Approximability of all Boolean CSPs in the dynamic streaming setting
semanticscholar(2021)
摘要
A Boolean constraint satisfaction problem (CSP), Max-CSP(f), is a maximization problem specified by a constraint f : {−1, 1} → {0, 1}. An instance of the problem consists of m constraint applications on n Boolean variables, where each constraint application applies the constraint to k literals chosen from the n variables and their negations. The goal is to compute the maximum number of constraints that can be satisfied by a Boolean assignment to the n variables. In the (γ, β)-approximation version of the problem for parameters γ ≥ β ∈ [0, 1], the goal is to distinguish instances where at least γ fraction of the constraints can be satisfied from instances where at most β fraction of the constraints can be satisfied. In this work we consider the approximability of Max-CSP(f) in the (dynamic) streaming setting, where constraints are inserted (and may also be deleted in the dynamic setting) one at a time. We completely characterize the approximability of all Boolean CSPs in the dynamic streaming setting. Specifically, given f , γ and β we show that either (1) the (γ, β)-approximation version of Max-CSP(f) has a probabilistic dynamic streaming algorithm using O(log n) space, or (2) for every ε > 0 the (γ − ε, β + ε)-approximation version of Max-CSP(f) requires Ω( √ n) space for probabilistic dynamic streaming algorithms. We also extend previously known results in the insertion-only setting to a wide variety of cases, and in particular the case of k = 2 where we get a dichotomy and the case when the satisfying assignments of f support a distribution on {−1, 1} with uniform marginals. Our positive results show wider applicability of bias-based algorithms used previously by [GVV17] and [CGV20] by giving a systematic way to discover biases. Our negative results combine the Fourier analytic methods of [KKS15], which we extend to a wider class of CSPs, with a rich collection of reductions among communication complexity problems that lie at the heart of the negative results. This paper replaces the paper [CGSV21] by the authors. The previous version had errors and is now withdrawn. School of Engineering and Applied Sciences, Harvard University, Cambridge, Massachusetts, USA. Supported by NSF awards CCF 1565264 and CNS 1618026. Email: chiningchou@g.harvard.edu. Department of Computer Science, Georgetown University. Email: alexgolovnev@gmail.com. School of Engineering and Applied Sciences, Harvard University, Cambridge, Massachusetts, USA. Supported in part by a Simons Investigator Award and NSF Award CCF 1715187. Email: madhu@cs.harvard.edu. School of Engineering and Applied Sciences, Harvard University, Cambridge, Massachusetts, USA. Supported in part by a Simons Investigator Award and NSF Award CCF 1715187. Email: svelusamy@g.harvard.edu.
更多查看译文
AI 理解论文
溯源树
样例
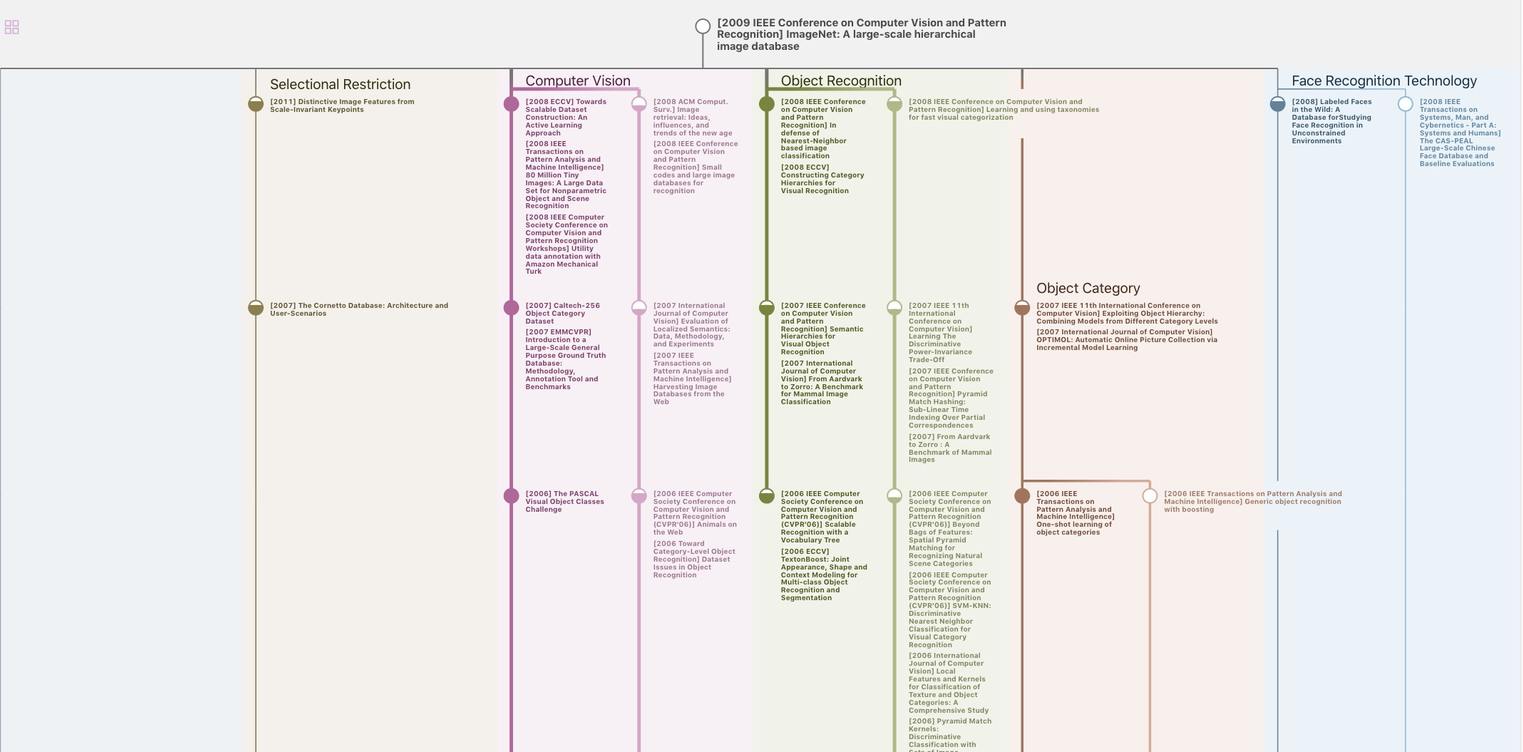
生成溯源树,研究论文发展脉络
Chat Paper
正在生成论文摘要