Reasoning by Anomaly Detection to Improve Planning Robustness for Autonomous Robots in Changing Environments
semanticscholar(2020)
摘要
The requirement for autonomous robots to exhibit higherlevel cognitive skills by planning and adapting in an ever changing environment and situation is indeed a great challenge for the AI community. In robotics task planning, the typical use of automated planners entails using fixed action descriptions that neglect the subtle differences that appear when environment change occurs or where behavioural capabilities are not adequately captured. Failure of action at execution time can be a result of a mismatch between the actions in the abstract plan and the low-level commands, because knowledge in the abstract level is incorrect or not sufficiently accurate. The choice of parameters in the low-level commands corresponding to the abstract actions, and their related information can strongly affect the success of execution. Incorrect choices may cause plan execution to fail. For a more robust robot capable of adapting to the changing environment, the goal of this project is to bridge the gap between abstract plans and robot action execution. Our approach is toplans and robot action execution. Our approach is to extend a high-level planning platform (ROSPlan) to help create more robust planning systems by using theory refinement (TR) techniques to develop intelligent robot behaviour based on experience. Refinement will involve reasoning over action execution failure using Anomaly Detection (AD) techniques. The general aims of our research are to make it possible for robot task planning to adapt to changing situations towards long-term autonomy, but still retaining some abstract theory of the environment which can be used as an explanation for behaviour. This paper reports on a crucial step in that theory refinement process: determination of the cause of failure in order to drive the changes.
更多查看译文
AI 理解论文
溯源树
样例
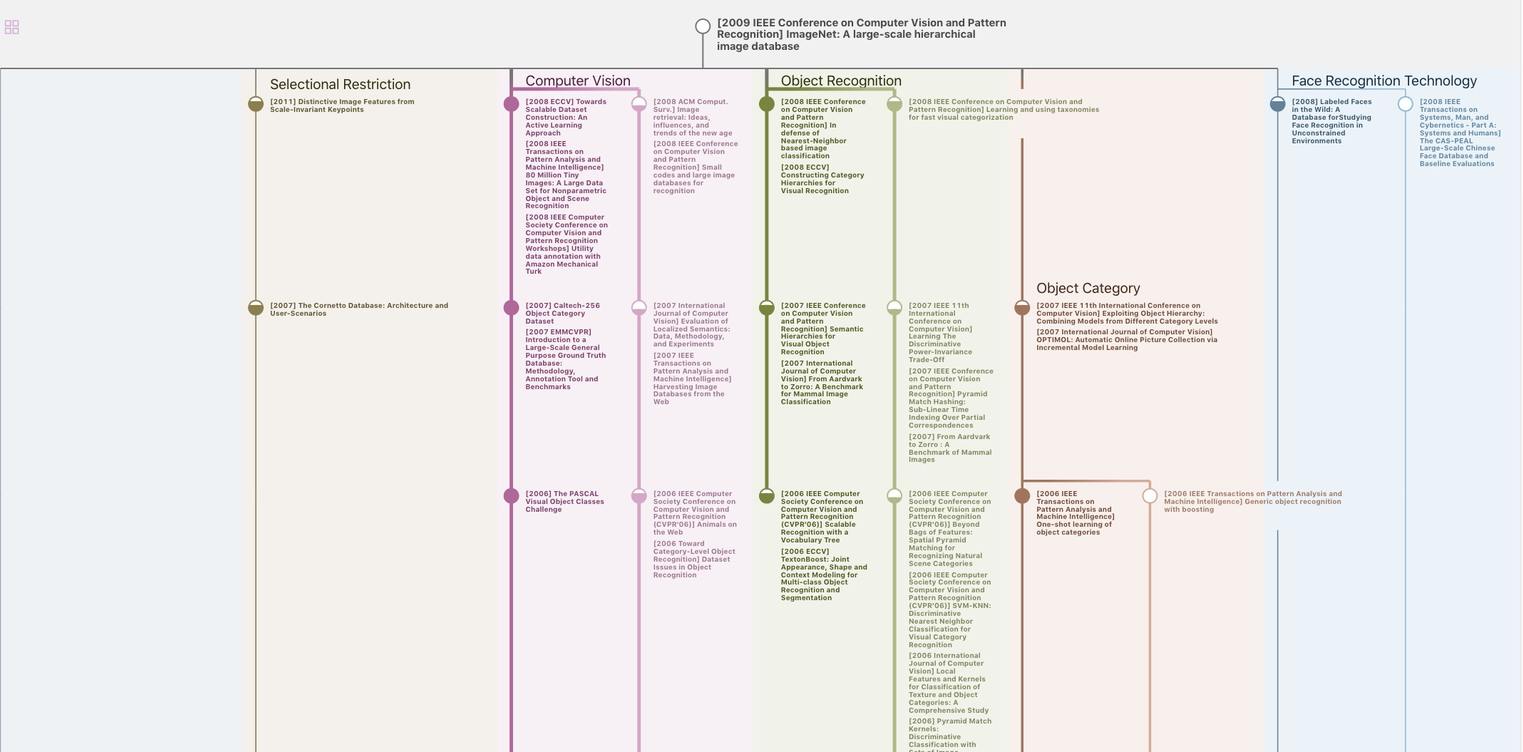
生成溯源树,研究论文发展脉络
Chat Paper
正在生成论文摘要