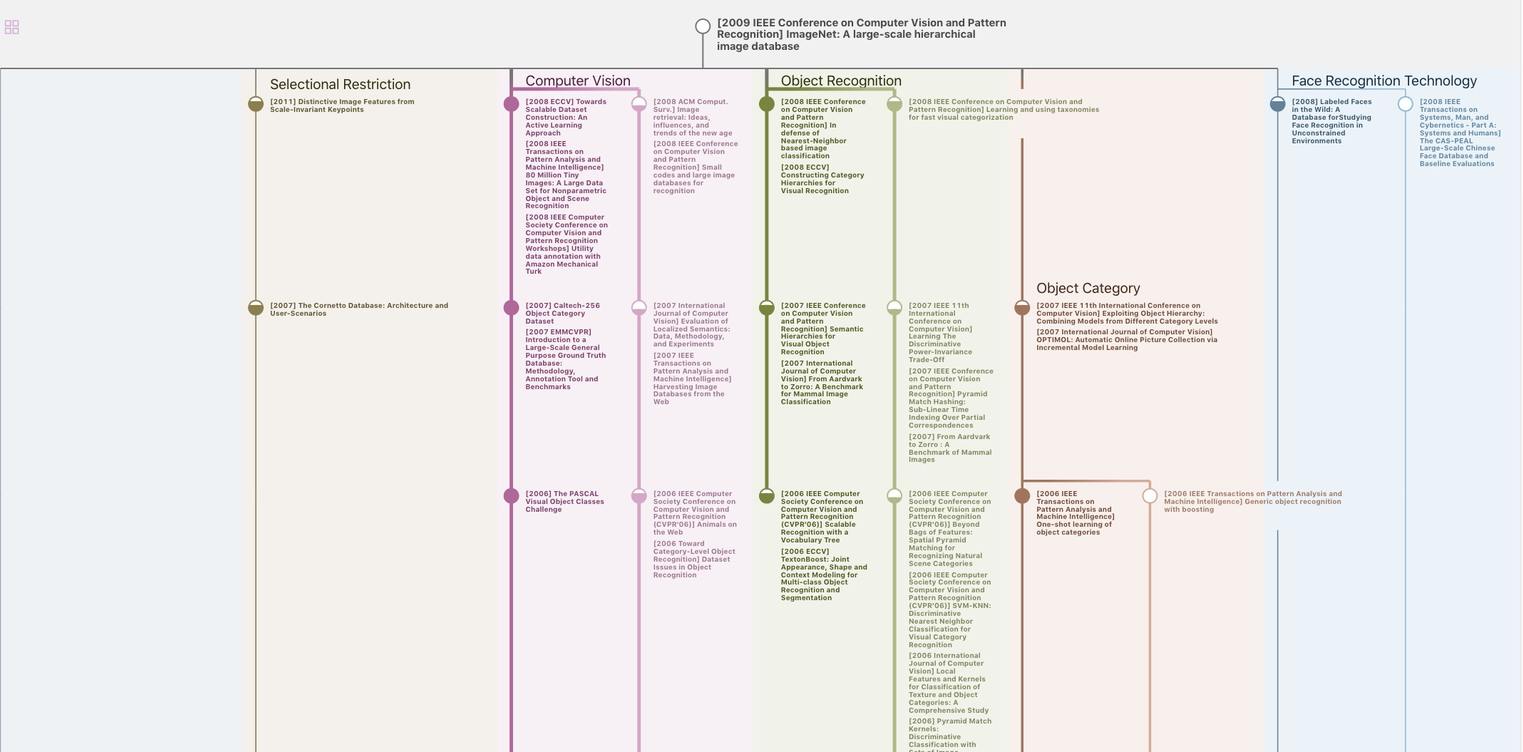
生成溯源树,研究论文发展脉络
Chat Paper
正在生成论文摘要