Quadratic regularization methods with finite-difference gradient approximations
COMPUTATIONAL OPTIMIZATION AND APPLICATIONS(2022)
摘要
This paper presents two quadratic regularization methods with finite-difference gradient approximations for smooth unconstrained optimization problems. One method is based on forward finite-difference gradients, while the other is based on central finite-difference gradients. In both methods, the accuracy of the gradient approximations and the regularization parameter in the quadratic models are jointly adjusted using a nonmonotone acceptance condition for the trial points. When the objective function is bounded from below and has Lipschitz continuous gradient, it is shown that the method based on forward finite-difference gradients needs at most 𝒪( nϵ ^-2) function evaluations to generate a ϵ -approximate stationary point, where n is the problem dimension. Under the additional assumption that the Hessian of the objective is Lipschitz continuous, an evaluation complexity bound of the same order is proved for the method based on central finite-difference gradients. Numerical results are also presented. They confirm the theoretical findings and illustrate the relative efficiency of the proposed methods.
更多查看译文
关键词
Nonconvex Optimization,Derivative-Free Methods,Finite-Differences,Worst-Case Complexity
AI 理解论文
溯源树
样例
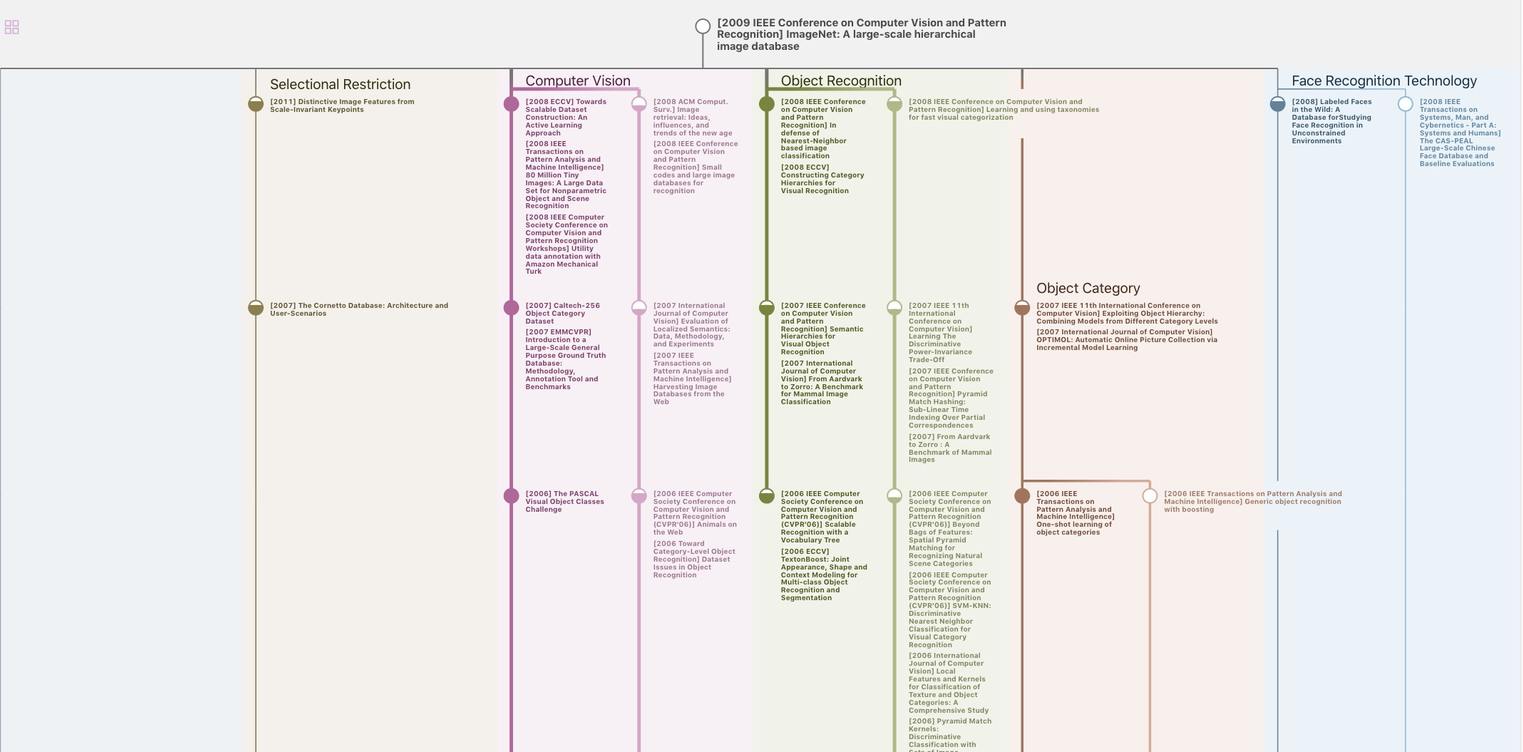
生成溯源树,研究论文发展脉络
Chat Paper
正在生成论文摘要