Efficient Action Recognition via Dynamic Knowledge Propagation -Supplementary Material-
semanticscholar(2021)
摘要
We consider two strategies for frame sampling. First, as used in the main paper, we adapt sampling intervals rs and rt across videos in order to have the same ns and nt for all the videos. For this ‘adaptive’ strategy, we plot for ns/nt = 4 as Adaptive-4 (used in the main paper) and ns/nt = 8 as Adaptive-8. The mAP-GFLOPs curves for our method are shown in Figure S-1. Second, we fix the sampling intervals rs and rt for all the videos, as a result ns and nt vary across videos and are proportional to the video-length. While ns and nt vary over videos, we set the ratio ns/nt as 4 and 8 to plot them as Fixed-4 and Fixed-8, respectively. As Figure S-1 illustrates, given enough sampled frames i.e. beyond 12 GFLOPs, all four plots of the two sampling strategies achieve similar and promising performances. However, Adaptive-4 and Adaptive-8 experience larger performance drop at lower GFLOPs. This is because, in this setup, only a few sampled frames nt = 3 are available per video, which leaves longer videos under-sampled. On the contrary, the fixed sampling interval strategy alleviates this problem by adjusting the number of sampled frames ns and nt according to the video-length, and achieves better mAP over lower computation range. Also, we see that Adaptive-8 and Fixed-8 perform a bit better than Adaptive-4 and Fixed4 at the low GFLOPs setting, respectively. This shows more sampled frames for student is better in the low computation range. Figure S-2 analyzes the impact of the number of sampled frames ns and nt by plotting mAP-GFLOPs curves. Specifically, nt-5 sets nt as 5 and varies ns as {5, 20, 35, 50}. Similarly, ns-20 sets ns as 20 and varies nt as {5, 10, 15, 20}.
更多查看译文
AI 理解论文
溯源树
样例
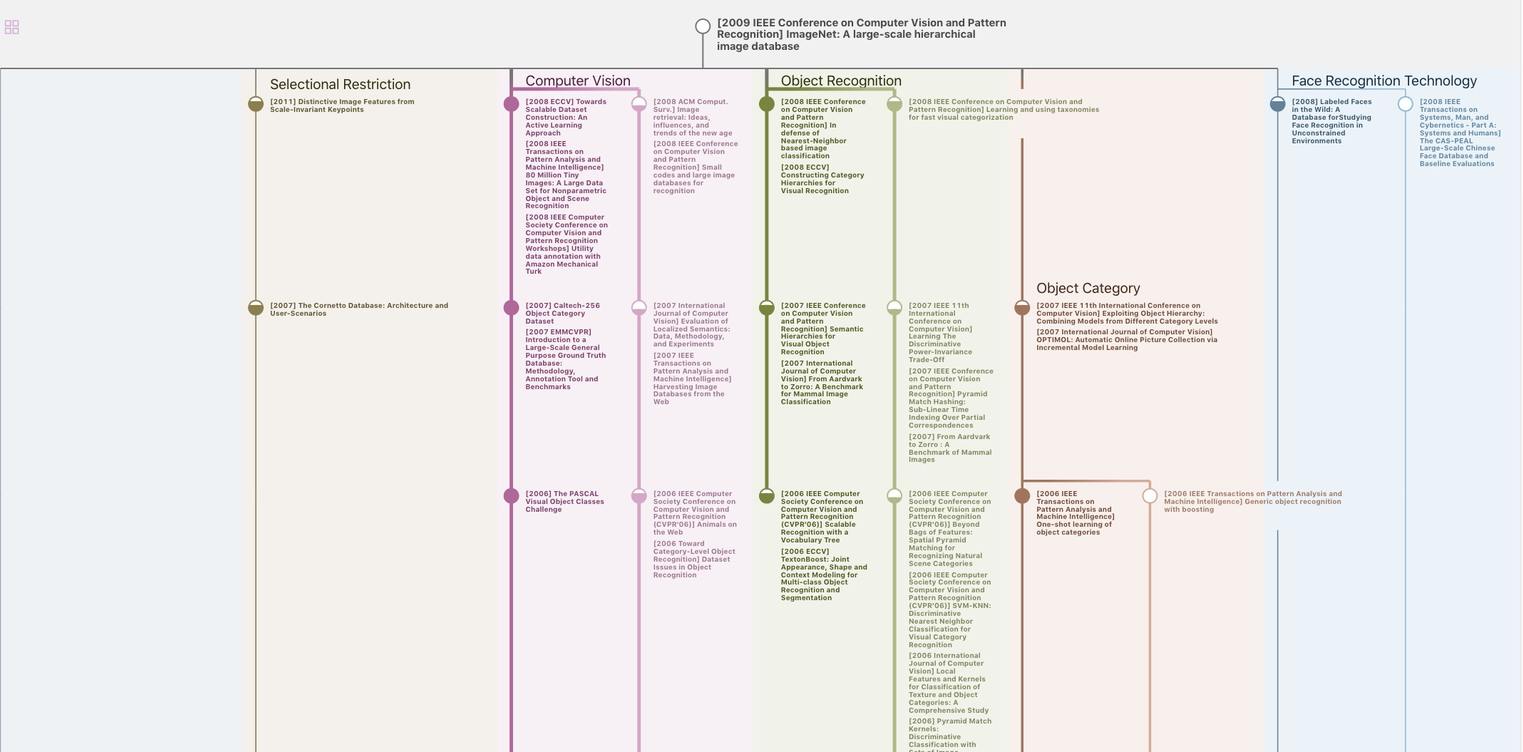
生成溯源树,研究论文发展脉络
Chat Paper
正在生成论文摘要