Validity and Feasibility of the Monitoring and Modeling Family Eating Dynamics (M2FED) System to Automatically Detect In-Field Family Eating Behavior (Preprint)
semanticscholar(2021)
摘要
BACKGROUND
The field of dietary assessment has a long history marked by both controversies and advances. Emerging technologies have been offered as a potential solution to address the limitations of self-report dietary assessment methods. The Monitoring and Modeling Family Eating Dynamics (M2FED) study utilizes wrist-worn smartwatches to automatically detect real-time eating activity in the field. Ecological momentary assessment (EMA) methodology is also employed to confirm whether eating occurred (i.e., ground-truth) and to measure other pertinent contextual information.
OBJECTIVE
The purpose of this paper is to report on participant compliance (feasibility) to the M2FED study’s two distinct EMA protocols (hourly time-triggered assessments and eating event-triggered assessments) and on the performance (validity) of the smartwatch algorithm in automatically detecting eating events in a family-based study.
METHODS
20 families (58 participants) participated in the two-week, observational, M2FED study. All participants were instructed to wear a smartwatch on their dominant hand and to respond to time-triggered and eating event-triggered mobile questionnaires via EMA while at home. EMA data were processed with a “participation algorithm” that identified time intervals in which participants were likely both at home and actively participating in the study. Compliance to EMA was calculated (i) overall, (ii) for hourly time-triggered mobile questionnaires, and (iii) for eating event-triggered mobile questionnaires. Predictors of compliance were determined with a logistic regression model. The number of true positive and false positive eating events was calculated, as well as the precision of the smartwatch algorithm. The Mann Whitney U Test, the Kruskal-Wallis Test, and Spearman’s Rank Correlation were used to determine whether there were differences in the detection of eating events by participant age, gender, family role, and height.
RESULTS
The overall compliance rate across the 20 deployments was 89.3% for all EMAs, 89.7% for time-triggered EMAs, and 85.7% for eating event-triggered EMAs. Time of day and whether other family members had also answered an EMA were significant predictors of compliance to time-triggered EMAs. Weekend status and deployment day were significant predictors of compliance to eating event-triggered EMAs. Approximately three-fourths (76.5%) of detected eating events were confirmed as true positives, and precision was 0.765. The proportion of correctly detected eating events did not significantly differ by participant age, gender, family role, nor height.
CONCLUSIONS
This paper demonstrates that EMA is a feasible tool to collect ground-truth eating activity and thus evaluate the performance of wearable sensors in the field. The combination of a wrist-worn smartwatch to automatically detect eating and a mobile or wearable device to capture ground-truth eating activity offers key advantages for the user (participant) and makes mHealth technologies more accessible to non-engineering behavioral researchers.
更多查看译文
AI 理解论文
溯源树
样例
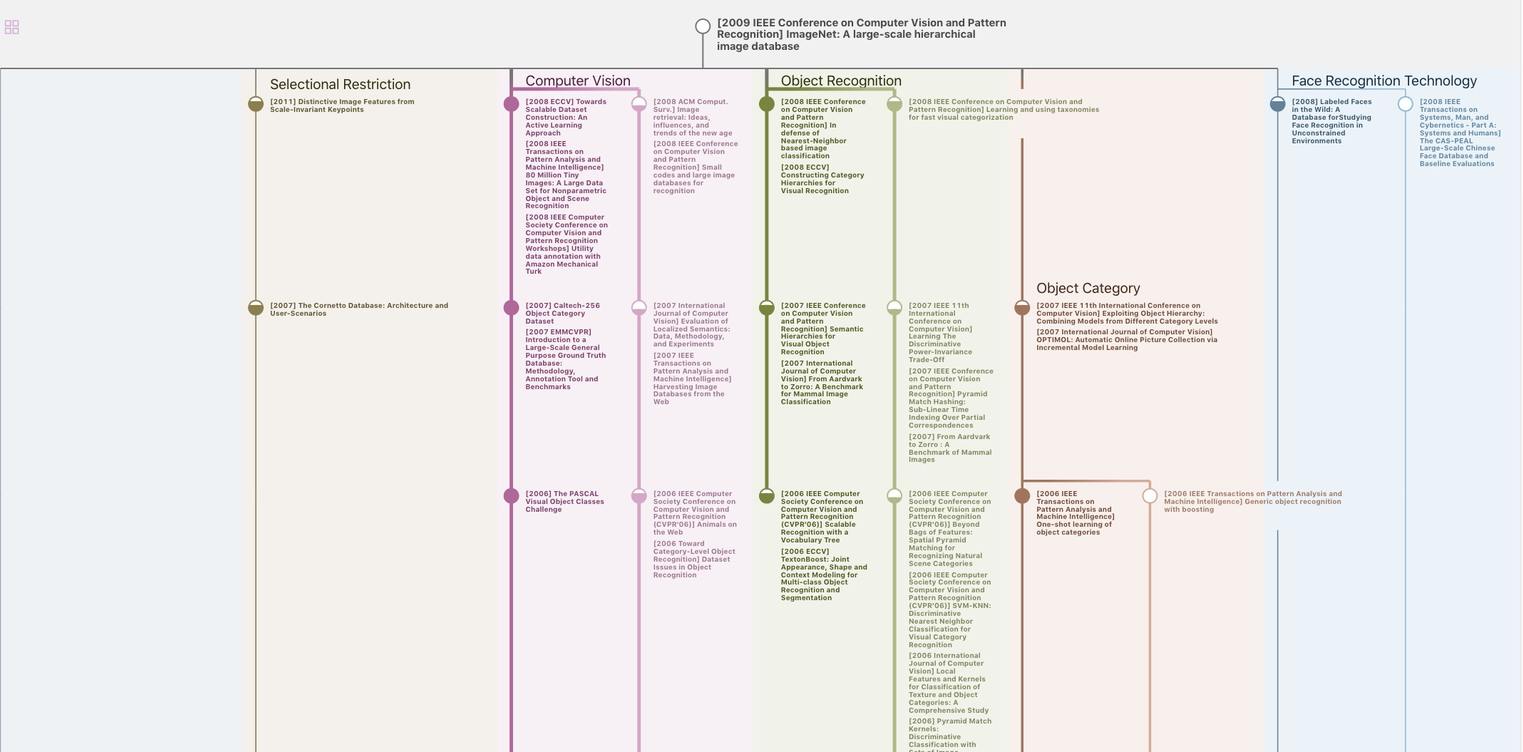
生成溯源树,研究论文发展脉络
Chat Paper
正在生成论文摘要