Cost Inference in Smooth Dynamic Games from Noise-Corrupted Partial State Observations
semanticscholar(2021)
摘要
Robots and autonomous systems must interact with one another and their environment to provide high-quality services to their users. Dynamic game theory provides an expressive theoretical framework for modeling scenarios involving multiple agents with differing objectives interacting over time. A core challenge when formulating a dynamic game is designing objectives for each agent that capture desired behavior. In this paper, we propose a method for inferring parametric objective models of multiple agents based on observed interactions. Our inverse game solver jointly optimizes player objectives and continuous state estimates by coupling them through Nash equilibrium constraints. Hence, our method is able to directly maximize the observation likelihood rather than other non-probabilistic surrogate criteria. We demonstrate our method in a simulated highway driving scenario. Results show that it reliably estimates player objectives from a short sequence of noise-corrupted, partial state observations. Furthermore, using the estimated objectives, our method makes accurate predictions of each player’s trajectory.
更多查看译文
AI 理解论文
溯源树
样例
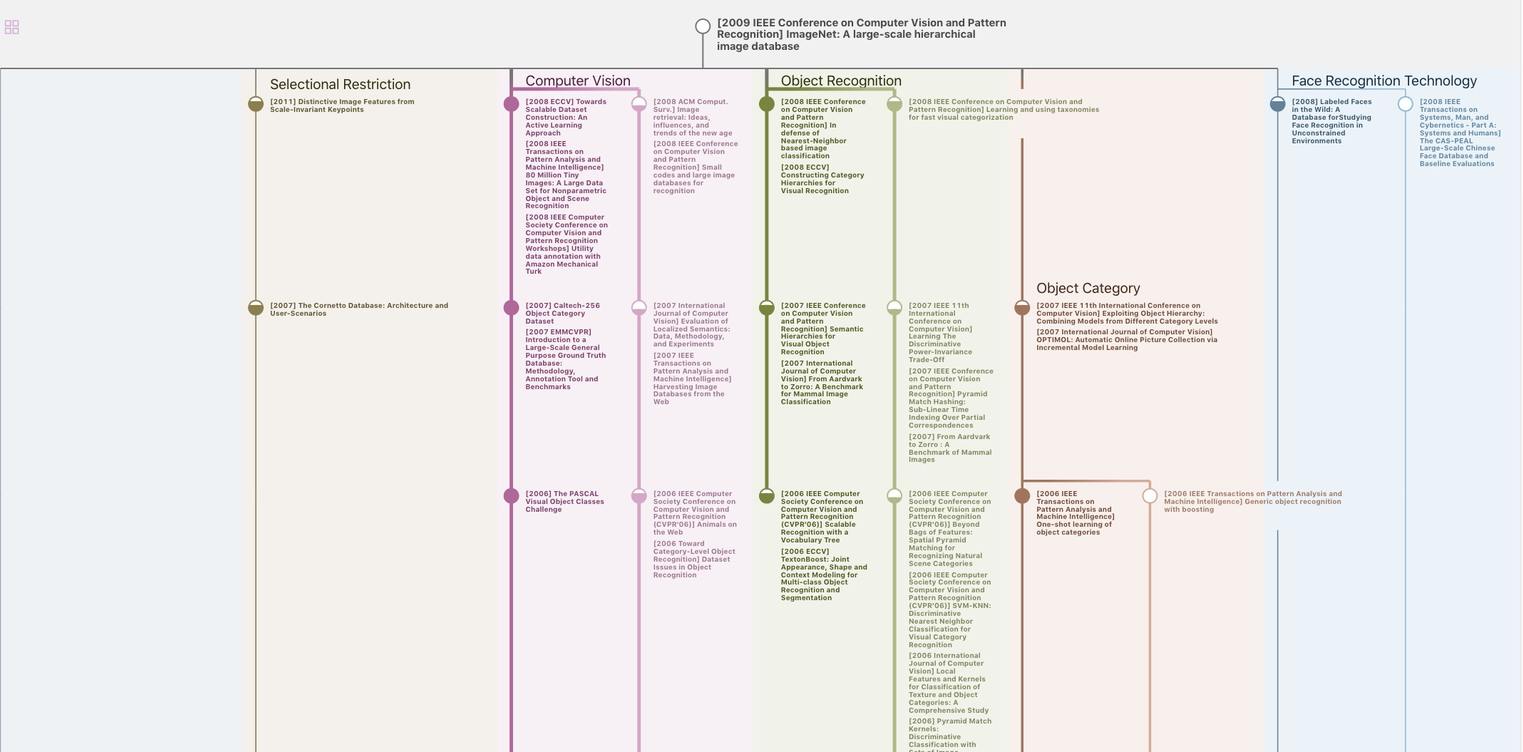
生成溯源树,研究论文发展脉络
Chat Paper
正在生成论文摘要