Improved Estimator Selection for Off-Policy Evaluation
semanticscholar(2021)
摘要
Off-policy policy evaluation is a fundamental problem in reinforcement learning. As a result, many estimators with different tradeoffs have been developed; however, selecting the best estimator is challenging with limited data and without additional interactive data collection. Recently, Su et al. (2020b) developed a datadependent selection procedure that competes with the oracle selection up to a constant and demonstrate its practicality. We refine the analysis to remove an extraneous assumption and improve the procedure. The improved procedure results in a tighter oracle bound and stronger empirical results on a contextual bandit task.
更多查看译文
AI 理解论文
溯源树
样例
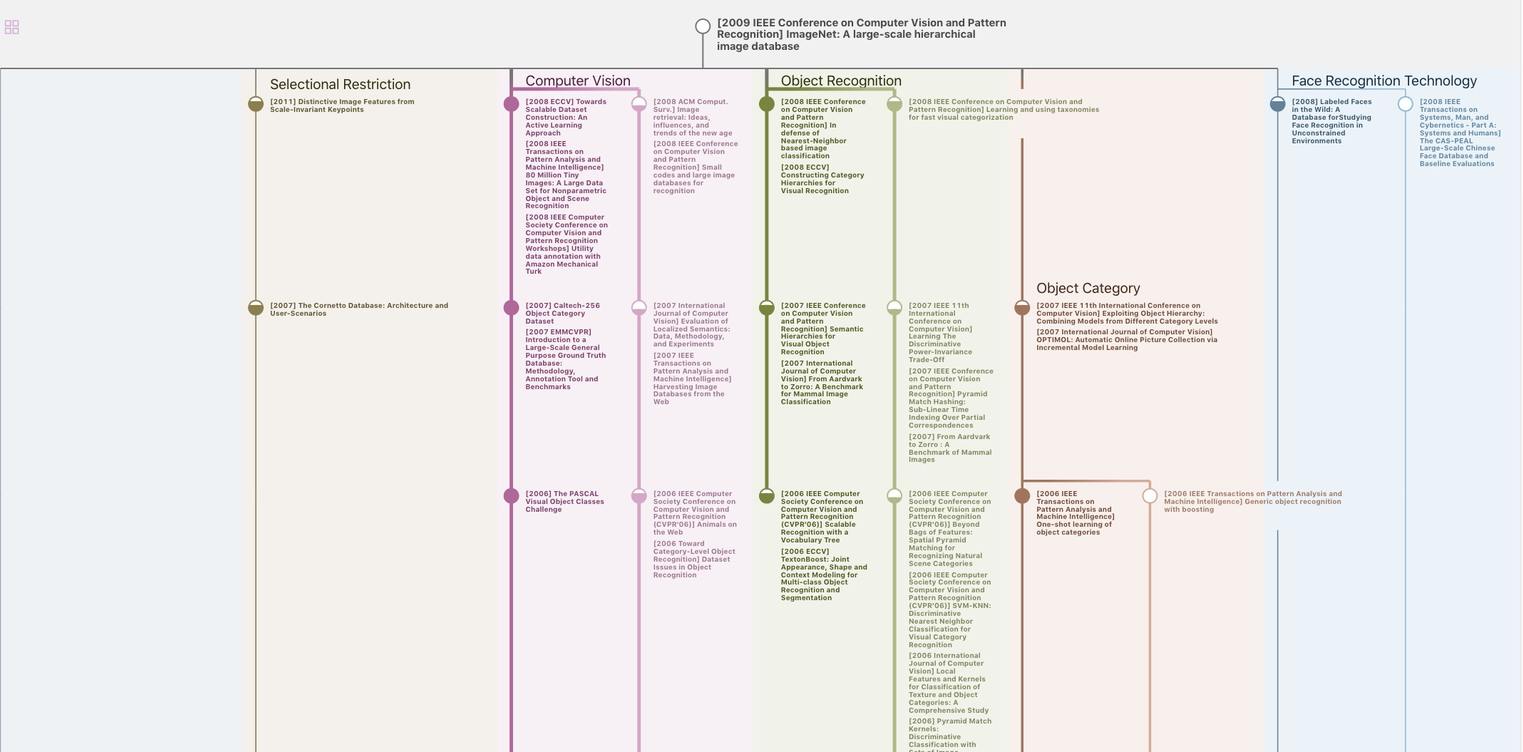
生成溯源树,研究论文发展脉络
Chat Paper
正在生成论文摘要