Multiple Moment Matching Inference: A Flexible Approximate Inference Algorithm
semanticscholar(2021)
摘要
Choosing the right approximation distribution family for variational inference (VI) requires case-by-case design to strike a good balance between representation power and computational cost. Particle-based variational inference (PVI) methods, such as Stein Variational Gradient Descent (SVGD), are proposed as more flexible alternatives to VI due to their non-parametric nature. However, the demand for more flexible inference algorithms never stops. In this paper, we present Multiple Moment Matching Inference (MMMI), a PVI algorithm based on the idea of moment matching. We argue that MMMI allows for more flexible priors and likelihood functions than other PVI algorithms, which further improves its representation power and extends potential application domains. We demonstrate MMMI’s competitive predictive performance for Bayesian neural networks on several real-world datasets.
更多查看译文
AI 理解论文
溯源树
样例
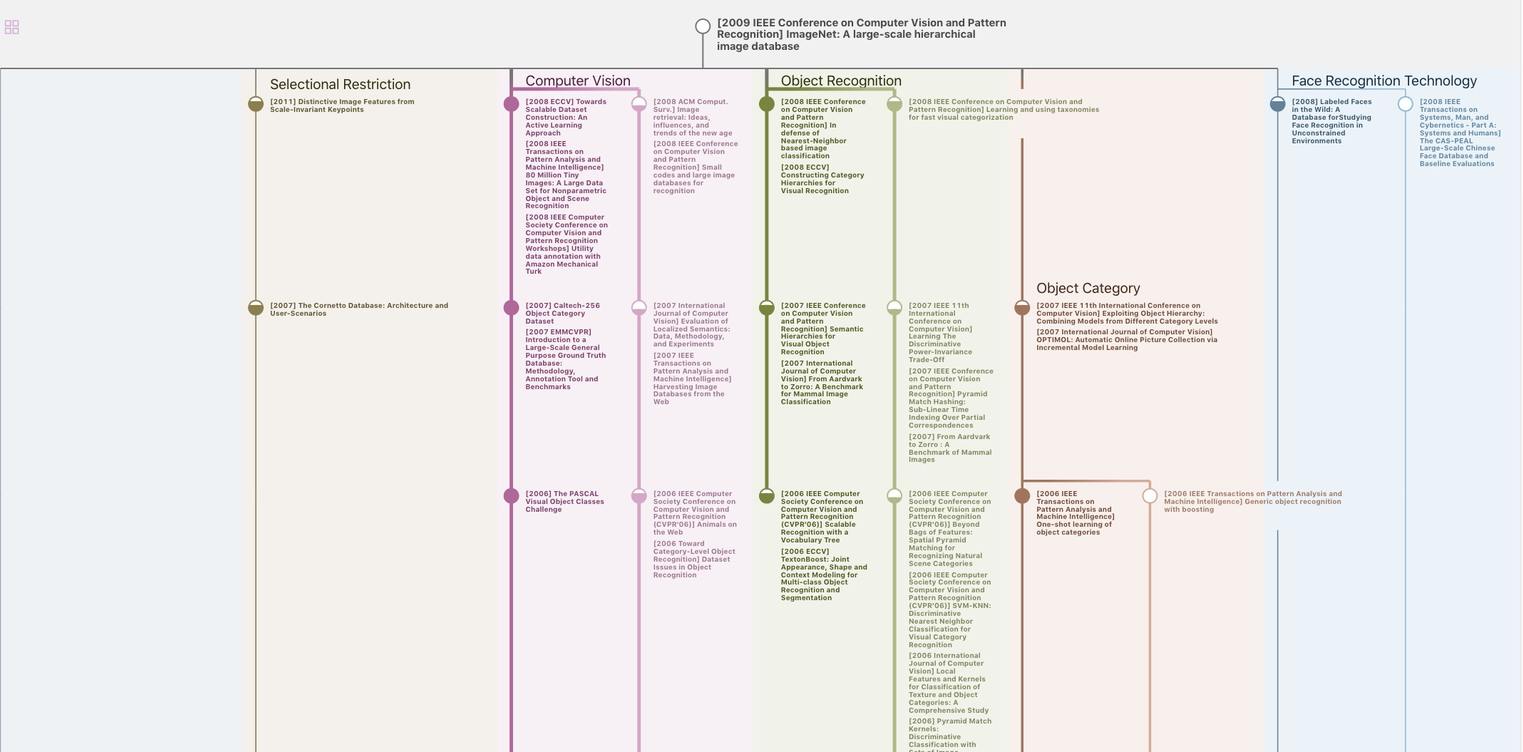
生成溯源树,研究论文发展脉络
Chat Paper
正在生成论文摘要