Self-Supervised Learning based Monaural Speech Enhancement with Multi-Task Pre-Training
arxiv(2021)
摘要
In self-supervised learning, it is challenging to reduce the gap between the enhancement performance on the estimated and target speech signals with existed pre-tasks. In this paper, we propose a multi-task pre-training method to improve the speech enhancement performance with self-supervised learning. Within the pre-training autoencoder (PAE), only a limited set of clean speech signals are required to learn their latent representations. Meanwhile, to solve the limitation of single pre-task, the proposed masking module exploits the dereverberated mask and estimated ratio mask to denoise the mixture as the second pre-task. Different from the PAE, where the target speech signals are estimated, the downstream task autoencoder (DAE) utilizes a large number of unlabeled and unseen reverberant mixtures to generate the estimated mixtures. The trained DAE is shared by the learned representations and masks. Experimental results on a benchmark dataset demonstrate that the proposed method outperforms the state-of-the-art approaches.
更多查看译文
关键词
monaural speech enhancement,learning,self-supervised,multi-task,pre-training
AI 理解论文
溯源树
样例
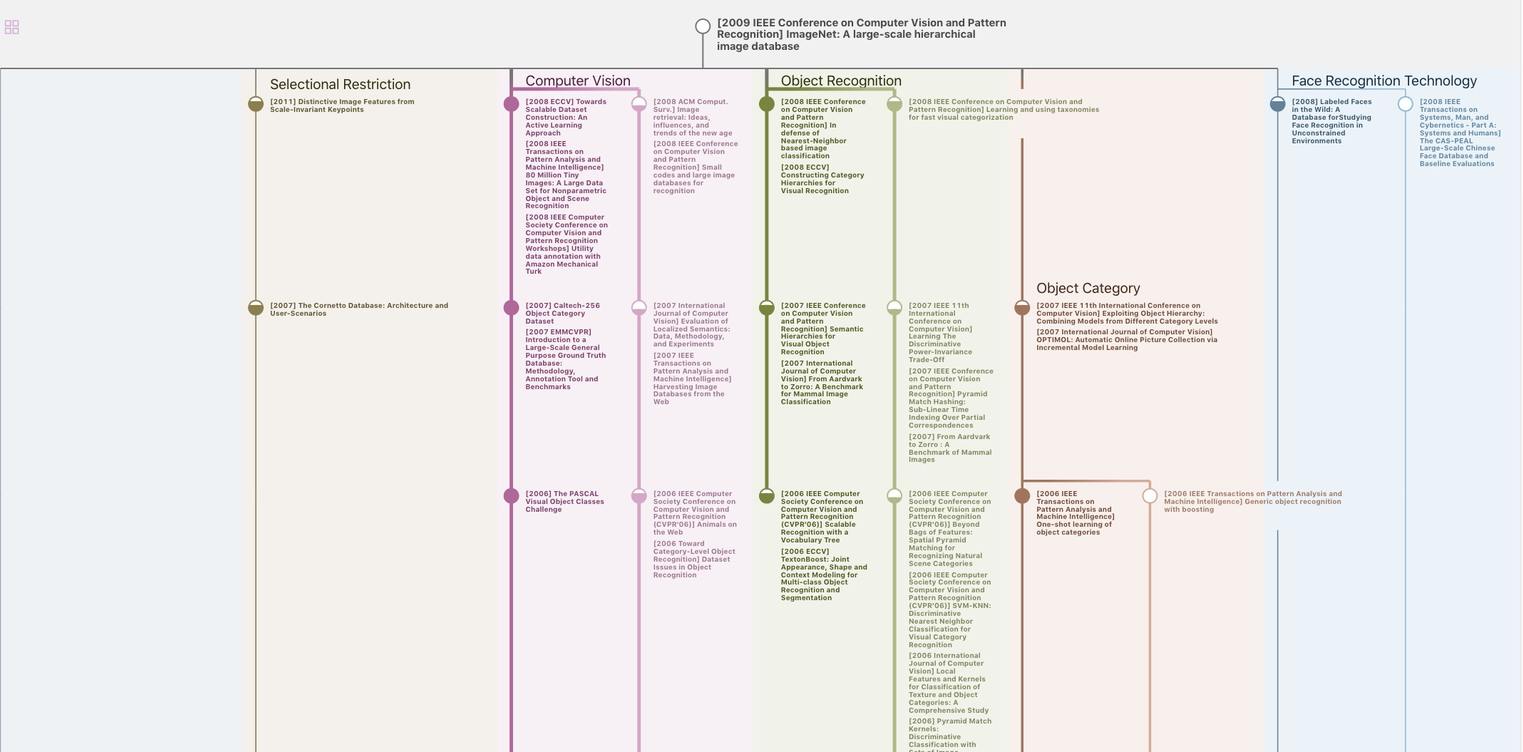
生成溯源树,研究论文发展脉络
Chat Paper
正在生成论文摘要