Turbo-Sim: a generalised generative model with a physical latent space
arxiv(2021)
摘要
We present Turbo-Sim, a generalised autoencoder framework derived from principles of information theory that can be used as a generative model. By maximising the mutual information between the input and the output of both the encoder and the decoder, we are able to rediscover the loss terms usually found in adversarial autoencoders and generative adversarial networks, as well as various more sophisticated related models. Our generalised framework makes these models mathematically interpretable and allows for a diversity of new ones by setting the weight of each loss term separately. The framework is also independent of the intrinsic architecture of the encoder and the decoder thus leaving a wide choice for the building blocks of the whole network. We apply Turbo-Sim to a collider physics generation problem: the transformation of the properties of several particles from a theory space, right after the collision, to an observation space, right after the detection in an experiment.
更多查看译文
关键词
generalised generative model,physical,turbo-sim
AI 理解论文
溯源树
样例
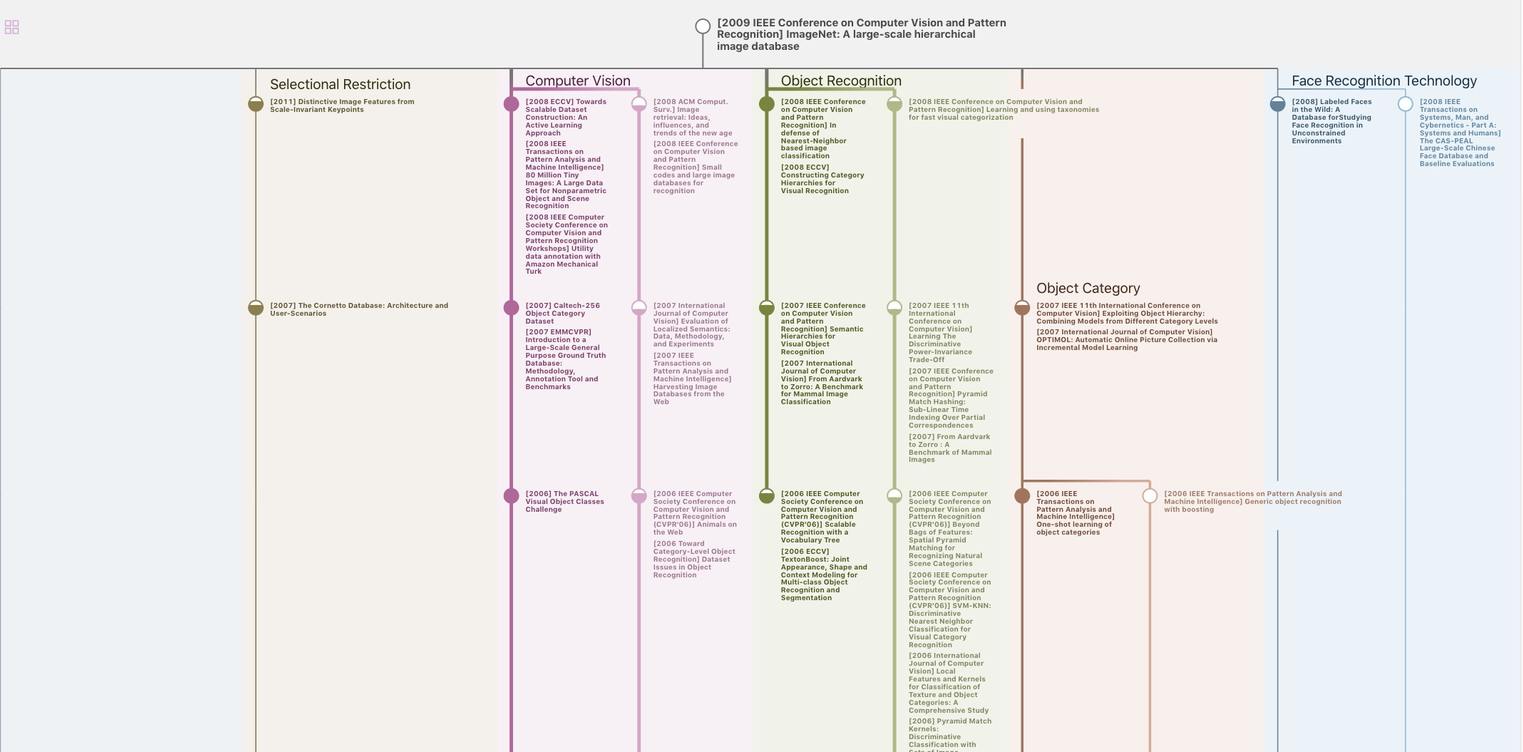
生成溯源树,研究论文发展脉络
Chat Paper
正在生成论文摘要