Adversarial Tracking Control via Strongly Adaptive Online Learning with Memory
semanticscholar(2021)
摘要
We consider tracking adversarial targets in a delayed time-varying linear system with adversarial disturbances and loss functions, which significantly generalizes earlier work. To this end, we develop three techniques that each could be of independent interest. First, we propose a black-box reduction from adversarial tracking control to strongly adaptive online learning with memory. Any solution to the latter translates to a tracking controller that pursues the best action on any time interval. Second, for the resulting online learning problem we develop a novel approach that further adapts to the observed gradients. Third, we propose a new algorithm for unconstrained online linear optimization: for all (unknown) T ∈ N+, the cumulative loss and movement on the time horizon [1 : T ] is upper-bounded by a user-specified constant. Combining these individual techniques, we propose a tracking controller with a sensible performance guarantee even when the adversarial target has a large range of movement.
更多查看译文
AI 理解论文
溯源树
样例
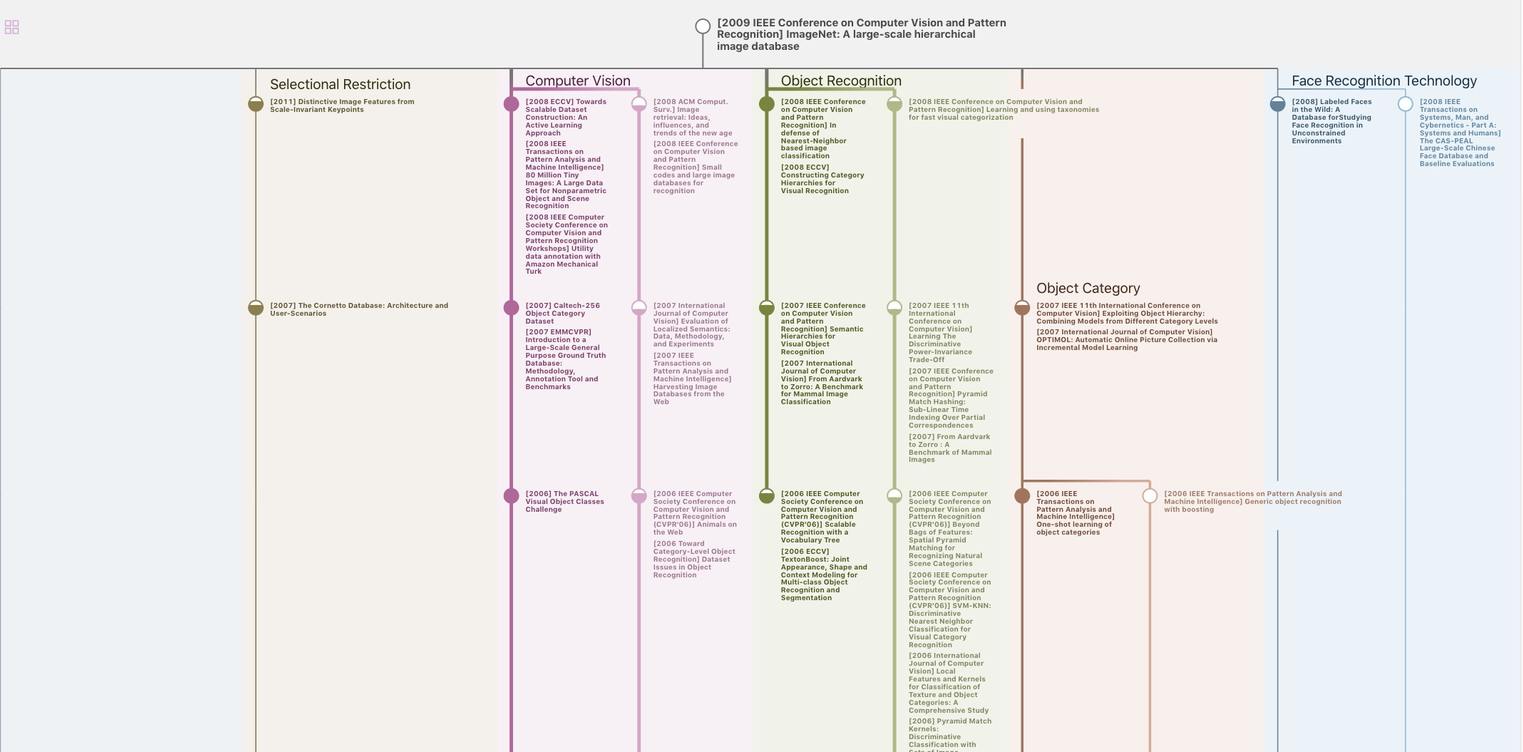
生成溯源树,研究论文发展脉络
Chat Paper
正在生成论文摘要