Revisiting Class Activation Mapping for Learning from Imperfect Data
semanticscholar(2020)
摘要
Weakly supervised object localization (WSOL) is a task of localizing an object in an image solely relying on imagelevel labels. To tackel the WSOL problem, most previous studies have employed a class activation mapping (CAM). Despite its universal use, in this work, we demonstrate it suffers from three fundamental issues: (i) the bias of GAP to assign a higher weight to a channel with a small activation area, (ii) negatively weighted activations inside the object regions and (iii) instability from the use of maximum value of a class activation map as a thresholding reference. They collectively cause the problem that the localization prediction to be highly limited to the small region of an object. We propose three simple but robust techniques that alleviate the problems, including thresholded average pooling, negative weight clamping and percentile as a thresholding standard. We participated in Learning from Imperfect Data (LID) 2020 challenge and won the 1st and 2nd places for weakly supervised object localization (Track 3) and semantic segmentation (Track 1).
更多查看译文
AI 理解论文
溯源树
样例
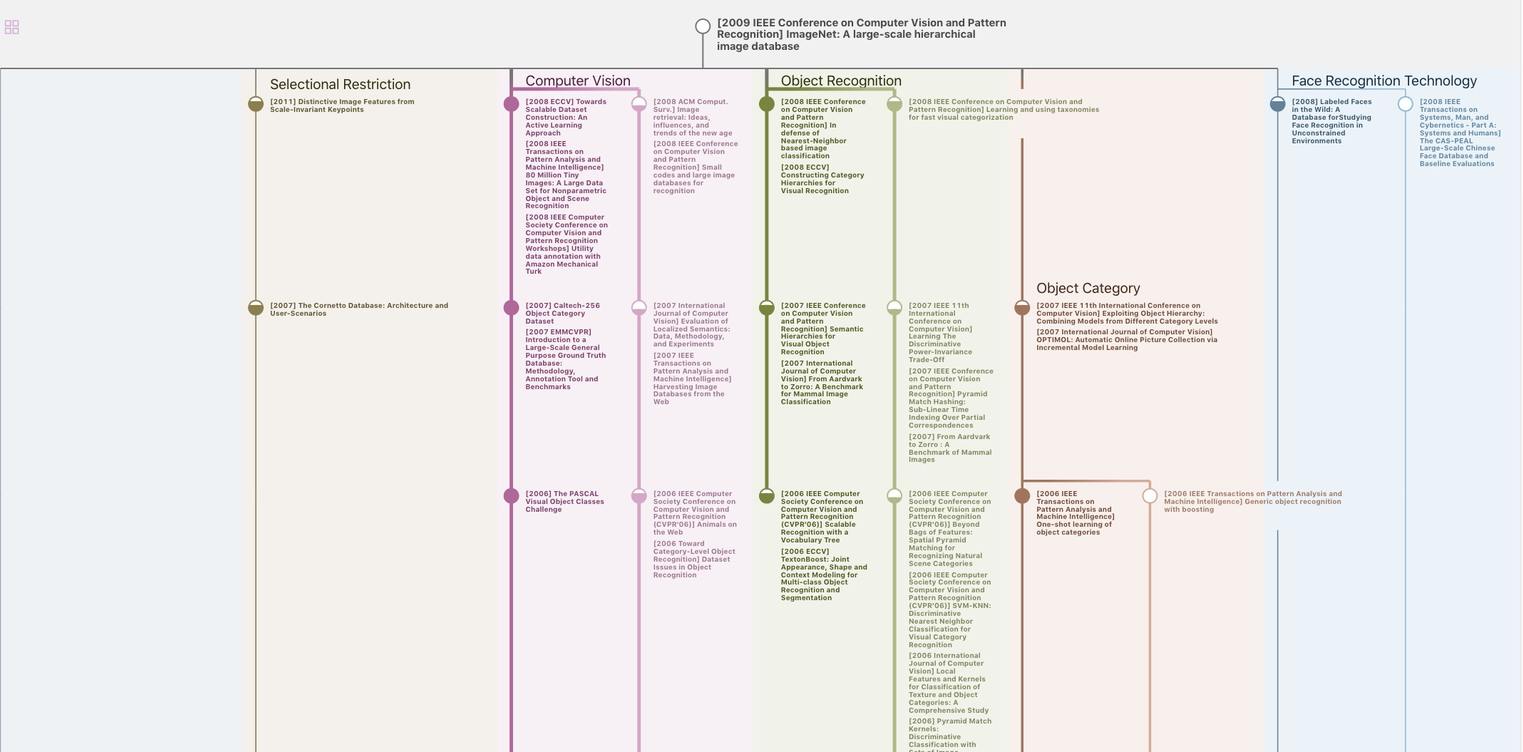
生成溯源树,研究论文发展脉络
Chat Paper
正在生成论文摘要