Continuous Viewpoint Planning in Conjunction with Dynamic Exploration for Active Object Recognition
ENTROPY(2021)
摘要
Active object recognition (AOR) aims at collecting additional information to improve recognition performance by purposefully adjusting the viewpoint of an agent. How to determine the next best viewpoint of the agent, i.e., viewpoint planning (VP), is a research focus. Most existing VP methods perform viewpoint exploration in the discrete viewpoint space, which have to sample viewpoint space and may bring in significant quantization error. To address this challenge, a continuous VP approach for AOR based on reinforcement learning is proposed. Specifically, we use two separate neural networks to model the VP policy as a parameterized Gaussian distribution and resort the proximal policy optimization framework to learn the policy. Furthermore, an adaptive entropy regularization based dynamic exploration scheme is presented to automatically adjust the viewpoint exploration ability in the learning process. To the end, experimental results on the public dataset GERMS well demonstrate the superiority of our proposed VP method.
更多查看译文
关键词
active object recognition, continuous viewpoint planning, adaptive entropy regularization, dynamic exploration, proximal policy optimization
AI 理解论文
溯源树
样例
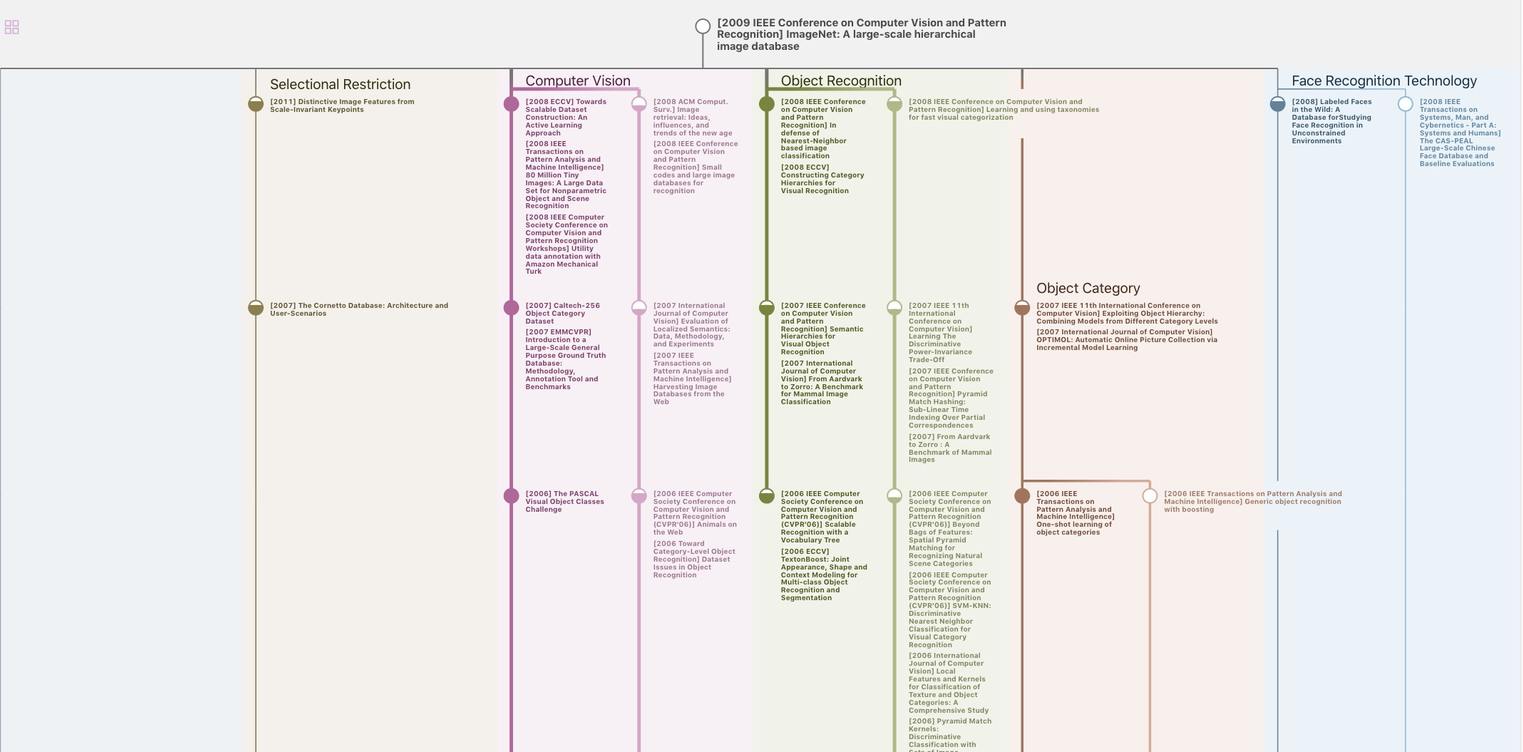
生成溯源树,研究论文发展脉络
Chat Paper
正在生成论文摘要