Exploring instance correlations with local discriminant model for multi-label feature selection
Applied Intelligence(2021)
摘要
Multi-Label Learning (MLL) is a classification task in which each instance may be associated with two or more class labels simultaneously. With the arrival of information explosion era, MLL is confronted with the curse of high-dimensional data. Feature selection is an effective technique that has been used to reduce dimensionality. Previous Multi-Label Feature Selection (MLFS) approaches only lay emphasis on the fitting relations between the feature space and the corresponding label space while neglecting the instance correlations. To remedy the deficiency, we present a novel MLFS method by Exploring Instance Correlations with Local Discriminant model (EICLD). More specifically, we first construct a local set for each instance, and use a local discriminant model for each local set to explore instance correlations. Then, we further integrate the local models of all instances to guarantee the global instance correlations. Finally, l 2,1 -norm is introduced into loss function and the regularization of feature weight matrix respectively to facilitate feature selection process. An optimization algorithm is designed for handling the proposed objective function. The paper is compared with seven representative approaches on twelve data sets. The results validate the superiority of the proposed approach.
更多查看译文
关键词
Multi-label learning, Feature selection, Local discriminant model, Optimization function
AI 理解论文
溯源树
样例
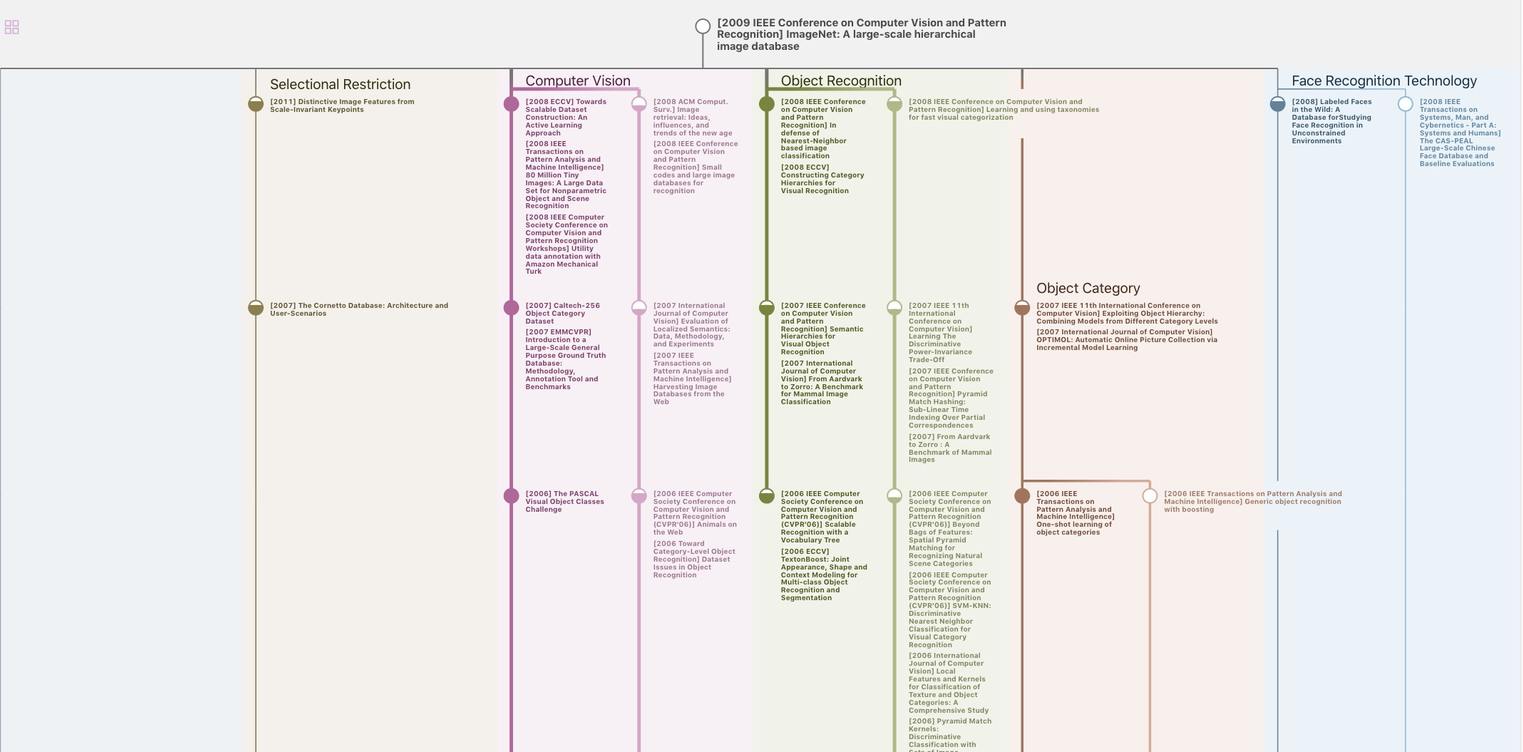
生成溯源树,研究论文发展脉络
Chat Paper
正在生成论文摘要