Online, Opt-in Surveys: Fast and Cheap, but are they Accurate?
semanticscholar(2021)
摘要
It is increasingly common for government and industry organizations to conduct online, optin surveys, in part because they are typically fast, inexpensive, and convenient. Online polls, however, attract a non-representative set of respondents, and so it is unclear whether results from such surveys generalize to the broader population. These non-representative surveys stand in contrast to probability-based sampling methods, such as random-digit dialing (RDD) of phones, which are a staple of traditional survey research. Here we investigate the accuracy of non-representative data by administering an online, fully opt-in poll of social and political attitudes. Our survey consisted of 49 multiple-choice attitudinal questions drawn from the probability-based, in-person General Social Survey (GSS) and select RDD phone surveys by the Pew Research Center. To correct for the inherent biases of non-representative data, we statistically adjust estimates via model-based poststratification, a classic statistical tool but one that is only infrequently used for bias correction. Our online survey took less than onetwentieth the time and money of traditional RDD polling, and less than one-hundredth the time and money of GSS polling. After statistical correction, we find the median absolute difference between the non-probability-based online survey and the probability-based GSS and Pew studies is 7 percentage points. This difference is considerably larger than if the surveys were all perfect simple random samples drawn from the same population; the gap, however, is comparable to that between the GSS and Pew estimates themselves. For most questions, the differences are small enough that many stakeholders would reach the same qualitative conclusion from either survey design. Our results suggest that with proper statistical adjustment, online, non-representative surveys are a valuable tool for practitioners in varied domains.
更多查看译文
AI 理解论文
溯源树
样例
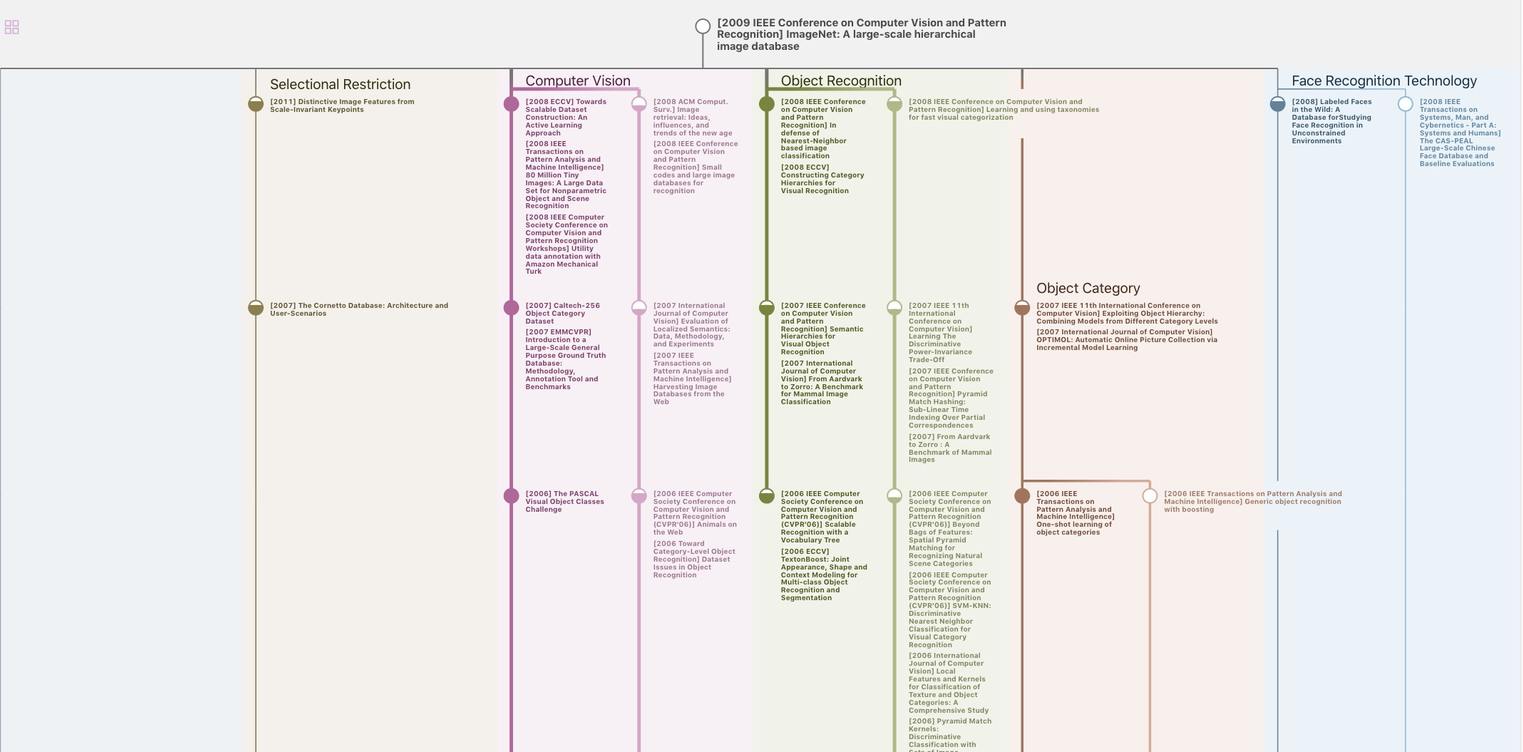
生成溯源树,研究论文发展脉络
Chat Paper
正在生成论文摘要