Look at the Loss: Towards Robust Detection of False Positive Feature Interactions Learned by Neural Networks on Genomic Data
semanticscholar(2020)
摘要
Gene expression is modulated by cooperative binding of regulatory proteins called transcription factors (TFs) to DNA sequences. Recent work has demonstrated that neural networks show promise at identifying candidate pairs of TFs that have super-additive or sub-additive interaction effects, but the reliability of these predicted interactions remains unclear. Here, we design a simulated dataset to study the propensity of neural networks to learn false positive interactions. We find that feature interaction scores obtained from popular neural network architectures trained with multiple random initializations are consistently prone to identifying false positive interactions with large predicted interaction effects, and that previously-proposed null distributions based on the effect size of the interaction scores do not adequately control for false positives even if combining results across different model architectures. Instead, we find that the contribution of an interaction effect to the prediction loss rather than the magnitude of the interaction itself is a far more robust indicator of whether an interaction is likely to be real. Coupled with checking for consistency across different model architectures, our proposed tests based on loss improvement can reliably distinguish between positive and negative controls in our simulated data. To our knowledge, these are the first proposed statistical tests for detecting false positive interactions that leverage improvement in prediction loss on held-out data. We also perform analysis to shed insight on why models may learn large interaction effects in the absence of a groundtruth interaction. Code + trained models to replicate results available at https://github.com/ kundajelab/feature_interactions.
更多查看译文
AI 理解论文
溯源树
样例
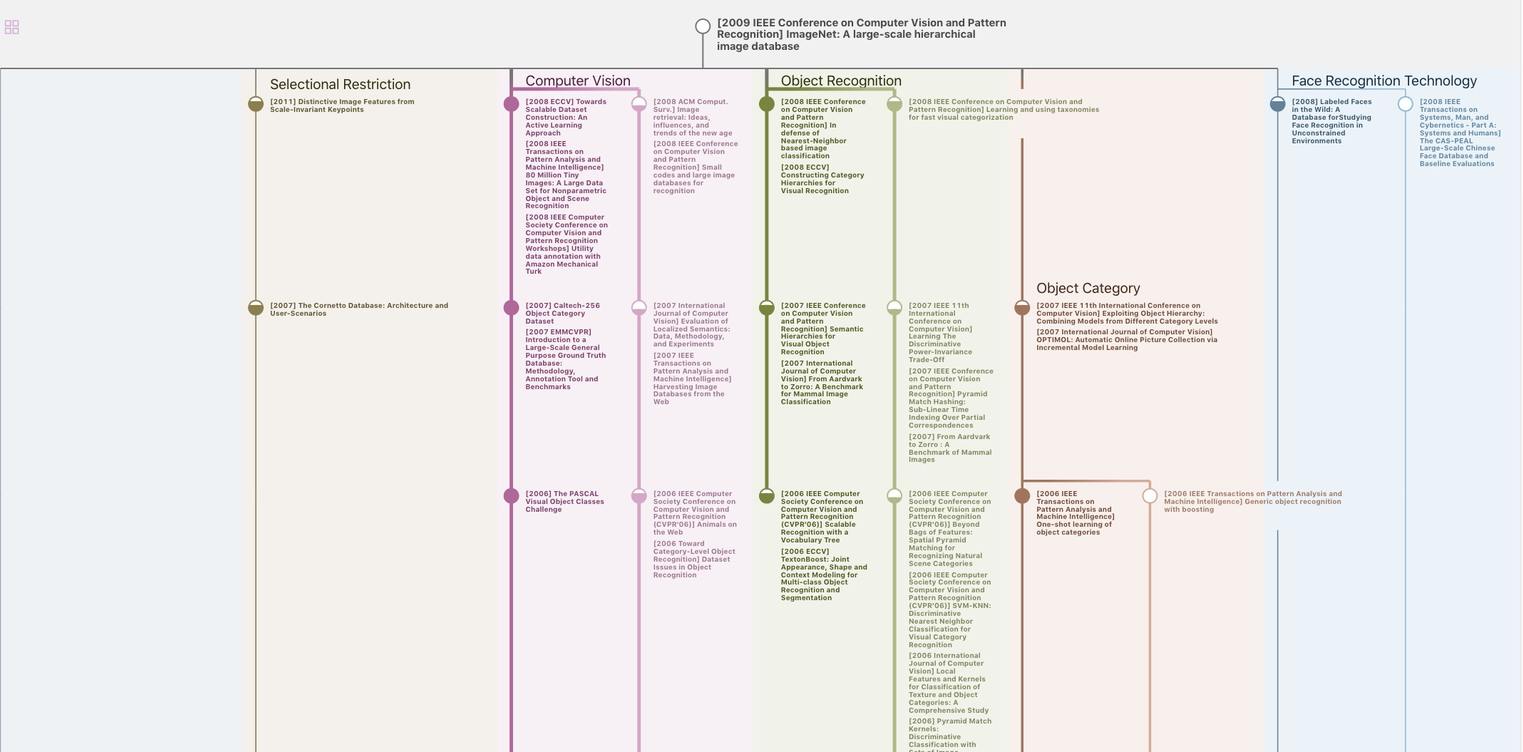
生成溯源树,研究论文发展脉络
Chat Paper
正在生成论文摘要