Optimization of an Automated Examination Generation System Using Hybrid Recurrent Neural Network
Computing Methodology eJournal(2020)
摘要
Globally, there has been a drastic increase in student-teacher ratio, over the years, which has led to the popularity of the digital examination system over classrooms, which is also eco-friendly. As a result, various new challenges have occurred due to the digitization of online education systems – for those who are not well versed with the technology, and for tracking malpractices. This issue can be acknowledged by automated examination question generation that will generate a unique exam. Using Recurrent Neural Network (RNN) models, the questions can be generated, however, the commonly used models – like, the simple RNN model, the bidirectional RNN model, the RNN model with embeddings and the encoder-decoder RNN model – do not provide high accuracies. This work proposes a hybridized RNN model that incorporates multiple layers of these commonly used RNN models, in a specific order, creating an optimized model that will be able to generate questions with higher accuracy. Additionally, this work also compares the performances of the regular RNN models with that of the hybrid model, to uphold the optimality of the system in terms of several widely accepted accuracy metrices like – precision score, recall score, F1 score, specificity score and sensitivity score. This work shows the output that can be expected from this model using a dummy data with a corpus prepared from a random online source. This system can be used in many domains ranging from offline academic areas to Massive Open Online Courses (MOOCs), and can be modified as required.
更多查看译文
AI 理解论文
溯源树
样例
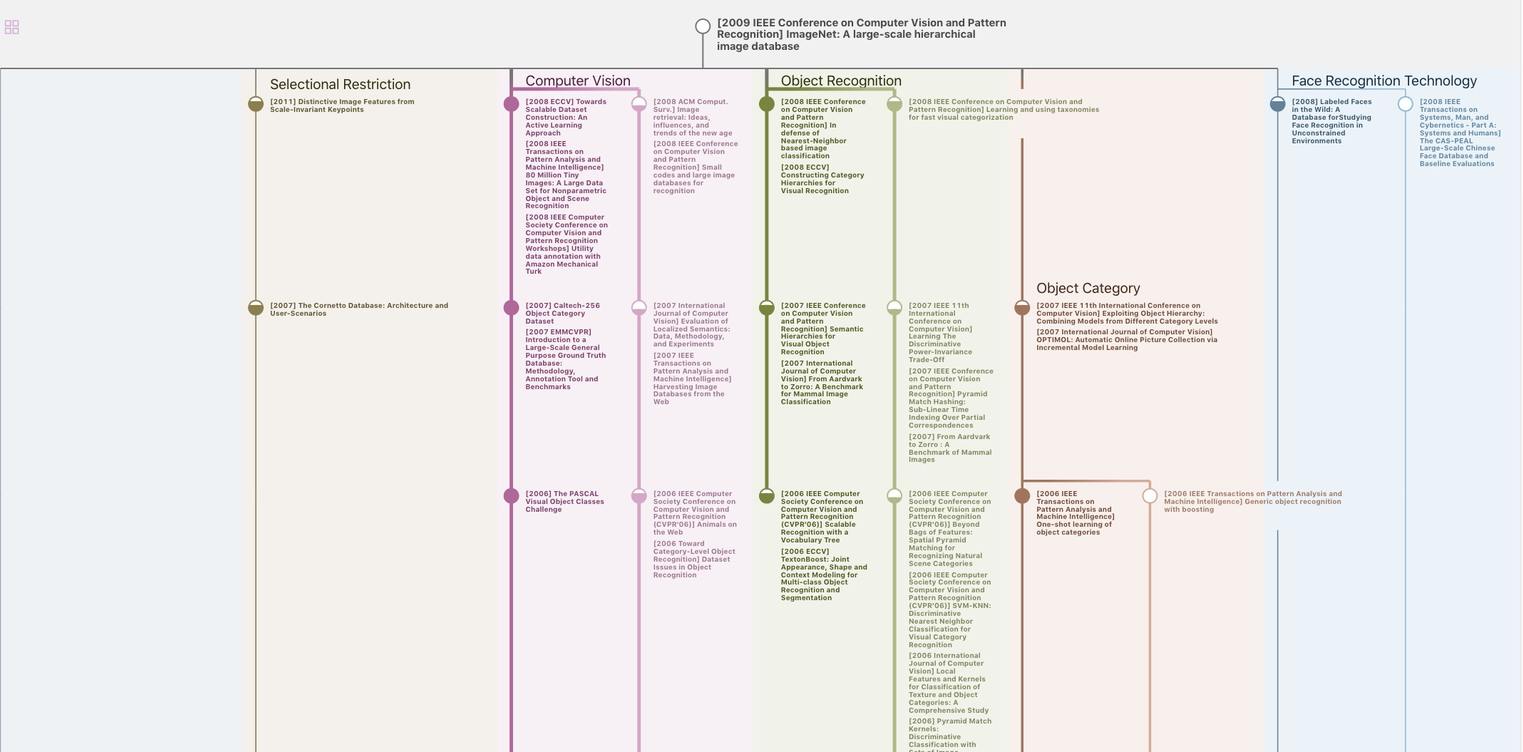
生成溯源树,研究论文发展脉络
Chat Paper
正在生成论文摘要