Sketch Your Own GAN Supplemental Material
semanticscholar(2021)
摘要
Training details We use the same training hyperparameters as [5]. In particular, we are using softplus for GAN loss, and R1 regularization [7] on both the sketch and image discriminator, DY and DX . We do not use path length regularization, as it has no effect on the latent mapping network. Also, we set the batch size to 4 for all of our experiments, except when the sketch inputs are less than four, where we set the batch size to 1. Hyperparameters. We use the same hyperparameters for our full method in all of our experiments. In particular, we use λimage = 0.7 In the main text (Sec 4.1), we compared several variants of our method in our ablation studies. To make the comparison fair, for each variant, we tuned the loss weights for optimal performance. In Table 2, we list the hyperparameters used for each variant. The only exception is that we use λweight = 50 for the Lsketch + Lweight and Lsketch + Lweight + aug. variant model trained on the standing cat task. Also, if the variants are not listed in the table, the same loss weights as the full method are used. The search space of the λimage is [0.3, 0.5, 0.7, 1.0], and the search space of λweight is [0.1, 1, 10, 50, 100, 1000]. Data collection. In the main text (Sec. 4.1), we selected sets of 30 sketches with similar shapes and poses to designate as the user input: examples of sketches from these sets are shown in Figure 1. To evaluate generation quality, we collected images that match the input sketches from LSUN [10]. To retrieve matching images, we experimented with two sketch-image cross-domain matching methods. We applied both the SBIR method of Bui et al. [2] and chamfer distance [1]. Both of these retrieval results are shown in Figure 2. We observe that with chamfer distance, the retrieved images match poses of the sketches more faithfully. As a result, we adopt this method to generate our evaluation sets. However, we notice that there still exists outliers after the retrieval; hence, we hand-selected 2,500 images out of top 10,000 matches to curate the evaluation sets. A comparison between curated dataset and top chamfer matches are shown in Figure 3. Evaluation procedure. To evaluate each model, we sample 2,500 images without truncation and save them into png files. Likewise, the evaluation set described in the main text (Sec. 4.1) consists of 2,500 256×256 images stored in png. We evaluate the Fréchet Inception Distance values using the CleanFID code [8].
更多查看译文
AI 理解论文
溯源树
样例
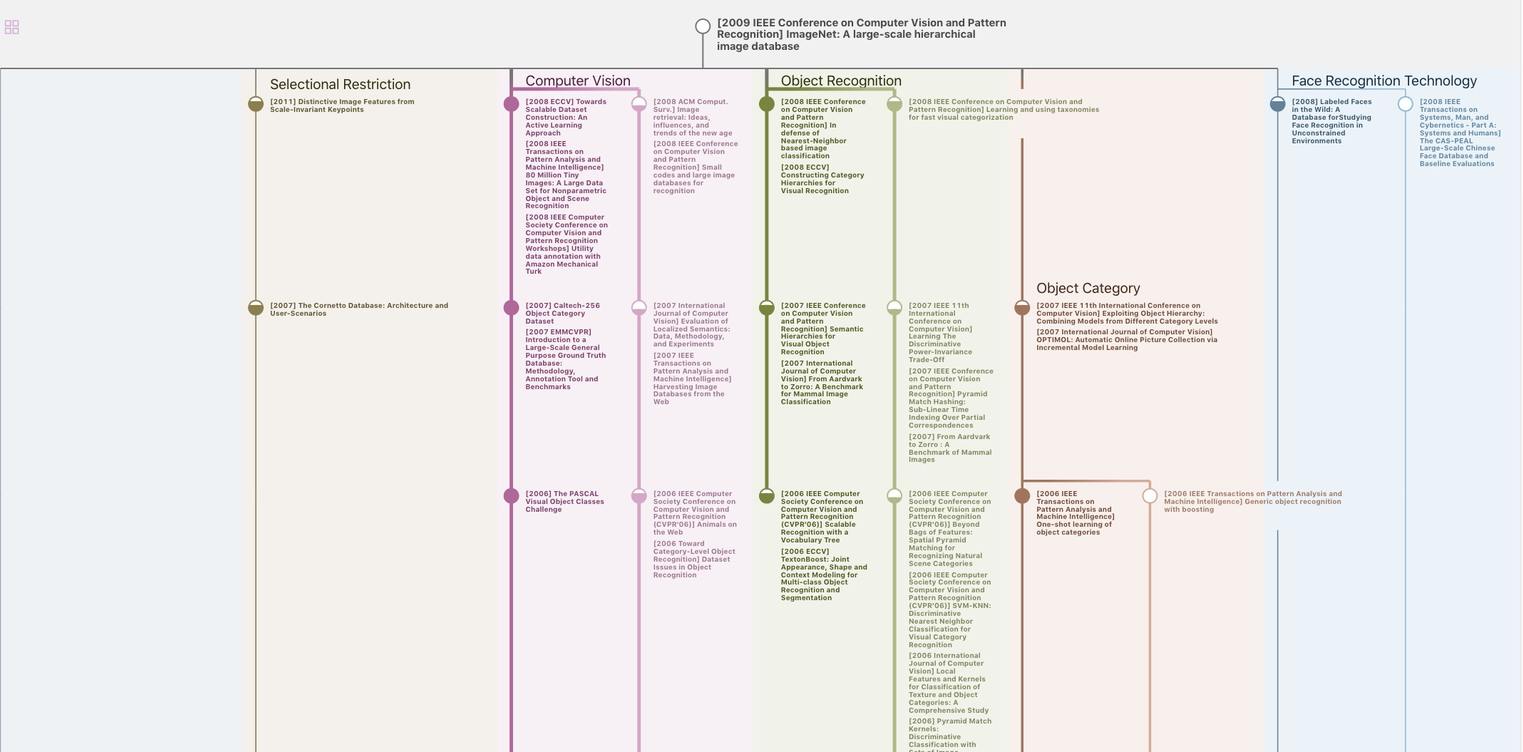
生成溯源树,研究论文发展脉络
Chat Paper
正在生成论文摘要