Automated Feature Selection and Classification for High-Dimensional Biomedical Data
semanticscholar(2021)
摘要
Background: Automated machine learning aims to automate the building of accurate predictive models, including the creation of complex data preprocessing pipelines. Although successful in many fields, they struggle to produce good results on biomedical datasets, especially given the high dimensionality of the data. Result: In this paper, we explore the automation of feature selection in these scenarios. We analyze which feature selection techniques are ideally included in an automated system, determine how to efficiently find the ones that best fit a given dataset, integrate this into an existing AutoML tool (TPOT), and evaluate it on four very different yet representative types of biomedical data: microarray, mass spectrometry, clinical and survey datasets. We focus on feature selection rather than latent feature generation since we often want to explain the model predictions in terms of the intrinsic features of the data. Conclusion: Our experiments show that for none of these datasets we need more than 200 features to accurately explain the output. Additional features did not increase the quality significantly. We also find that the automated machine learning results are significantly improved after adding additional feature selection methods and prior knowledge on how to select and tune them.
更多查看译文
关键词
automated feature selection,feature selection,classification,high-dimensional
AI 理解论文
溯源树
样例
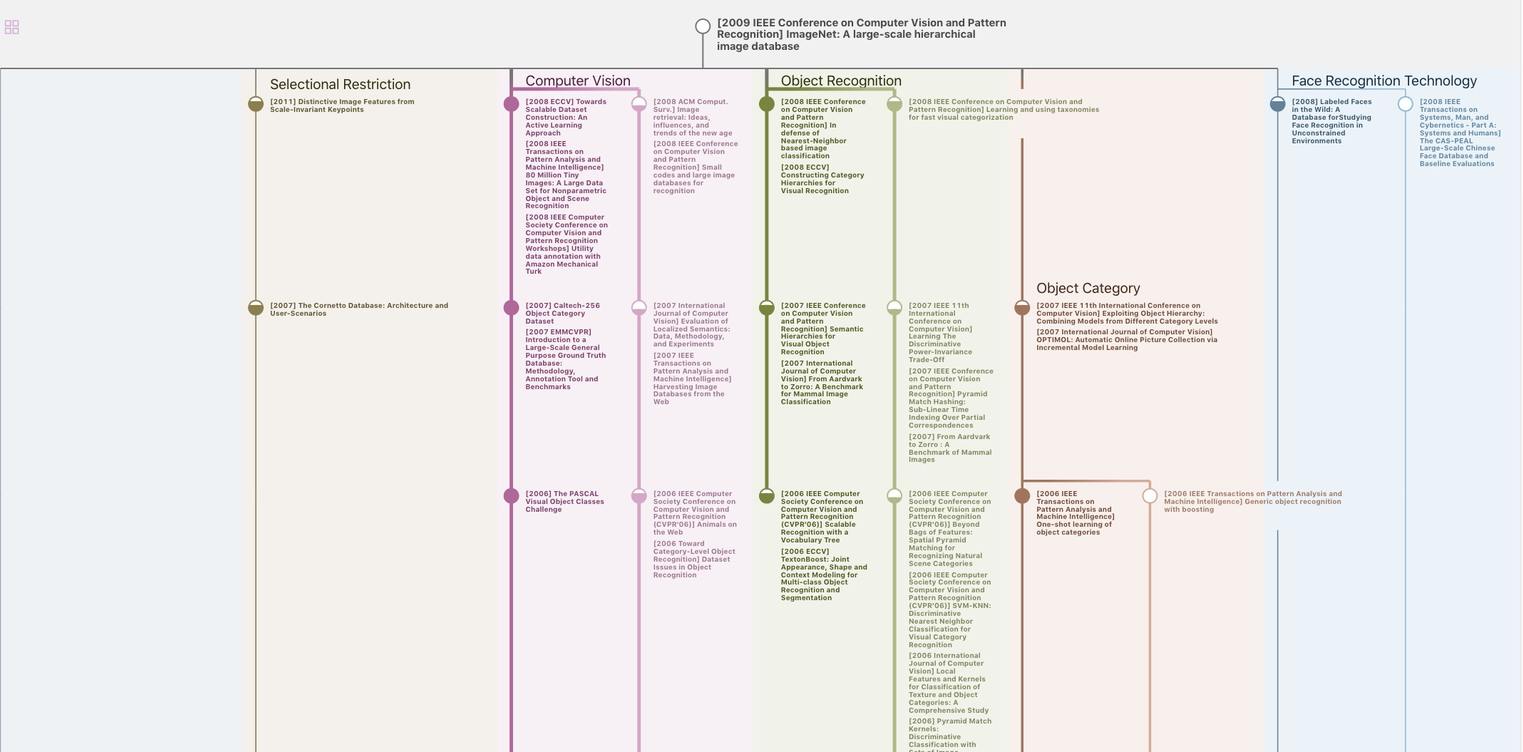
生成溯源树,研究论文发展脉络
Chat Paper
正在生成论文摘要