Regularity based spectral clustering and mapping the Fiedler-carpet
arxiv(2021)
摘要
Spectral clustering is discussed from many perspectives, by extending it to rectangular arrays and discrepancy minimization too. Near optimal clusters are obtained with singular value decomposition and with the weighted $k$-means algorithm. In case of rectangular arrays, this means enhancing the method of correspondence analysis with clustering, and in case of edge-weighted graphs, a normalized Laplacian based clustering. In the latter case it is proved that a spectral gap between the $(k-1)$th and $k$th smallest positive eigenvalues of the normalized Laplacian matrix gives rise to a sudden decrease of the inner cluster variances when the number of clusters of the vertex representatives is $2^{k-1}$, but only the first $k-1$ eigenvectors, constituting the so-called Fiedler-carpet, are used in the representation. Application to directed migration graphs is also discussed.
更多查看译文
关键词
multiway discrepancy, correspondence analysis, normalized Laplacian, multiway cuts, Fiedler-vector, weighted k-means algorithm
AI 理解论文
溯源树
样例
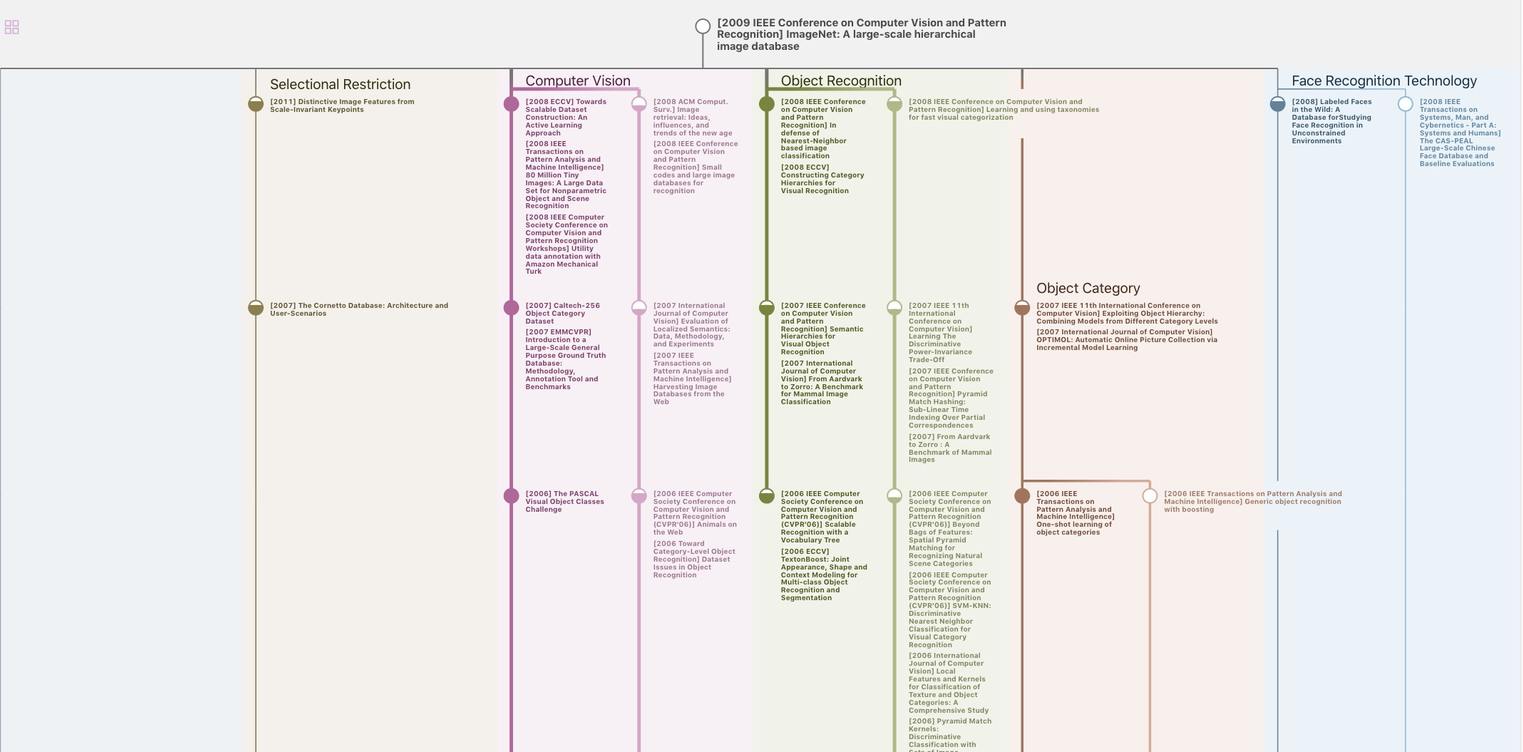
生成溯源树,研究论文发展脉络
Chat Paper
正在生成论文摘要