Sample Selection Guided by Domain and Task for Cross-Domain Targeted Sentiment Analysis
semanticscholar(2021)
摘要
Building supervised targeted sentiment analysis models for a new target domain requires substantial annotation effort since most datasets for this task are domain-specific. Domain adaptation for this task has two dimensions: the nature of targets and the opinion words used to describe sentiment towards the target. We present a data sampling strategy informed by domain differences across these two dimensions with the goal of selecting a small number of examples, thereby minimizing annotation effort. This obtains performance in the 86-100% range compared to the full supervised model using only ∼4-15% of the full training data.
更多查看译文
AI 理解论文
溯源树
样例
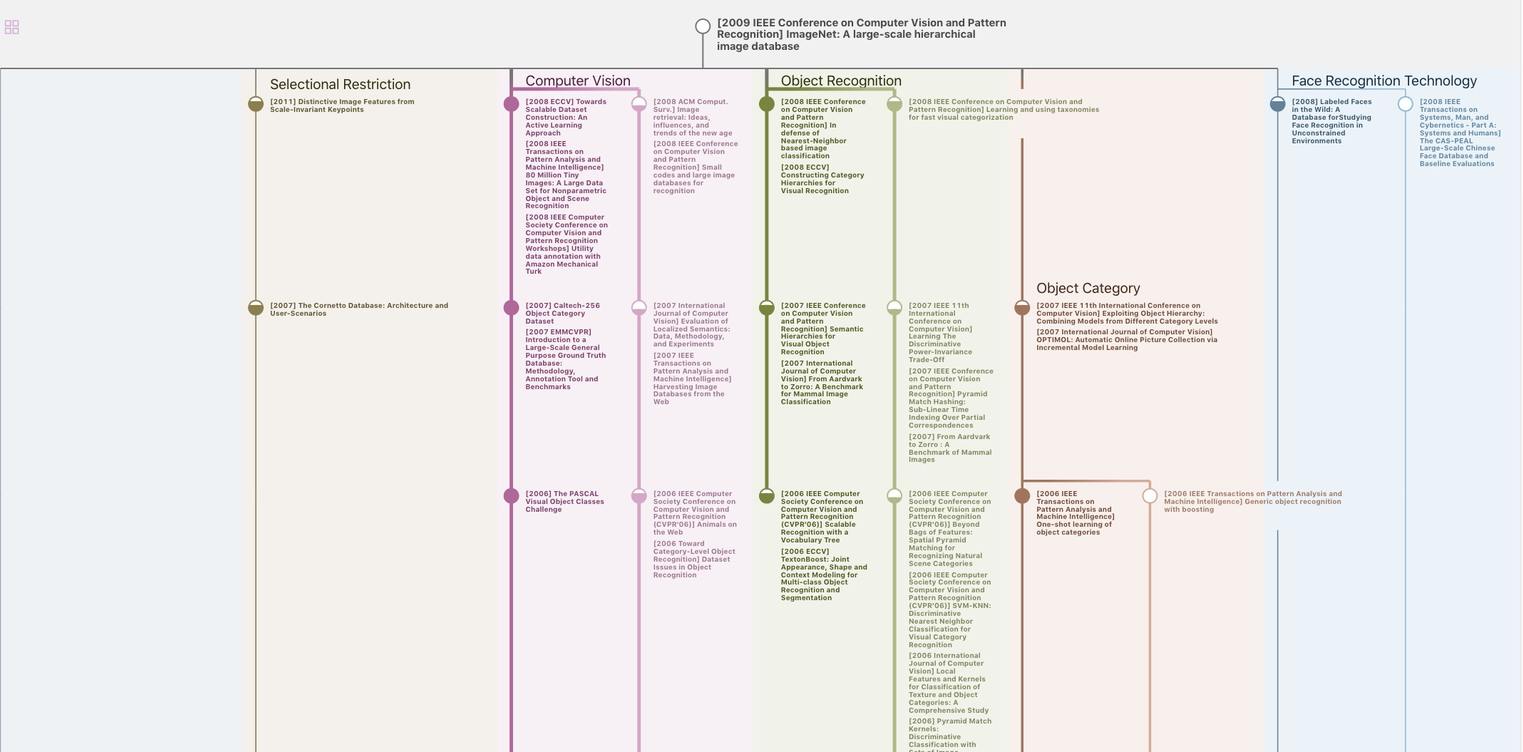
生成溯源树,研究论文发展脉络
Chat Paper
正在生成论文摘要