Predicting infections in the Covid-19 pandemic -- lessons learned
arxiv(2021)
摘要
Throughout the Covid-19 pandemic, a significant amount of effort had been put into developing techniques that predict the number of infections under various assumptions about the public policy and non-pharmaceutical interventions. While both the available data and the sophistication of the AI models and available computing power exceed what was available in previous years, the overall success of prediction approaches was very limited. In this paper, we start from prediction algorithms proposed for XPrize Pandemic Response Challenge and consider several directions that might allow their improvement. Then, we investigate their performance over medium-term predictions extending over several months. We find that augmenting the algorithms with additional information about the culture of the modeled region, incorporating traditional compartmental models and up-to-date deep learning architectures can improve the performance for short term predictions, the accuracy of medium-term predictions is still very low and a significant amount of future research is needed to make such models a reliable component of a public policy toolbox.
更多查看译文
关键词
infections
AI 理解论文
溯源树
样例
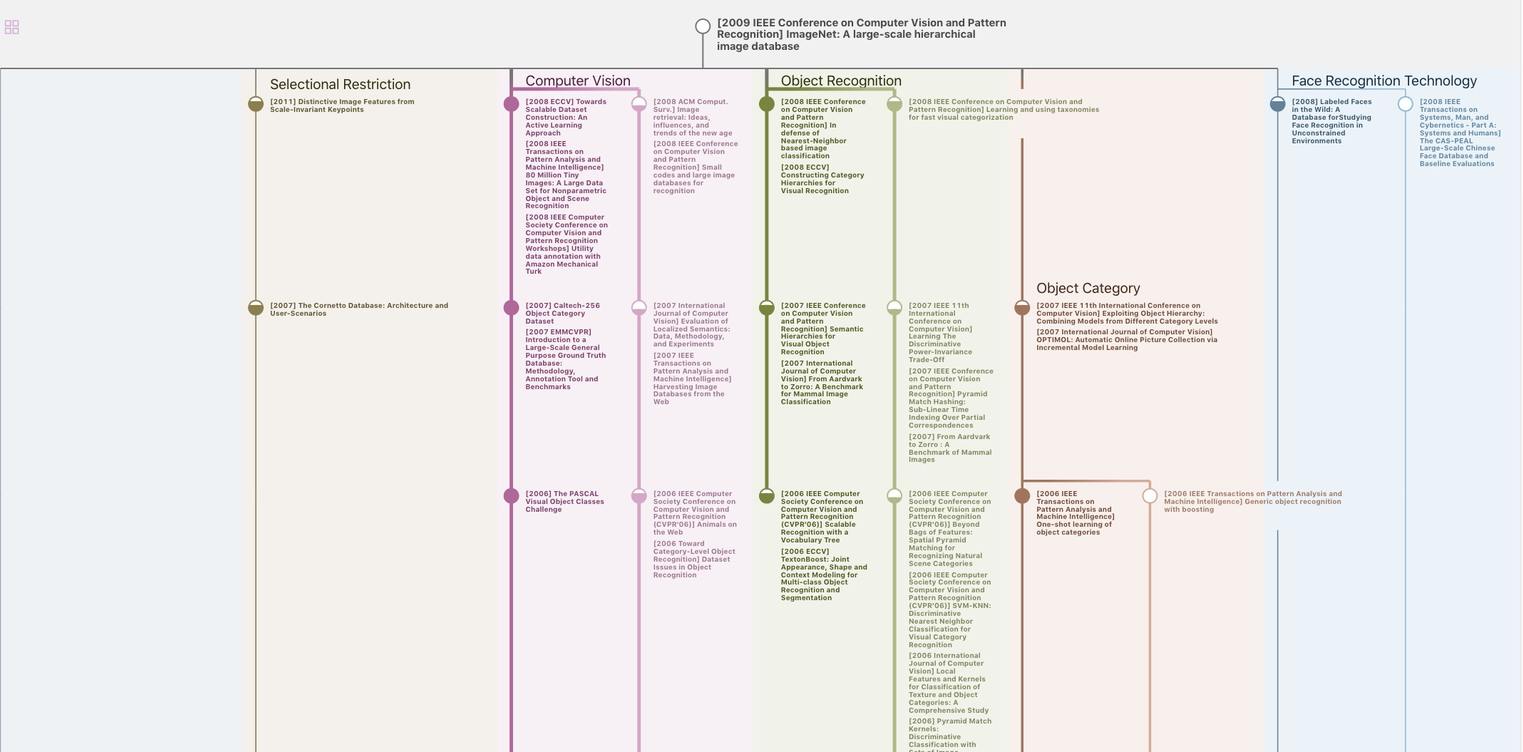
生成溯源树,研究论文发展脉络
Chat Paper
正在生成论文摘要