Personalized Diversity in MDP-Based Recommendation Engines
semanticscholar(2019)
摘要
Diversity is an important aspect of recommender systems. Recommendations that are diverse encourage users to explore new interests and avoid boredom over time. However, common recommender systems designed to fit users’ dominant interests tend to make narrow recommendations and are likely to form filter bubbles. Furthermore, uniformly enforcing diversity among all users is likely to harm the utility of recommendations. Therefore, we adopt a dynamic recommender model that adjusts individual recommendation diversity according to their tolerance to diversity. We pose the recommendation problem as a Markov Decision Process with modified reward function, which favors recommendations that relevant and satisfies users’ preferred level of diversity. We evaluate our method against a diversity-driven approach and a relevancy-driven approach in a synthetic setting. We compare the relevancy and diversity of recommendations using standard evaluation metrics. Using our quantitative results, we will provide qualitative results on the trade-off between relevancy and diversity and on the prevention of preference polarization.
更多查看译文
AI 理解论文
溯源树
样例
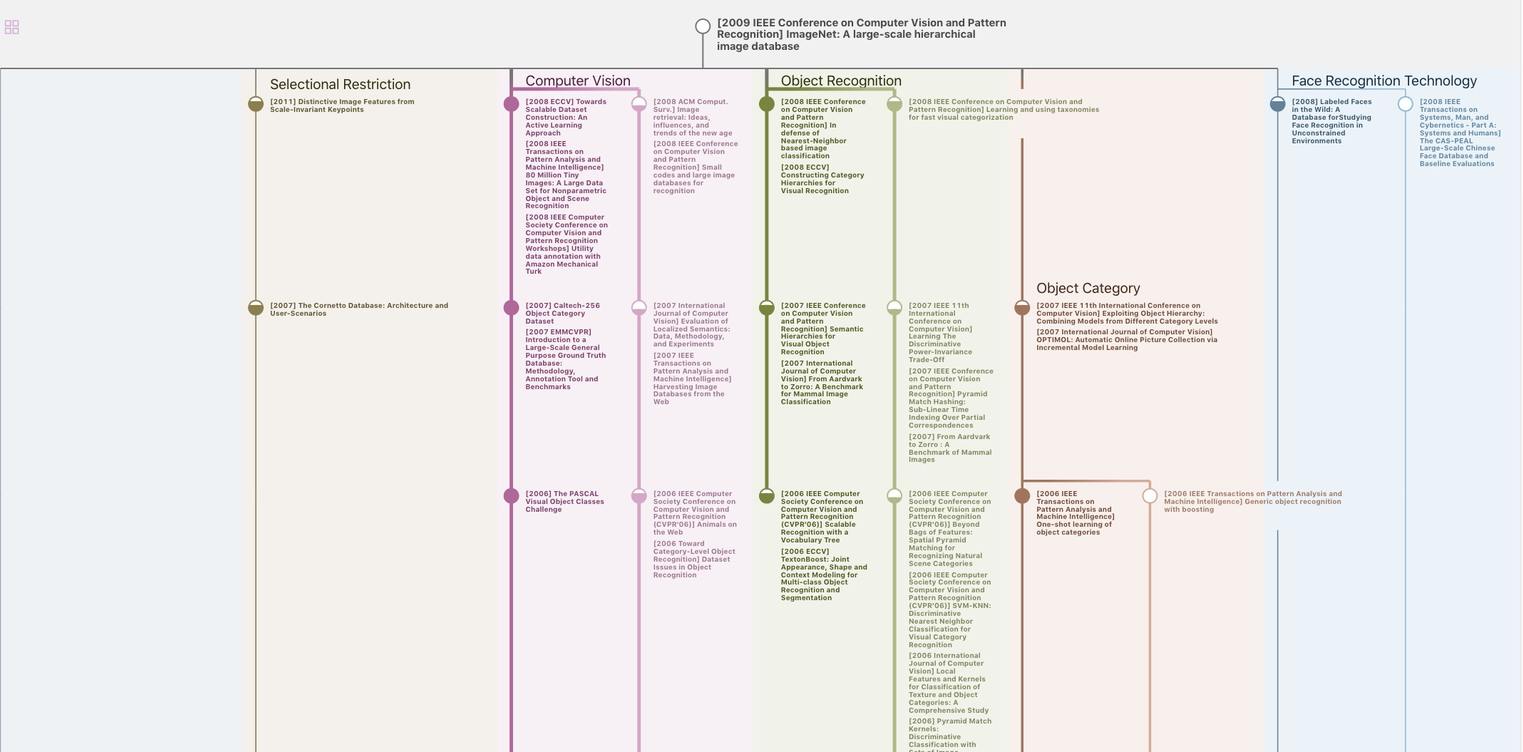
生成溯源树,研究论文发展脉络
Chat Paper
正在生成论文摘要