RAD: Rapid Automobile Data Analytics Framework for Structured Data
semanticscholar(2020)
摘要
Machine learning models are useful for vehicle performance optimization and characterization. They can be used to forecast future events or conditions, classify events as cautious or concerning, and act as a prognostic tool. Currently, supporting these machine learning models requires analytical computations and analysis of raw data collected from vehicles in the cloud. This incurs a large cost associated with transferring large amounts of data to (and from) the cloud to train and run models. Alternatively, models could be executed on board the vehicle. The compromise is that there are limited resources available on an automotive electronic control unit (ECU) and the architecture is currently decentralized to perform these computations. Therefore, any deployed models would need a model execution environment that uses limited computing resources. In this scenario it is important to also consider the trade-off between resources and performance. In this paper, we develop a unified framework enabling rapid deployment of flexible machine learning models to handle a variety of use-cases in constrained environments called the Rapid Automobile Data Analytics (RAD) framework. This paper focuses primarily on creating models and architectures for sequential and structured data. Multiple architectures and models are investigated and evaluated, and an automated pipeline for deployment of the models is developed.
更多查看译文
AI 理解论文
溯源树
样例
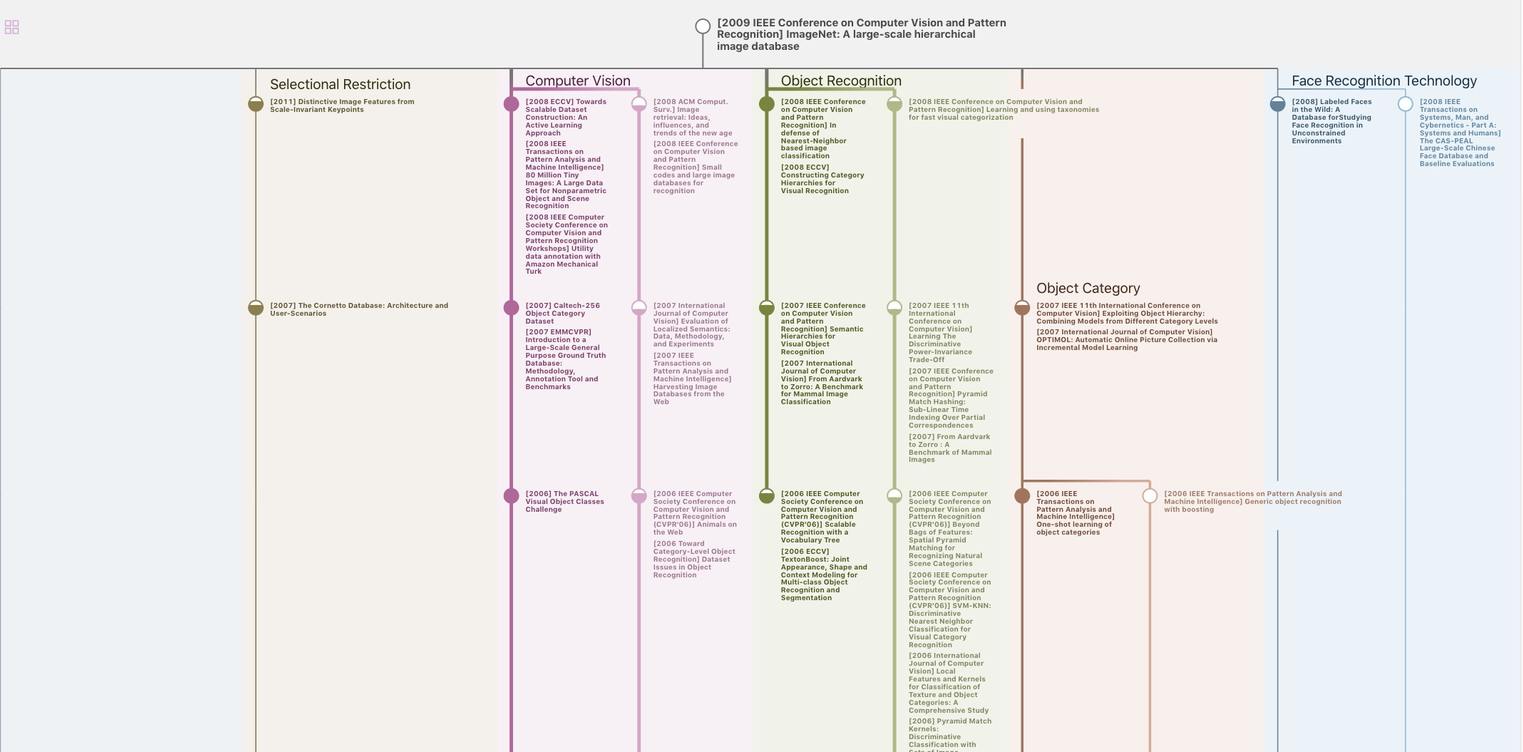
生成溯源树,研究论文发展脉络
Chat Paper
正在生成论文摘要