High-Accuracy Spiking Neural Network for Objective Recognition Based on Proportional Attenuating Neuron
Neural Processing Letters(2021)
摘要
Spiking neural network (SNN) is one of the most successful methods to imitate biological brain behavior and learning potential. The information processing mechanism of SNN combines the concepts of time and space. To address the performance dropping problem during the conversion process from the artificial neural network (ANN) to SNN, this paper proposes a proportional attenuation leaky integrate-and-fire (RA-LIF) neuron model to solve the problem of membrane potential loss caused by leaky integrate-and-fire (LIF) neurons. The accuracy of the SNN network based on RA-LIF neurons on MNIST is 98.76%. This paper also proposes a weight normalization method to help adjust the network spiking rate to reduce the loss. We evaluate and analyze the performance of SNN networks based on LIF, RA-LIF, and AD-LIF. By analyzing the spike firing rate and convergence rate, the effects of spike frequency and neuron threshold on the performance of the network are discussed. So far, the SNN transformed by ANN can only achieve worse or similar performance compared with the original network. For the first time, the performance of the SNN transformed by the proposed RA-LIF neuron model and weight normalization method is better than the original network, which shows the test accuracy of 98.88% on MNIST. The SNN obtained by converting the LeNet network using the above method achieves a test accuracy of 98.91% on MNIST. In conclusion, the RA-LIF neuron model and normalization method proposed in this paper have promising applicability. Moreover, this method has the characteristics of high precision and fast calculation speed, which can provide a reference for the research of the SNN framework.
更多查看译文
关键词
Spiking neural network,RA-LIF neuron,Weight normalization,Spiking rate
AI 理解论文
溯源树
样例
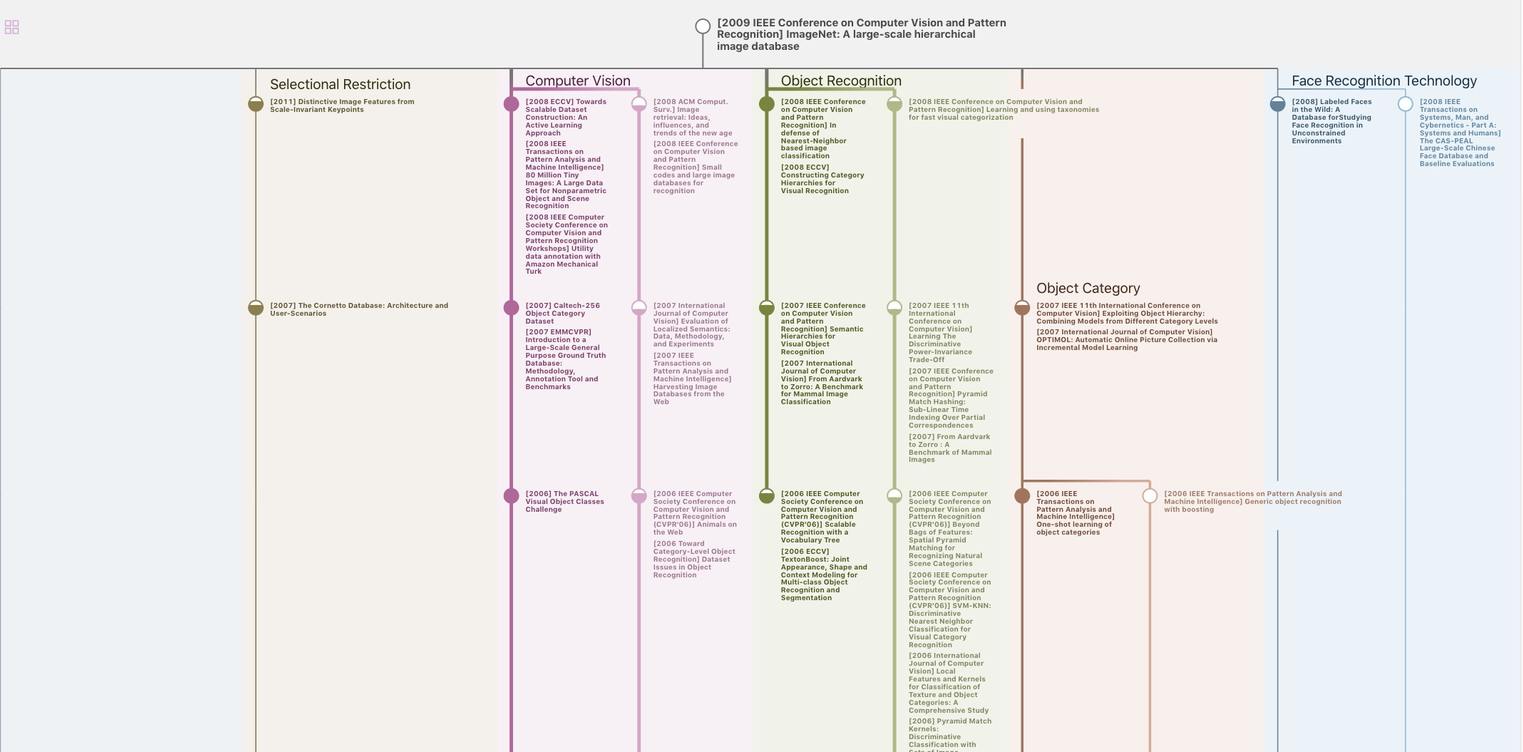
生成溯源树,研究论文发展脉络
Chat Paper
正在生成论文摘要