Information-theoretic approach to sensor selection
semanticscholar(2017)
摘要
It is important to be able to explore and estimate target state by using distributed sensing. For such sensing tasks not all measurements are equally informative. Therefore, for large sensor networks the relevant data can either be extracted by removing correlated measurements in post statistical analysis, or by limiting the number of selected sensors. The first method is computationally intensive (especially for the case of a moving target) when fast successive measurements are desired. Therefore, a sensing task should employ more sensors than necessary, and only use the ones that provide the most information. From an information-theoretic perspective each sensor is tasked with observing the target and reducing the ambiguity of target state. The information gain associated to each sensor can be different if the system is anisotropic (Fig. 1D). Therefore, repeatedly selecting sensors that are most informative reduces the overall uncertainty of the target parameters. This allows us to represent the problem as choosing k sensors among m possible sensors to minimize the error of estimating target state.
更多查看译文
AI 理解论文
溯源树
样例
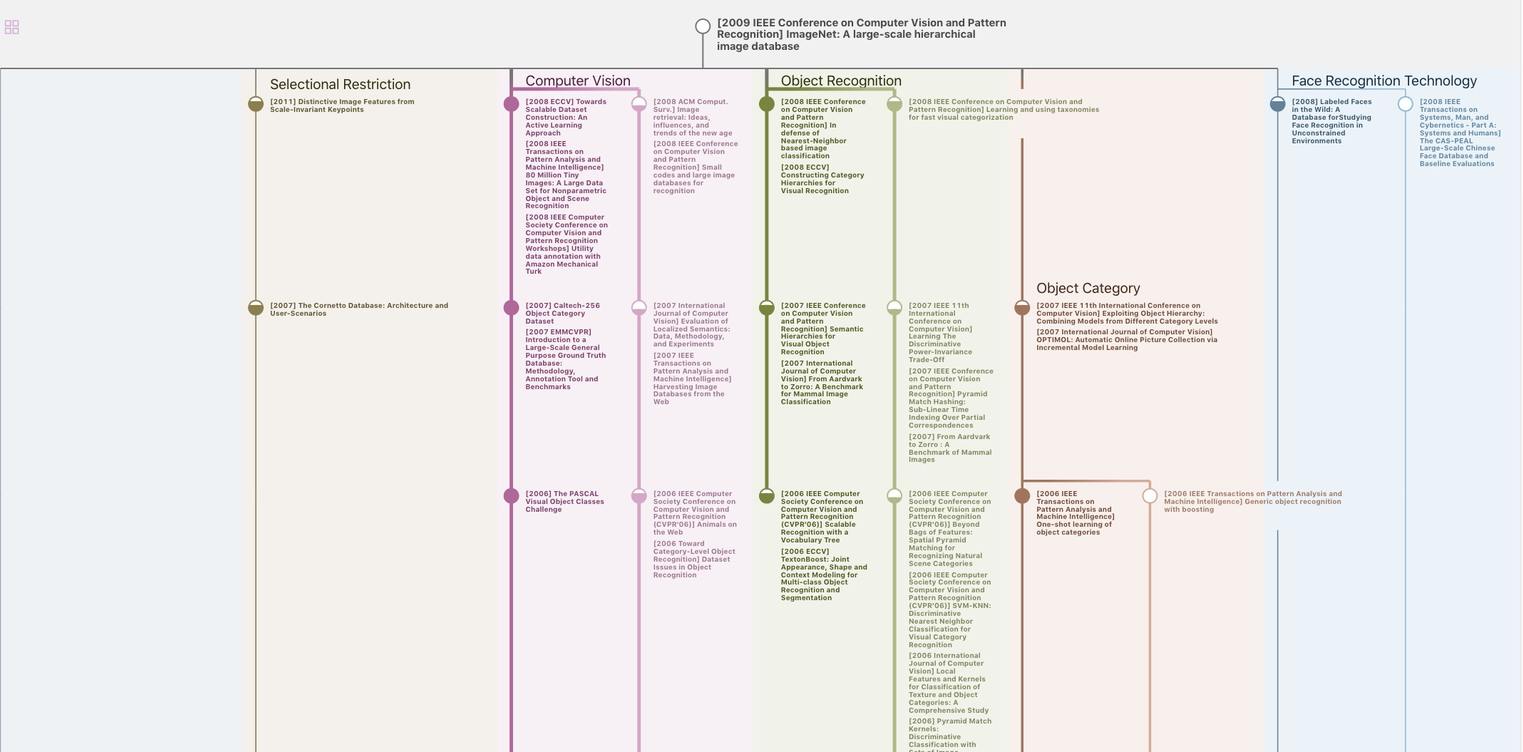
生成溯源树,研究论文发展脉络
Chat Paper
正在生成论文摘要