Analyzing data streams for social scientists
Handbook of Computational Social Science, Volume 2(2021)
摘要
The technological developments of the last decades have created opportunities to efficiently collect data of many individuals over time. While these technologies provide exciting research opportunities, they also provide challenges: datasets collected using these technologies grow increasingly large, or be continuously augmented with new observations. These data streams make the standard computation of well-known estimators inefficient, as computations are repeated each time new data enter. This chapter details online learning, an analysis method that updates parameter estimates instead of re-estimating them to analyze large and/or streaming data. The chapter presents several simple (and exact) examples of the online estimation for independent observations. Additionally, social scientists are often faced with nested data: pupils are nested within schools, or repeated measurements are nested within individuals. Nested data are typically analyzed using multilevel models. Estimating multilevel models, however, can be challenging in data streams: the standard algorithms used to fit these models repeatedly revisit all data points, which becomes infeasible in a data stream context. We present a solution to this problem by introducing the Streaming Expectation Maximization Approximation (SEMA) algorithm for fitting multilevel models online. We end this chapter with a discussion of the methodological challenges that remain.
更多查看译文
关键词
data streams,analyzing
AI 理解论文
溯源树
样例
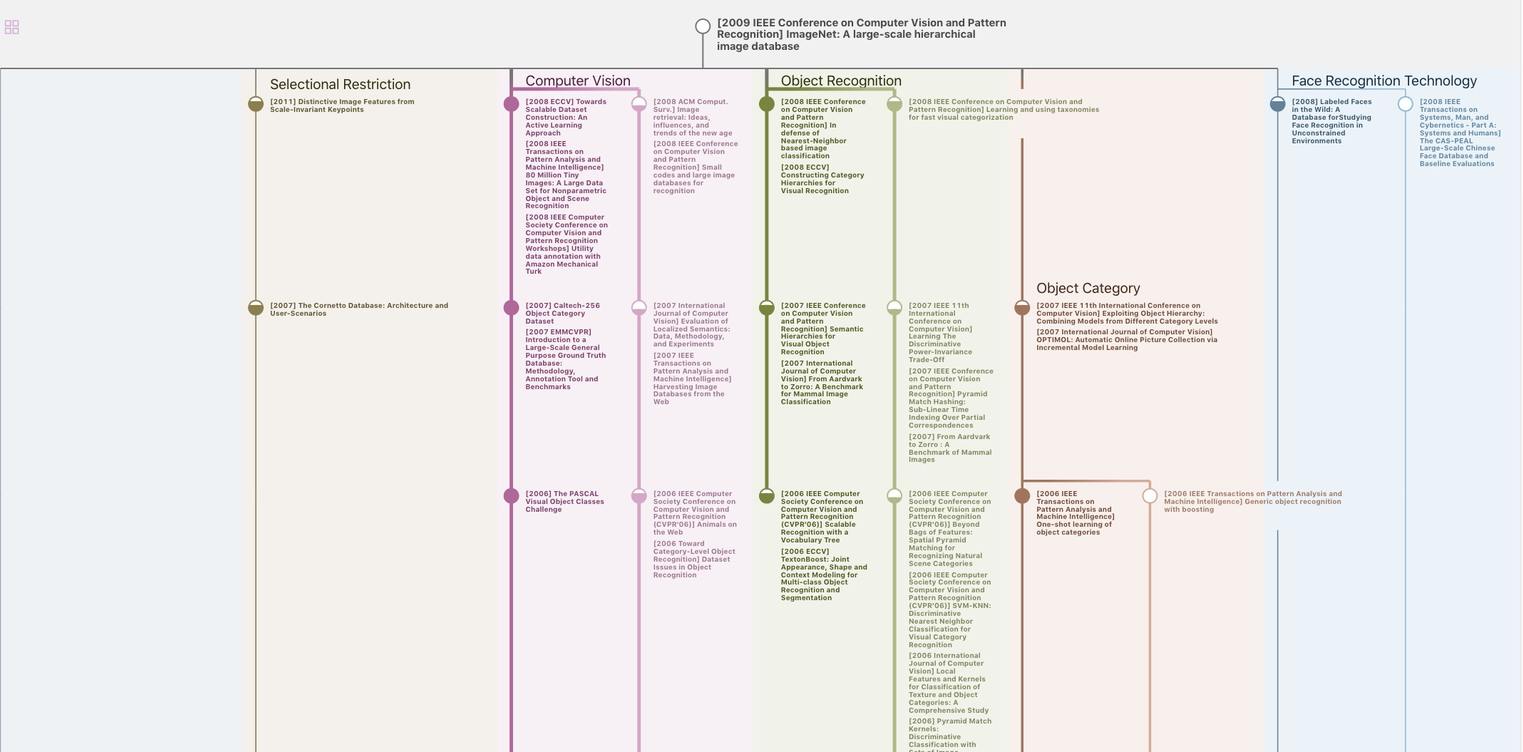
生成溯源树,研究论文发展脉络
Chat Paper
正在生成论文摘要