Decoupled self-supervised label augmentation for fully-supervised image classification
Knowledge-Based Systems(2022)
摘要
Self-supervised label augmentation has emerged as an effective means to overcome the data scarcity problem for supervised vision tasks. Existing rotation-based self-supervised label augmentation methods either impose or relax the rotation invariance constraint on the primary classifier, which omit necessary supervisory information and may degrade the classification performance depending on the given working data. To overcome this problem, we propose a decoupled self-supervised label augmentation method to enhance the feature representation for fully-supervised image classification. Experimental results on diverse datasets demonstrate that the proposed method consistently outperforms state-of-the-art data augmentation methods. The proposed method is also complementary to conventional data augmentation methods, such as AutoAugment and Fast AutoAugment.
更多查看译文
关键词
Self-supervised label augmentation,Image classification,Multi-task learning
AI 理解论文
溯源树
样例
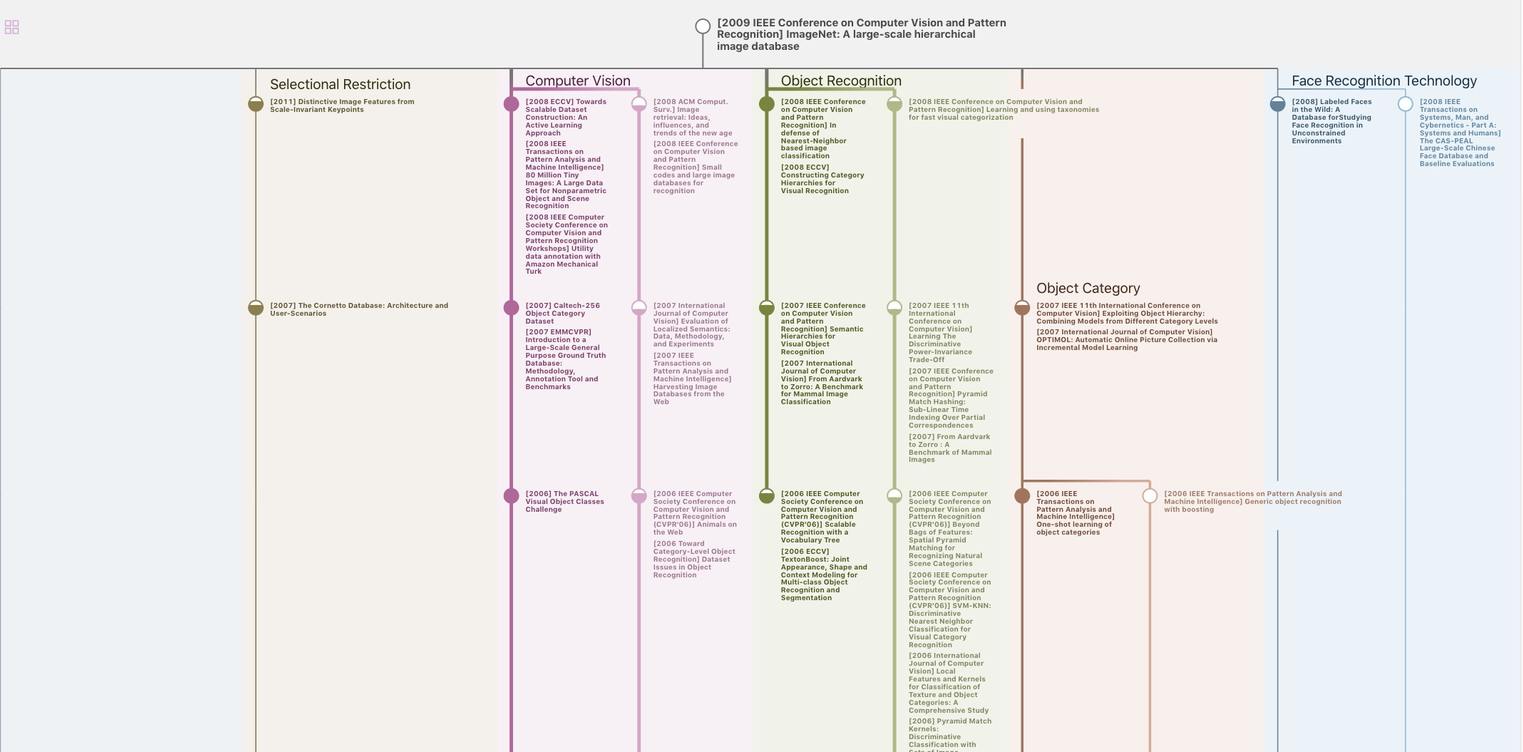
生成溯源树,研究论文发展脉络
Chat Paper
正在生成论文摘要