Face dissimilarity judgements are predicted by representational distance in deep neural networks and principal-component face space
semanticscholar(2021)
摘要
Despite the importance of face perception in human and computer vision, no quantitative model of perceived face dissimilarity exists. 1 We designed an efficient behavioural task to collect dissimilarity and same/different identity judgements for 232 pairs of realistic faces 2 that densely sampled geometric relationships in a face space derived from principal components of 3D shape and texture (Basel Face 3 Model, BFM). In a comparison of 15 models, we found that representational distances in deep neural networks (DNNs) and Euclidean 4 distances within BFM space predicted human judgements best. A face-trained DNN explained unique variance over simpler models 5 and was statistically indistinguishable from the noise ceiling. Sigmoidal transformation of distances improved performance for all 6 models. Identity judgements were better predicted by Euclidean than angular or radial distances in BFM space. DNNs provide 7 the best available image-computable models of perceived face dissimilarity. The success of BFM space suggests that human face 8 perception is attuned to the natural distribution of faces. 9
更多查看译文
AI 理解论文
溯源树
样例
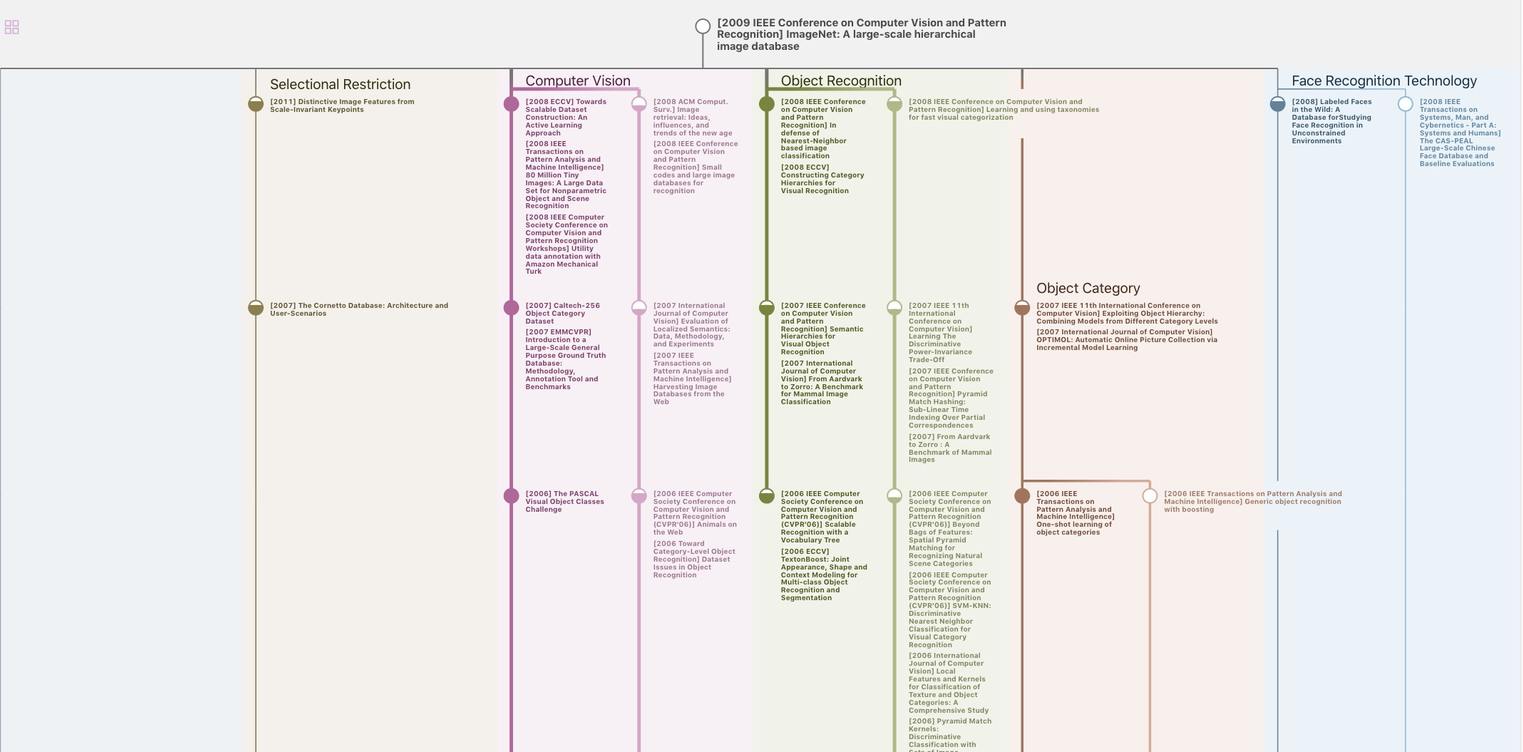
生成溯源树,研究论文发展脉络
Chat Paper
正在生成论文摘要