A Nonparametric Bayesian Model for Sparse Dynamic Multigraphs
semanticscholar(2021)
摘要
Many large networks take the form of sequences of different types of interactions between entities, which can be represented as a sparse, structured, dynamically evolving multigraph. Bayesian edge-exchangeable models have been proposed as a model for sparse multigraphs, and these have been incorporated into hierarchical models that are able to capture community-like structure. However, these models assume exchangeability of the edges, precluding us from capturing dynamic behavior, such as the tendency of individuals to reply to recent emails. To capture evolving graph dynamics, we propose a dynamic Bayesian nonparametric model for interaction networks that tends to reinforce recent behavioral patterns. Our Dynamic Nonparametric Network Distribution (DNND) describes a generative distribution over sequences of interactions, captured as a time-evolving mixture of dynamic behavioral patterns, that is able to capture both sparse and dense behavior. Conditioned on the hyperparameters, we are able to sample directly from the posterior distribution over the mixing components. The resulting posterior predictive distribution can be used to predict future interactions. We demonstrate impressive predictive performance against a range of state-of-the-art dynamic graph models.
更多查看译文
AI 理解论文
溯源树
样例
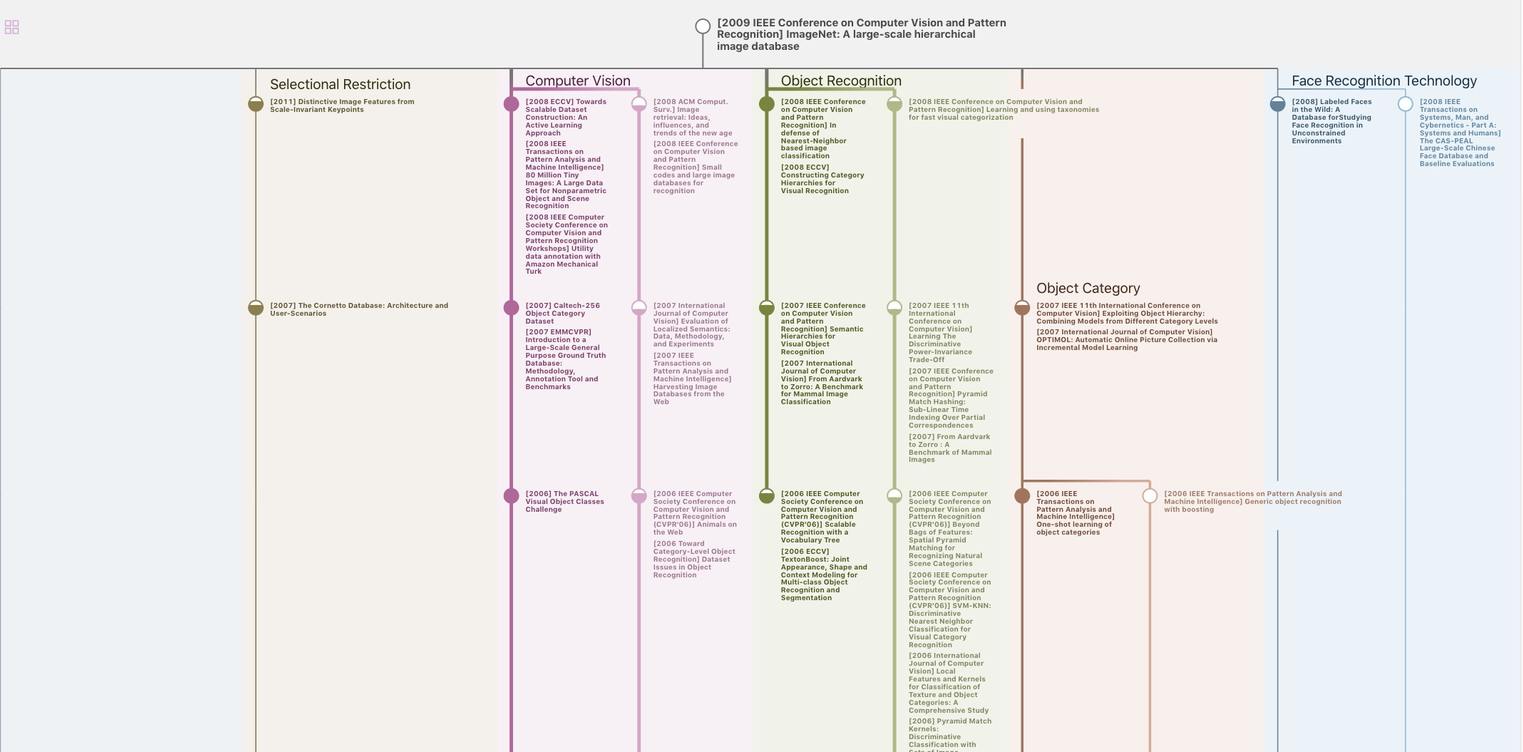
生成溯源树,研究论文发展脉络
Chat Paper
正在生成论文摘要