UnMICST: Deep learning with real augmentation for robust segmentation of highly multiplexed images of human tissues
bioRxiv(2021)
摘要
Abstract Newly developed technologies have made it feasible to routinely collect highly multiplexed (20-60 channel) images at subcellular resolution from human tissues for research and diagnostic purposes. Extracting single cell data from such images requires efficient and accurate image segmentation. This starts with identification of nuclei, a challenging problem in tissue imaging that has recently benefited from the use of deep learning. In this paper, we demonstrate two generally applicable approaches to improving segmentation accuracy for multiple human tissues. The first involves the use of “real augmentations” during training. These augmentations comprise defocused and saturated image data and improve model accuracy whereas computational augmentation (Gaussian blurring) does not. The second involves collection of nuclear envelope data to better identify nuclear outlines. The two approaches cumulatively and substantially improve segmentation with three different deep learning frameworks, yielding a set of highly accurate segmentation models. We speculate that the use of real augmentations may have applications in image processing outside of microscopy.
更多查看译文
AI 理解论文
溯源树
样例
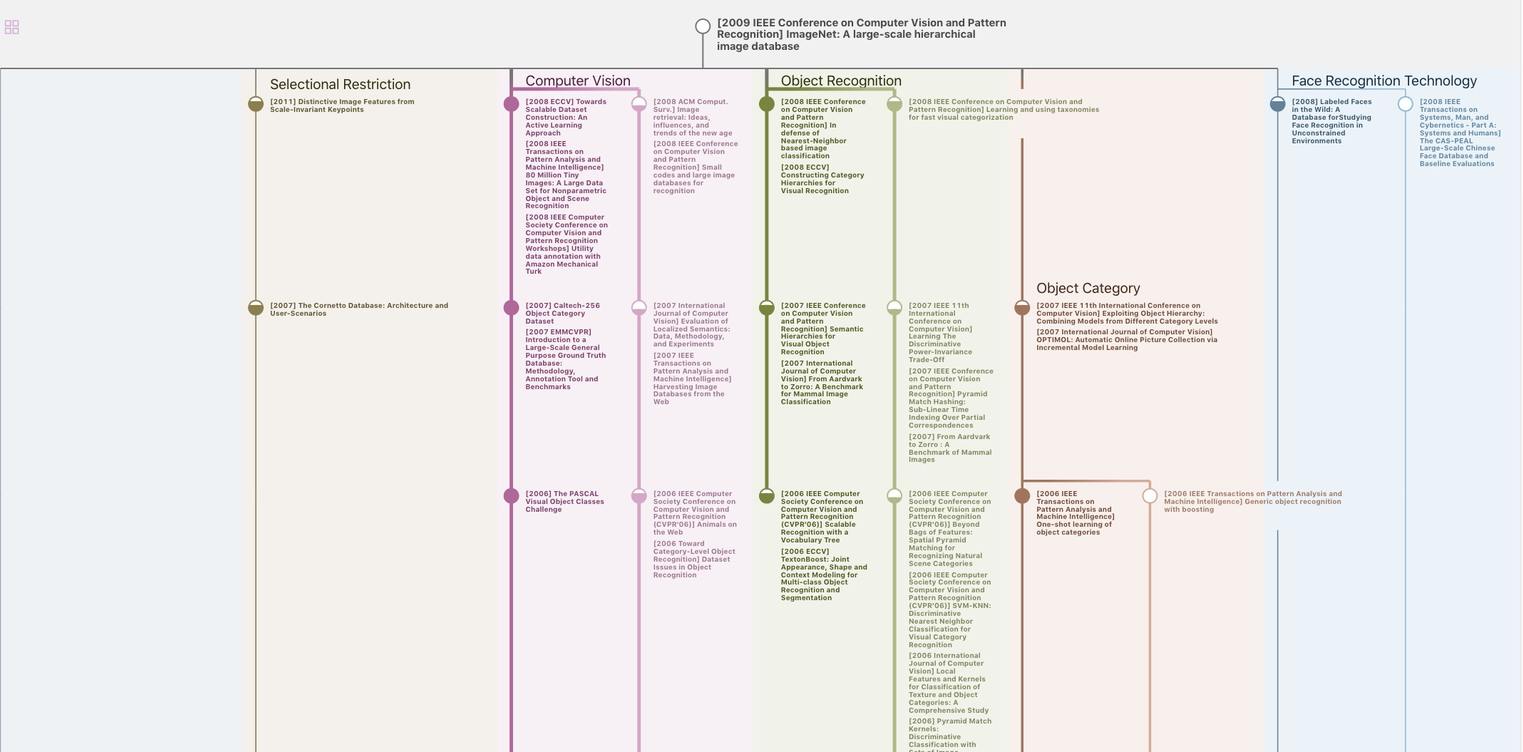
生成溯源树,研究论文发展脉络
Chat Paper
正在生成论文摘要