Connecting the Dots: Explaining Human Reasoning on the Graph A Case Study on Deep Question Generation
semanticscholar(2021)
摘要
Deep Question Generation (DQG) involves generating a complex question given an input passage. This task involves reasoning over multiple sources of information in the passage. To tackle this, Pan et al. (2020) leverages recent advances in graph neural networks (GNN) to represent the passage as a collection of phrases, where each phrase forms a node in a graph. Then, to reason over this graph, information is aggregated via a confined graph structure to combine them to generate meaningful questions. However, what is unclear at this moment is why is the graph important, which structures are important, and if this human imposed structure is even required. This paper looks deeper into answering these 3 main questions via the following approaches: (1) A linguistic heuristic approach to investigate the linguistic structures that are crucial for reasoning in DQG, which can be used as structural priors for building better reasoning models, (2) A GNN learnable approach to investigate the computational structure the model uses and if this aligns with the human one, (3) Generalizing the GNN model used to allow the model to learn its own computational graph structure for the reasoning task.
更多查看译文
AI 理解论文
溯源树
样例
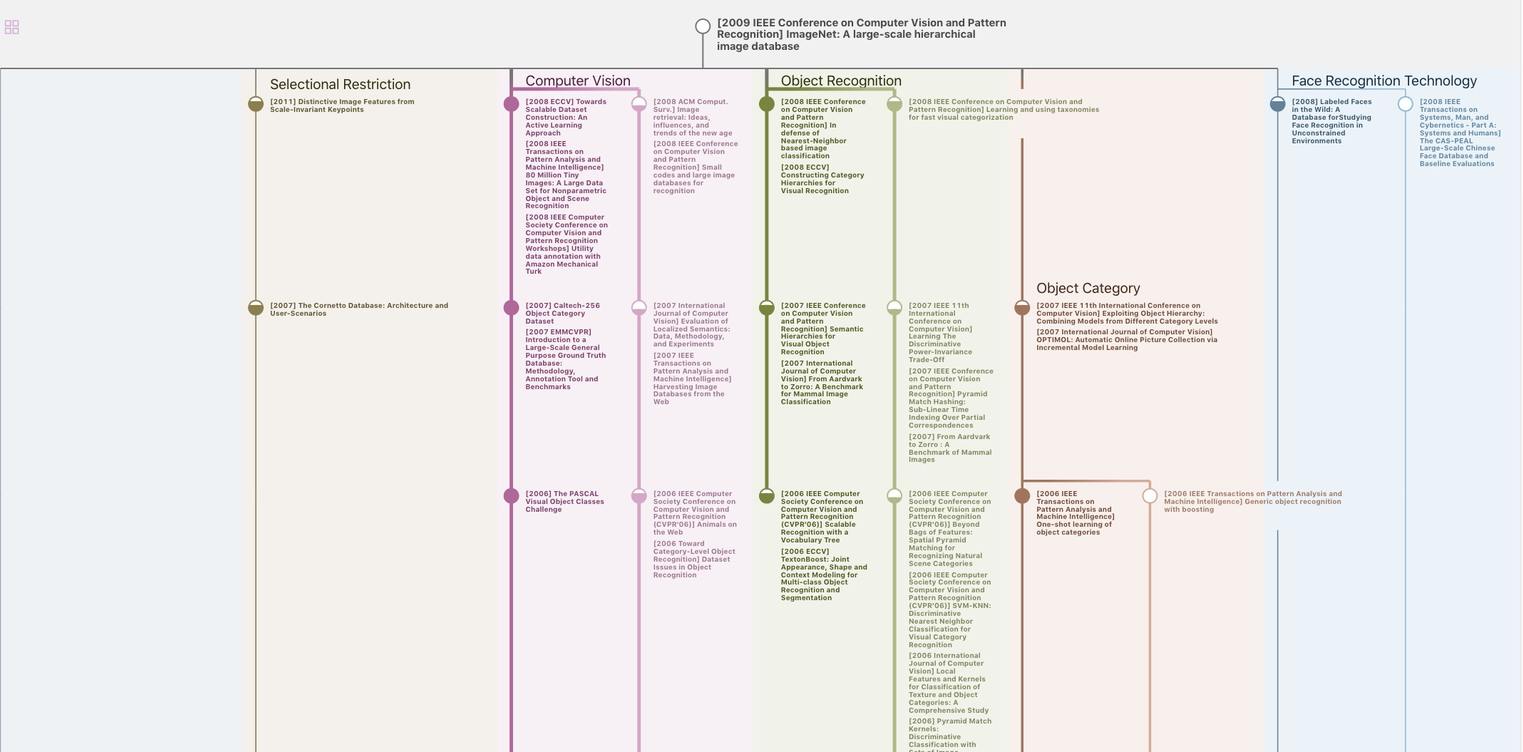
生成溯源树,研究论文发展脉络
Chat Paper
正在生成论文摘要