WiFi mobility models for COVID-19 enable less burdensome and more localized interventions for university campuses
medRxiv(2021)
摘要
Infectious diseases, like COVID-19, pose serious challenges to university campuses, and they typically adopt closure as a non-pharmaceutical intervention to control spread early and ensure a gradual return to normalcy. These policies, like remote instruction (SQ), reduce potential contact but also have broad side-effects on campus by hampering local economy, students learning outcomes, and community wellbeing. In this paper, we demonstrate that university policymakers can mitigate these tradeoffs by leveraging anonymized data from their WiFi infrastructure to learn community mobility (WF) and in turn explore more granular policies like localized closures (LC). WF can construct contact networks that capture behavior in a variety of spaces, highlighting new potential transmission pathways and temporal variation in contact behavior. Additionally, WF enables us to design LC policies that close super-spreader locations on campus. On simulating disease spread with contact networks from WF, we find that LC maintains the same reduction in cumulative infections as SQ while showing greater reduction in peak infections and internal transmission. Moreover, LC reduces campus burden by closing fewer locations, forcing fewer students into completely online schedules, and requiring no additional isolation. WF can empower universities to conceive and assess a variety of closure policies to prevent future outbreaks.
更多查看译文
关键词
Mobility model,Internet privacy,Variety (cybernetics),Intervention (law),Control (management),Psychological intervention,Computer science,Contact behavior,Coronavirus disease 2019 (COVID-19),Local economy
AI 理解论文
溯源树
样例
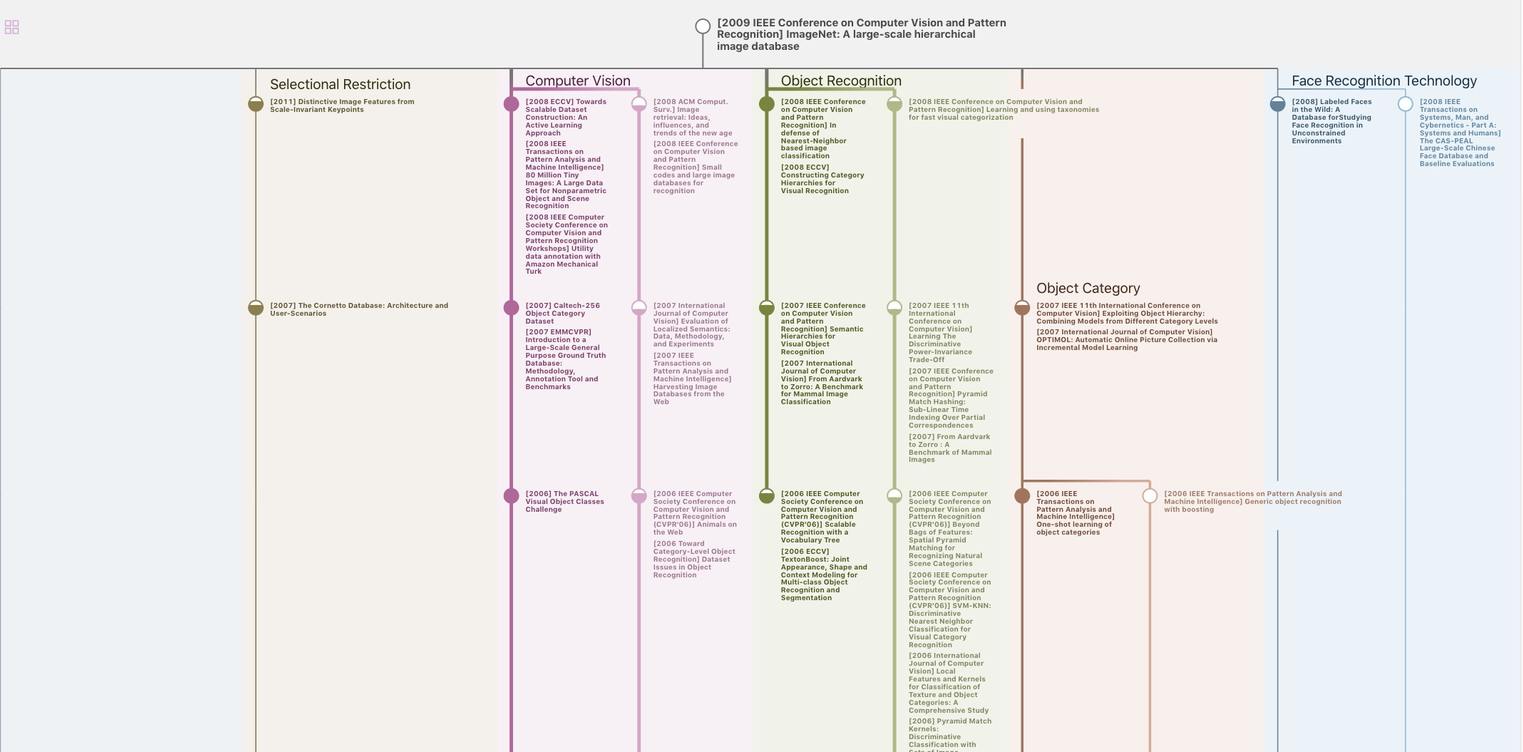
生成溯源树,研究论文发展脉络
Chat Paper
正在生成论文摘要