A Real-Time Steel Surface Defect Detection Approach With High Accuracy
IEEE TRANSACTIONS ON INSTRUMENTATION AND MEASUREMENT(2022)
摘要
Surface defect inspection is a key step to ensure the quality of the hot rolled steel surface. However, current advanced detection (DET) methods have high precision but low detection speed, which hinders the application of the detector in actual production. In this work, a real-time detection network (RDN) focusing on both speed and accuracy is proposed to solve the problem of steel surface defect detection. RDN takes ResNet-dcn, a modular encoding, and decoding network with light weights, as the basic convolutional architecture whose backbone is pretrained on ImageNet. To improve the detection accuracy, a skip layer connection module (SCM) and a pyramid feature fusion module (PFM) are involved into RDN. On the standard dataset NEU-DET, the proposed method can achieve the state-of-the-art recognition speed of 64 frames per second (FPS) and the mean average precision of 80.0% on a single GPU, which fully meets the requirements of the detection accuracy and speed in the actual production line.
更多查看译文
关键词
Feature extraction, Steel, Convolution, Production, Strips, Real-time systems, Object detection, Detection (DET) speed and accuracy, hot rolled steel surface, pyramid feature fusion module (PFM), skip layer connection module (SCM), surface defect detection
AI 理解论文
溯源树
样例
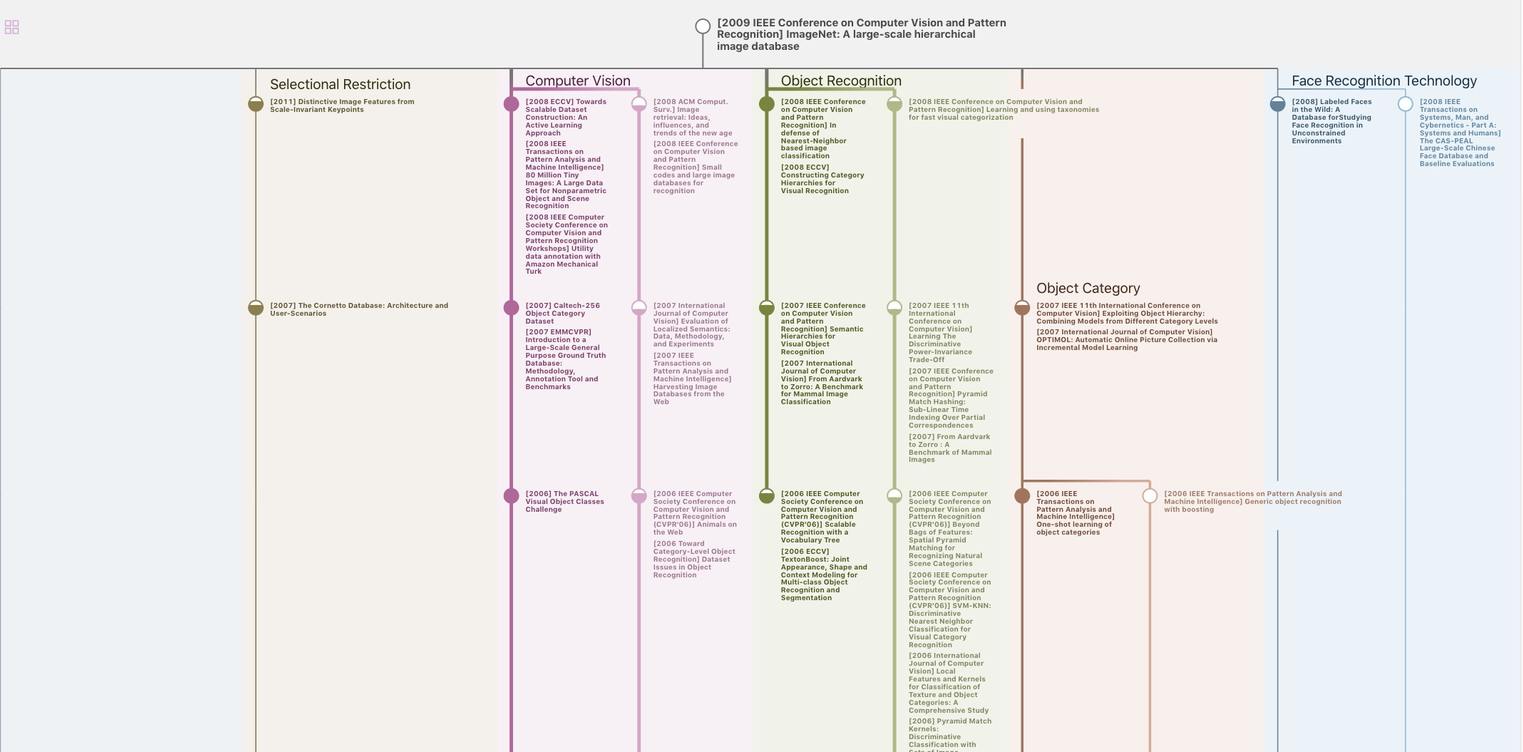
生成溯源树,研究论文发展脉络
Chat Paper
正在生成论文摘要